auc的置信区间python
时间: 2023-08-07 14:01:17 浏览: 176
在Python中,我们可以使用Scikit-learn库来计算AUC(Area Under the Curve)以及其置信区间。以下是一个简单的示例:
```python
from sklearn import metrics
import numpy as np
# 生成随机的真实标签和预测概率
y_true = np.random.randint(0, 2, 100) # 真实标签(0或1)
y_pred_proba = np.random.rand(100) # 预测概率(0到1之间)
# 计算AUC
auc = metrics.roc_auc_score(y_true, y_pred_proba)
# 使用bootstrap方法获取AUC的置信区间
n_bootstrap = 1000 # bootstrap迭代次数
auc_scores = []
for _ in range(n_bootstrap):
indices = np.random.choice(len(y_true), len(y_true), replace=True)
sample_true = y_true[indices]
sample_pred_proba = y_pred_proba[indices]
auc_score = metrics.roc_auc_score(sample_true, sample_pred_proba)
auc_scores.append(auc_score)
conf_interval = np.percentile(auc_scores, [2.5, 97.5]) # 置信区间的下限和上限
print("AUC:", auc)
print("Confidence Interval:", conf_interval)
```
上述示例中,我们首先生成了100个随机的真实标签和预测概率。然后,使用`roc_auc_score`函数计算了AUC的值。接下来,通过bootstrap方法进行1000次迭代,随机选择数据样本并使用`roc_auc_score`计算每个样本的AUC值,并将这些值保存在`auc_scores`列表中。最后,使用`np.percentile`函数计算auc_scores的置信区间的下限和上限。
请注意,bootstrap方法是一种通过重新采样建立置信区间的技术。我们通过生成的独立样本来估计AUC的分布,并使用这些样本计算置信区间。这样,我们可以对AUC的估计结果进行统计显著性的推断。
阅读全文
相关推荐
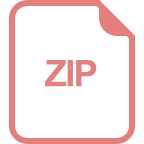
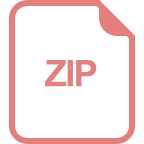
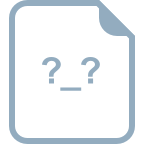











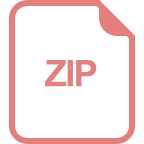
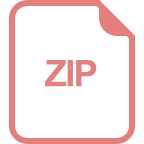
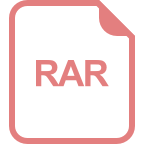
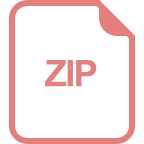
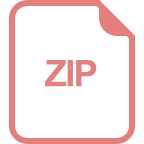