immatrix1 = array([array(Image.open(imname1)).flatten() for imname1 in imlist1], 'f')
时间: 2024-06-01 17:11:03 浏览: 12
This code creates a 2D array called "immatrix1" where each row corresponds to an image and each column corresponds to a pixel value. It does this by iterating through a list of image names "imlist1", opening each image with the PIL (Python Imaging Library) "Image.open" function, flattening the image into a 1D array with the "flatten" method, and converting each element to a float datatype ('f'). The resulting array can be used for image processing and analysis.
相关问题
# Save PointMap to pm_path. def SavePlyFile(self,pm_path, pm, image): width = pm.GetSize().width height = pm.GetSize().height pm_num = width * height pm_ptr = np.array(pm, copy=False).reshape((-1, 3)) image_ptr = np.array(image, copy=False) if image.GetType() == RVC.ImageTypeEnum.Mono8: tmp = image_ptr.copy().flatten() image_ptr = np.zeros((height * width, 3)) image_ptr[:, 0] = tmp image_ptr[:, 1] = tmp image_ptr[:, 2] = tmp else: image_ptr = image_ptr.reshape(-1, 3) if image.GetType() == RVC.ImageTypeEnum.BGR8: image_ptr[:, [0, 2]] = image_ptr[:, [2, 0]] data = np.concatenate((pm_ptr, image_ptr), axis=1) np.savetxt(pm_path, data, fmt = '%f %f %f %d %d %d') with open(pm_path, 'r+') as f: old = f.read() f.seek(0) f.write("{}".format("ply")) f.write("\n{}".format("format ascii 1.0")) f.write("\n{}".format("comment Created by Rvbust, Inc")) f.write("\nelement vertex {}".format(pm_num)) f.write("\n{}".format("property float x")) f.write("\n{}".format("property float y")) f.write("\n{}".format("property float z")) f.write("\n{}".format("property uchar red")) f.write("\n{}".format("property uchar green")) f.write("\n{}".format("property uchar blue")) f.write("\n{}\n".format("end_header")) f.write(old) f.close() return
这段代码是用来将一个 PointMap 和一张图片保存为一个 .ply 文件的函数。具体来说,它接受三个参数:pm_path 表示保存的路径,pm 表示 PointMap 对象,image 表示图片对象。
首先,它通过 pm.GetSize() 获取 PointMap 的宽高信息,计算出 PointMap 中点的数量。然后,它将 PointMap 转换为一个二维 numpy 数组 pm_ptr,将图片转换为一个三维 numpy 数组 image_ptr。
接下来,它根据图片的类型进行判断。如果是 Mono8 类型,表示图片是单通道灰度图像,那么它将其转换为一个三通道数组,其中三个通道的值都相等。如果是其他类型,那么就将其转换为一个二维的三通道数组,并将 BGR 通道顺序转换为 RGB。
最后,它将 pm_ptr 和 image_ptr 按列连接成一个大的 numpy 数组 data,并将其写入到 pm_path 指定的文件中。在写入之前,它先写入了一个 .ply 文件的头部信息,包括文件格式、版本、注释、点的数量和属性等。最后,它将原文件中的内容追加到新写入的内容之后,完成文件的保存。
ValueError: cannot reshape array of size 1 into shape (417,556)
这个错误提示表明,您尝试将大小为1的数组重新形状为(417,556),但这是不可能的。出现这种情况的原因可能是在图像压缩或解压缩过程中出现了错误,导致数据大小不正确。
为了解决这个问题,您可以检查图像压缩或解压缩的过程,并确保数据的大小正确。您可以使用以下代码来调试并查看压缩后的数据的大小:
```python
import numpy as np
from PIL import Image
import lzss
def compress_gray_image(image_path, compression_level):
# 读取输入图像并转换为灰度图像
img = Image.open(image_path).convert('L')
# 转换为一维的numpy数组
data = np.array(img).flatten()
# 使用lzss算法进行压缩
compressed_data = lzss.compress(data, compression_level)
# 输出压缩后的数据大小
print('Compressed data size:', len(compressed_data))
# 将压缩后的数据写入到输出文件中
with open('compressed.bin', 'wb') as f:
f.write(compressed_data)
def decompress_gray_image(compressed_image_path):
# 读取压缩后的数据
with open(compressed_image_path, 'rb') as f:
compressed_data = f.read()
# 输出压缩后的数据大小
print('Compressed data size:', len(compressed_data))
# 使用lzss算法进行解压缩
decompressed_data = lzss.decompress(compressed_data)
# 输出解压缩后的数据大小
print('Decompressed data size:', len(decompressed_data))
# 将解压缩后的一维数组转换为二维数组
h, w = 417, 556 # 假设图像大小为417x556
data = np.reshape(decompressed_data, (h, w))
# 将二维数组转换为图像并显示
img = Image.fromarray(data.astype('uint8'), mode='L')
img.show()
# 测试代码
compress_gray_image('lena.png', compression_level=2)
decompress_gray_image('compressed.bin')
```
在上述代码中,我们分别在压缩和解压缩函数中输出了压缩后和解压缩后的数据大小,以便于您检查数据是否正确。如果数据大小不正确,您可以检查图像大小、压缩级别等参数是否正确,并尝试调整它们。
相关推荐
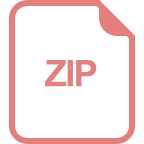
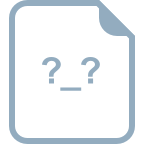
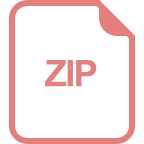












