基于python实现ROC曲线绘制(二分类和多分类)
时间: 2023-09-04 20:12:02 浏览: 118
好的,我来回答你的问题。
首先,ROC曲线是用于评估二分类或多分类模型的性能指标之一。它是一条以假正率(False Positive Rate)为横坐标,真正率(True Positive Rate)为纵坐标的曲线。在二分类模型中,真正率等于真阳性率(True Positive Rate),假正率等于假阳性率(False Positive Rate)。
下面是基于Python实现二分类和多分类ROC曲线的步骤:
1. 导入必要的库:
```python
import numpy as np
import matplotlib.pyplot as plt
from sklearn.metrics import roc_curve, auc
```
2. 准备数据:
```python
# 二分类模型
y_true = np.array([0, 0, 1, 1, 0, 1])
y_score = np.array([0.3, 0.4, 0.5, 0.6, 0.2, 0.7])
# 多分类模型
y_true = np.array([0, 1, 2, 0, 1, 2])
y_score = np.array([[0.9, 0.1, 0.0], [0.2, 0.6, 0.2], [0.1, 0.2, 0.7], [0.8, 0.1, 0.1], [0.3, 0.4, 0.3], [0.2, 0.3, 0.5]])
```
其中,y_true为真实标签,y_score为预测标签的概率值。
3. 计算ROC曲线:
```python
# 二分类模型
fpr, tpr, thresholds = roc_curve(y_true, y_score)
roc_auc = auc(fpr, tpr)
# 多分类模型
n_classes = y_score.shape[1]
fpr = dict()
tpr = dict()
roc_auc = dict()
for i in range(n_classes):
fpr[i], tpr[i], _ = roc_curve(y_true, y_score[:, i], pos_label=i)
roc_auc[i] = auc(fpr[i], tpr[i])
```
4. 绘制ROC曲线:
```python
# 二分类模型
plt.figure()
plt.plot(fpr, tpr, color='darkorange', lw=2, label='ROC curve (area = %0.2f)' % roc_auc)
plt.plot([0, 1], [0, 1], color='navy', lw=2, linestyle='--')
plt.xlim([0.0, 1.0])
plt.ylim([0.0, 1.05])
plt.xlabel('False Positive Rate')
plt.ylabel('True Positive Rate')
plt.title('Receiver operating characteristic')
plt.legend(loc="lower right")
plt.show()
# 多分类模型
plt.figure()
colors = ['blue', 'red', 'green']
for i, color in zip(range(n_classes), colors):
plt.plot(fpr[i], tpr[i], color=color, lw=2, label='ROC curve of class %d (area = %0.2f)' % (i, roc_auc[i]))
plt.plot([0, 1], [0, 1], color='navy', lw=2, linestyle='--')
plt.xlim([0.0, 1.0])
plt.ylim([0.0, 1.05])
plt.xlabel('False Positive Rate')
plt.ylabel('True Positive Rate')
plt.title('Receiver operating characteristic')
plt.legend(loc="lower right")
plt.show()
```
这样,就可以得到二分类和多分类模型的ROC曲线了。
阅读全文
相关推荐
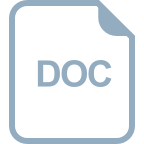
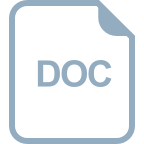
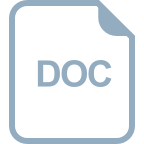
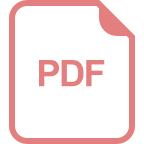
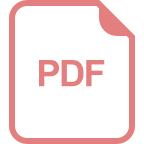
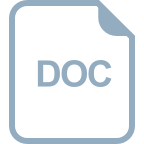











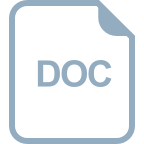
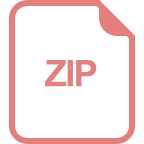
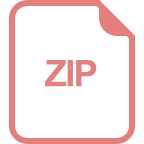