python 实现图神经网络链路预测
时间: 2023-10-01 11:07:54 浏览: 70
图神经网络(Graph Neural Network,GNN)是一种用于处理图形数据的神经网络。链路预测是指在一个已知的图中,预测两个节点之间是否存在一条边。下面,我们将介绍如何使用 Python 实现图神经网络链路预测。
一、安装 PyTorch 和 DGL
首先,我们需要安装 PyTorch 和 DGL(Deep Graph Library)。可以通过以下命令来安装它们:
```
pip install torch
pip install dgl
```
二、准备数据
我们将使用一个来自 DGL 的数据集来演示链路预测。该数据集包含了一个论文引用网络,其中每个节点表示一篇论文,边表示引用关系。我们的任务是预测两篇论文之间是否存在引用关系。
我们可以使用以下代码来加载数据:
```
import dgl.data
dataset = dgl.data.CoraGraphDataset()
g = dataset[0]
```
在这个例子中,我们加载了 Cora 数据集,并获取了其中的第一个图。
三、构建模型
我们将使用 GNN 模型来预测链路。我们将使用 PyTorch Geometric 库来构建模型。以下是我们的代码:
```
import torch
import torch.nn.functional as F
from torch_geometric.nn import GCNConv
class Net(torch.nn.Module):
def __init__(self):
super(Net, self).__init__()
self.conv1 = GCNConv(dataset.num_features, 16)
self.conv2 = GCNConv(16, dataset.num_classes)
def forward(self, g, inputs):
h = self.conv1(g, inputs)
h = F.relu(h)
h = F.dropout(h, training=self.training)
h = self.conv2(g, h)
return h
```
我们定义了一个名为 Net 的类,它继承自 torch.nn.Module。在构造函数中,我们初始化了两个 GCNConv 层,分别用于输入层和输出层。在前向传递中,我们首先使用第一个层对输入进行卷积,然后使用 ReLU 激活函数和 Dropout 层进行激活和正则化,最后使用第二个层进行卷积并返回输出。
四、训练模型
在训练模型之前,我们需要定义一些超参数,如学习率、迭代次数等。以下是我们的代码:
```
import time
import numpy as np
import torch.optim as optim
from torch.utils.data import DataLoader
from torch.utils.data.sampler import SubsetRandomSampler
# 设置超参数
lr = 0.01
epochs = 200
batch_size = 32
train_size = 0.6
# 划分数据集
num_nodes = g.num_nodes()
indices = np.random.permutation(num_nodes)
split_idx = int(num_nodes * train_size)
train_loader = DataLoader(dataset, batch_size=batch_size,
sampler=SubsetRandomSampler(indices[:split_idx]))
test_loader = DataLoader(dataset, batch_size=batch_size,
sampler=SubsetRandomSampler(indices[split_idx:]))
device = torch.device('cuda' if torch.cuda.is_available() else 'cpu')
# 初始化模型和优化器
model = Net().to(device)
optimizer = optim.Adam(model.parameters(), lr=lr)
# 训练模型
model.train()
start_time = time.time()
for epoch in range(epochs):
train_loss = 0.0
for batch_idx, (inputs, targets, edge_index) in enumerate(train_loader):
inputs, targets, edge_index = inputs.to(device), targets.to(device), edge_index.to(device)
optimizer.zero_grad()
outputs = model(g, inputs)
loss = F.binary_cross_entropy_with_logits(outputs[edge_index[0]], targets.float())
loss.backward()
optimizer.step()
train_loss += loss.item() * inputs.size(0)
train_loss /= len(train_loader.dataset)
print('Epoch: {:04d}'.format(epoch+1),
'train_loss: {:.4f}'.format(train_loss),
'time: {:.4f}s'.format(time.time()-start_time))
```
在这个例子中,我们先将数据集划分为训练集和测试集。然后,我们使用 DataLoader 类将训练集和测试集转换为可迭代的数据集。接下来,我们初始化模型和优化器。在训练循环中,我们对每个批次进行前向传递和反向传递,并更新模型参数。我们还计算了训练集的平均损失,并打印了每个 epoch 的结果。
五、测试模型
最后,我们使用以下代码测试模型的性能:
```
def test(model, loader):
model.eval()
correct = 0
for batch_idx, (inputs, targets, edge_index) in enumerate(loader):
inputs, targets, edge_index = inputs.to(device), targets.to(device), edge_index.to(device)
with torch.no_grad():
outputs = model(g, inputs)
pred = (outputs[edge_index[0]] > 0).float()
correct += (pred == targets.float()).sum().item()
accuracy = correct / len(loader.dataset)
return accuracy
train_acc = test(model, train_loader)
test_acc = test(model, test_loader)
print('Train accuracy: {:.4f}'.format(train_acc))
print('Test accuracy: {:.4f}'.format(test_acc))
```
在这个例子中,我们使用 test() 函数对模型进行测试。在测试循环中,我们对每个批次进行前向传递,并计算预测准确率。最后,我们打印了训练集和测试集的准确率。
六、总结
在本文中,我们介绍了如何使用 Python 实现图神经网络链路预测。我们使用了 PyTorch 和 DGL 库来构建和训练 GNN 模型,并使用 PyTorch Geometric 库进行模型构建。通过本文的学习,您应该能够了解如何使用 Python 实现图神经网络链路预测,并且能够应用这些知识到实际的项目中。
相关推荐
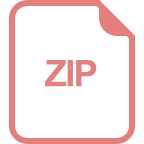













