optim.RMSprop(net.parameters(), lr=lr, weight_decay=1e-8, momentum=0.9)
时间: 2024-05-29 10:14:41 浏览: 182
这是一个使用RMSprop优化器训练神经网络的代码。其中,net是你构建的神经网络模型,lr是学习率,weight_decay是权重衰减,momentum是动量。RMSprop是一种适应性学习率优化算法,可以自适应地调整每个参数的学习率,在训练过程中能够更快地收敛到最优解。同时,weight_decay可以有效地控制模型的复杂度,防止过拟合,而momentum则可以加速模型的收敛速度。
相关问题
import torch import torch.nn as nn import torch.nn.functional as F from torch.autograd import Variable class Bottleneck(nn.Module): def init(self, last_planes, in_planes, out_planes, dense_depth, stride, first_layer): super(Bottleneck, self).init() self.out_planes = out_planes self.dense_depth = dense_depth self.conv1 = nn.Conv2d(last_planes, in_planes, kernel_size=1, bias=False) self.bn1 = nn.BatchNorm2d(in_planes) self.conv2 = nn.Conv2d(in_planes, in_planes, kernel_size=3, stride=stride, padding=1, groups=32, bias=False) self.bn2 = nn.BatchNorm2d(in_planes) self.conv3 = nn.Conv2d(in_planes, out_planes+dense_depth, kernel_size=1, bias=False) self.bn3 = nn.BatchNorm2d(out_planes+dense_depth) self.shortcut = nn.Sequential() if first_layer: self.shortcut = nn.Sequential( nn.Conv2d(last_planes, out_planes+dense_depth, kernel_size=1, stride=stride, bias=False), nn.BatchNorm2d(out_planes+dense_depth) ) def forward(self, x): out = F.relu(self.bn1(self.conv1(x))) out = F.relu(self.bn2(self.conv2(out))) out = self.bn3(self.conv3(out)) x = self.shortcut(x) d = self.out_planes out = torch.cat([x[:,:d,:,:]+out[:,:d,:,:], x[:,d:,:,:], out[:,d:,:,:]], 1) out = F.relu(out) return out class DPN(nn.Module): def init(self, cfg): super(DPN, self).init() in_planes, out_planes = cfg['in_planes'], cfg['out_planes'] num_blocks, dense_depth = cfg['num_blocks'], cfg['dense_depth'] self.conv1 = nn.Conv2d(3, 64, kernel_size=3, stride=1, padding=1, bias=False) self.bn1 = nn.BatchNorm2d(64) self.last_planes = 64 self.layer1 = self._make_layer(in_planes[0], out_planes[0], num_blocks[0], dense_depth[0], stride=1) self.layer2 = self._make_layer(in_planes[1], out_planes[1], num_blocks[1], dense_depth[1], stride=2) self.layer3 = self._make_layer(in_planes[2], out_planes[2], num_blocks[2], dense_depth[2], stride=2) self.layer4 = self._make_layer(in_planes[3], out_planes[3], num_blocks[3], dense_depth[3], stride=2) self.linear = nn.Linear(out_planes[3]+(num_blocks[3]+1)dense_depth[3], 10) def _make_layer(self, in_planes, out_planes, num_blocks, dense_depth, stride): strides = [stride] + 1 layers = [] for i,stride in (strides): layers.append(Bottleneck(self.last_planes, in_planes, out_planes, dense_depth, stride, i==0)) self.last_planes = out_planes + (i+2) * dense_depth return nn.Sequential(*layers) def