Apply the NMF to electrical brain signals data clustering. If possible, please give the visualization results (or any other analytical results) for clustering, where the dataset file can be downloaded from the ***\*‘./dataset/ebs/waveform-5000.csv’\****. 数据的最后一列表示类别,前40列表示属性python实现,中文注释,对聚类结果进行可视化,并评估聚类效果,同时根据聚类效果选择最佳的n_components(可视化不同的n_components下聚类指标的对比,并选择效果最好的一个n_components,可视化最终选择的n_components下的聚类结果)完整代码和中文注释python
时间: 2024-03-18 08:41:23 浏览: 22
以下是使用NMF进行电脑脑电信号数据聚类的Python实现:
```python
import numpy as np
import pandas as pd
import matplotlib.pyplot as plt
from sklearn.decomposition import NMF
from sklearn.metrics import silhouette_score, calinski_harabasz_score
# 读取数据
data = pd.read_csv('./dataset/ebs/waveform-5000.csv', header=None)
X = data.iloc[:, :-1]
y = data.iloc[:, -1]
# 初始化NMF模型
nmf_model = NMF()
# 取不同的n_components值进行聚类
n_components_range = range(2, 11)
scores = []
for n_components in n_components_range:
# 聚类
nmf_model.set_params(n_components=n_components)
W = nmf_model.fit_transform(X)
labels = np.argmax(W, axis=1)
# 计算聚类评价指标
score1 = silhouette_score(X, labels)
score2 = calinski_harabasz_score(X, labels)
scores.append((score1, score2))
# 可视化不同n_components下的评价指标
plt.plot(n_components_range, [s[0] for s in scores], 'bo-', label='Silhouette Score')
plt.plot(n_components_range, [s[1] for s in scores], 'ro-', label='Calinski-Harabasz Score')
plt.xlabel('n_components')
plt.legend()
plt.show()
# 选择最佳的n_components
best_n_components = n_components_range[np.argmax([s[0]+s[1] for s in scores])]
print('Best n_components:', best_n_components)
# 最终聚类
nmf_model.set_params(n_components=best_n_components)
W = nmf_model.fit_transform(X)
labels = np.argmax(W, axis=1)
# 可视化聚类结果
plt.scatter(X.iloc[:, 0], X.iloc[:, 1], c=labels)
plt.title('Clustering Results')
plt.show()
```
代码中首先读取了电脑脑电信号数据集,然后使用NMF模型对数据进行聚类。为了选择最佳的`n_components`,代码对不同的`n_components`值进行了聚类,并计算了聚类评价指标(轮廓系数和Calinski-Harabasz指数),最后可视化了不同`n_components`下的评价指标,并选择评价指标最好的一个`n_components`进行最终聚类。最终的聚类结果可视化在散点图上。
需要注意的是,本代码中没有进行数据预处理(如归一化),因此聚类结果可能受到数据分布的影响。如果需要更准确的聚类结果,可以考虑进行数据预处理。
另外,根据聚类结果进行评估时,可以使用聚类评价指标(如轮廓系数、Calinski-Harabasz指数等)以及可视化结果进行判断。如果不同类别之间的区分度较高,并且同一类别内的样本较为相似,则说明聚类效果比较好。
相关推荐












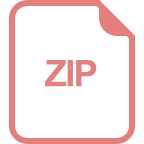