多分类问题绘制roc曲线,python
时间: 2023-07-28 07:27:19 浏览: 83
在Python中,可以使用scikit-learn库来绘制多分类问题的ROC曲线。以下是一个示例代码:
```python
from sklearn.metrics import roc_curve, auc
from sklearn.preprocessing import label_binarize
from itertools import cycle
import matplotlib.pyplot as plt
import numpy as np
# 生成随机数据
n_classes = 5
n_samples = 1000
y = np.random.randint(0, n_classes, size=n_samples)
y_score = np.random.rand(n_samples, n_classes)
# 将标签转换为二进制形式
y_bin = label_binarize(y, classes=np.arange(n_classes))
# 计算每个类别的ROC曲线和AUC值
fpr = dict()
tpr = dict()
roc_auc = dict()
for i in range(n_classes):
fpr[i], tpr[i], _ = roc_curve(y_bin[:, i], y_score[:, i])
roc_auc[i] = auc(fpr[i], tpr[i])
# 计算平均AUC值
average_auc = np.mean(list(roc_auc.values()))
# 绘制ROC曲线
plt.figure()
colors = cycle(['blue', 'red', 'green', 'purple', 'orange'])
for i, color in zip(range(n_classes), colors):
plt.plot(fpr[i], tpr[i], color=color, lw=2,
label='ROC curve of class {0} (AUC = {1:0.2f})'
''.format(i, roc_auc[i]))
plt.plot([0, 1], [0, 1], 'k--', lw=2)
plt.xlim([0.0, 1.0])
plt.ylim([0.0, 1.05])
plt.xlabel('False Positive Rate')
plt.ylabel('True Positive Rate')
plt.title('Multi-class ROC Curve')
plt.legend(loc="lower right")
plt.show()
```
在该示例代码中,我们先生成了一个随机的多分类数据集。然后将标签转换为二进制形式,并计算每个类别的ROC曲线和AUC值。最后,将所有类别的ROC曲线绘制在同一张图中,并计算平均AUC值。运行代码,就可以得到多分类问题的ROC曲线。
相关推荐
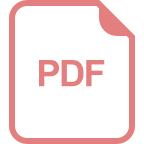
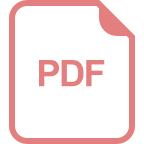
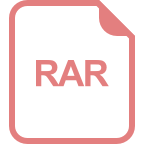
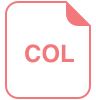
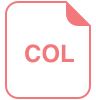
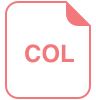
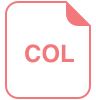