forward(self, x): out = F.relu(self.bn1(self.conv1(x))) out = self.layer1(out) out = self.layer2(out) out = self.layer3(out) out = self.layer4(out) out = F.avg_pool2d(out, 4) out = out.view(out.size(0), -1) out = self.linear(out) return out def DPN92(): cfg = { 'in_planes': (96,192,384,768), 'out_planes': (256,512,1024,2048), 'num_blocks': (3,4,20,3), 'dense_depth': (16,32,24,128) } return DPN(cfg)基于这个程序利用pytorch框架修改成图像检测与分类输出坐标、大小和种类
这个程序是一个深度神经网络模型DPN,用于图像分类任务。要将其修改为图像检测和分类模型,需要进行以下步骤:
1. 修改最后一层的输出。原来最后一层是线性层,输出分类结果。现在需要输出物体的坐标、大小和种类,可以将最后一层修改为三个分别输出这三个信息的线性层,例如:
```python
self.classifier = nn.Sequential(
nn.Linear(out_planes[3] * (num_blocks[3] + 1) * dense_depth[3], 256),
nn.Linear(256, num_classes + 4) # 输出种类+4个坐标
)
```
2. 修改前向传播函数。原来的前向传播函数将最后一层的输出经过线性层,得到分类结果。现在需要将最后一层的输出分别经过三个线性层,得到坐标、大小和种类信息,例如:
```python
out = F.avg_pool2d(out, 4)
out = out.view(out.size(0), -1)
out = self.classifier(out)
```
3. 修改训练过程。原来的训练过程只需要计算交叉熵损失,现在需要计算分类损失和回归损失。分类损失可以使用交叉熵损失,回归损失可以使用均方误差损失,例如:
```python
criterion_cls = nn.CrossEntropyLoss()
criterion_reg = nn.MSELoss()
optimizer = torch.optim.SGD(model.parameters(), lr=0.1, momentum=0.9, weight_decay=1e-4)
for epoch in range(num_epochs):
for i, (images, labels) in enumerate(train_loader):
images = images.to(device)
labels = labels.to(device)
optimizer.zero_grad()
outputs = model(images)
cls_loss = criterion_cls(outputs[:, :num_classes], labels[:, 0])
reg_loss = criterion_reg(outputs[:, num_classes:], labels[:, 1:])
loss = cls_loss + reg_loss
loss.backward()
optimizer.step()
if (i+1) % 100 == 0:
print ('Epoch [{}/{}], Step [{}/{}], Loss: {:.4f}'.format(epoch+1, num_epochs, i+1, total_step, loss.item()))
```
其中,`labels`是一个大小为`(batch_size, 5)`的张量,第一列是物体的种类,后四列是物体的坐标和大小。`num_classes`是物体的种类数目,`criterion_cls`是分类损失函数,`criterion_reg`是回归损失函数。
import torch.optim
`torch.optim`是PyTorch中用于优化算法的模块。它提供了各种优化器,如随机梯度下降(SGD)、Adam、Adagrad等,用于更新神经网络的参数以最小化损失函数。通过使用`torch.optim`模块,我们可以方便地在训练过程中调整学习率、设置动量、设置权重衰减等。
以下是一个使用`torch.optim`模块的例子:
```python
import torch
import torch.nn as nn
import torch.optim as optim
# 定义模型
model = nn.Linear(10, 2)
# 定义优化器
optimizer = optim.SGD(model.parameters(), lr=0.01, momentum=0.9, weight_decay=1e-5)
# 在训练过程中使用优化器更新模型参数
for epoch in range(100):
# 前向传播和计算损失
loss = ...
# 梯度清零
optimizer.zero_grad()
# 反向传播
loss.backward()
# 更新模型参数
optimizer.step()
```
在上面的例子中,我们使用了`optim.SGD`优化器,它使用随机梯度下降算法来更新模型参数。`lr`参数表示学习率,`momentum`参数表示动量,`weight_decay`参数表示权重衰减。
阅读全文
相关推荐
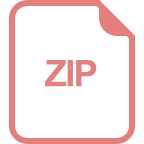
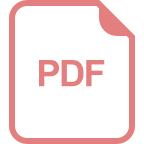
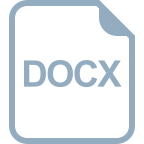
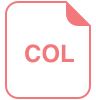










