bp神经网络预测python包
时间: 2023-08-19 10:12:51 浏览: 214
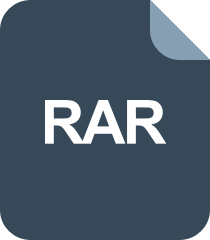
BP神经网络预测(python)
有几个常用的Python包可以用来构建和训练BP神经网络进行预测,其中最流行的包括:
1. Keras:Keras是一个高级神经网络API,可以在多个深度学习框架的后端上运行,包括TensorFlow、Microsoft Cognitive Toolkit(CNTK)和Theano。Keras简单易用,支持快速原型设计,并且提供了丰富的层和模型类型,适用于多种任务,包括BP神经网络的预测。
2. TensorFlow:TensorFlow是一个开源的深度学习框架,提供了丰富的工具和资源来构建和训练神经网络模型。通过TensorFlow的高级API,如tf.keras,可以方便地构建BP神经网络模型,并使用其强大的计算能力进行预测。
3. PyTorch:PyTorch是另一个流行的深度学习框架,提供了动态图机制和易于使用的API。PyTorch可以用于构建和训练BP神经网络,并且具有良好的可扩展性和灵活性。
这些包都提供了丰富的功能和工具来进行神经网络的构建、训练和预测。你可以根据自己的喜好和需求选择适合的包进行使用。
阅读全文
相关推荐
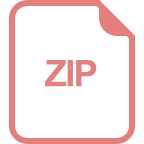
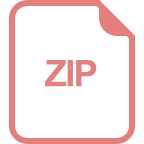
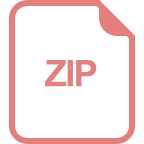











