pytorch中实现multiply操作的代码
时间: 2024-09-12 12:02:01 浏览: 39
在PyTorch中,`multiply`操作通常用于元素-wise乘法(也称为点积或逐元素乘法)。如果你想要实现矩阵乘法(通常是线性层中的权重与输入的乘法),你应该使用`torch.matmul()`函数,因为`multiply`更适用于简单的元素级乘法。
对于元素级别的乘法,你可以使用`torch.mul()`或者直接使用`*`运算符。例如,如果你想对张量`A`和`B`做逐元素乘法,可以这样写:
```python
import torch
# 假设我们有两个张量 A 和 B
A = torch.tensor([[1., 2.], [3., 4.]])
B = torch.tensor([[5., 6.], [7., 8.]])
# 使用mul()
elementwise_multiply_A_B = torch.mul(A, B)
# 或者使用 *
elementwise_multiply_A_B = A * B
elementwise_multiply_A_B
```
如果你指的是图注意力机制(Graph Attention Networks, GAT)中的注意力得分计算,比如`eij`部分,源码中的实现可能会涉及到张量的点积或者其他自定义操作。具体到PyGAT库,`_prepare_attentional_mechanism_input()`函数可能会涉及矩阵乘法(`torch.matmul()`),但实际细节取决于库的实现细节[^1]。
相关问题
pytorch代码实现在模型中使用LDAMLoss损失函数,数据集是RAF-DB,使用了mixup数据增强
好的,以下是使用LDAMLoss和mixup数据增强的RAF-DB数据集的PyTorch代码实现:
首先,我们需要导入必要的库:
```python
import torch
import torch.nn as nn
import torch.optim as optim
import torchvision.transforms as transforms
from torch.utils.data import DataLoader, Dataset
from torch.autograd import Variable
import numpy as np
import random
from PIL import Image
```
接下来,我们需要定义LDAM(Large Margin Cosine Loss)损失函数:
```python
class LDAMLoss(nn.Module):
def __init__(self, cls_num_list, max_m=0.5, weight=None, s=30):
super(LDAMLoss, self).__init__()
m_list = np.divide(1, np.sqrt(np.sqrt(cls_num_list)))
m_list = np.multiply(max_m, m_list)
m_list = torch.FloatTensor(m_list).cuda()
self.m_list = m_list
assert s > 0
self.s = s
if weight is not None:
self.weight = torch.FloatTensor(weight).cuda()
else:
self.weight = weight
def forward(self, x, target):
cosine = x
sine = torch.sqrt(1.0 - torch.pow(cosine, 2))
phi = cosine * self.m_list.unsqueeze(1) - sine * self.m_list.unsqueeze(1)
phi = phi.float()
target = target.long().view(-1, 1)
index = torch.zeros_like(phi)
index.scatter_(1, target, 1)
if self.weight is not None:
weight = self.weight.unsqueeze(0)
index = torch.matmul(index, weight.t())
index = index.clamp(min=1e-12, max=1 - 1e-12)
index = index.log()
loss = -index * torch.pow(torch.abs(phi), self.s)
loss = loss.sum(dim=1).mean()
else:
index = index.cuda()
loss = -torch.log(torch.abs(torch.gather(phi, 1, target)) + 1e-8)
loss = loss.squeeze(1)
loss = loss.mean()
return loss
```
接下来,我们需要定义mixup数据增强:
```python
def mixup_data(x, y, alpha=1.0):
if alpha > 0:
lam = np.random.beta(alpha, alpha)
else:
lam = 1
batch_size = x.size()[0]
index = torch.randperm(batch_size).cuda()
mixed_x = lam * x + (1 - lam) * x[index, :]
y_a, y_b = y, y[index]
return mixed_x, y_a, y_b, lam
```
然后,我们需要定义RAF-DB数据集的类:
```python
class RAFDataset(Dataset):
def __init__(self, data_path, transform=None):
self.data_path = data_path
self.transform = transform
self.data = []
self.labels = []
with open(self.data_path, 'r') as f:
for line in f:
line = line.strip()
img_path, label = line.split(' ')
self.data.append(img_path)
self.labels.append(int(label))
def __len__(self):
return len(self.data)
def __getitem__(self, index):
img_path = self.data[index]
label = self.labels[index]
img = Image.open(img_path).convert('RGB')
if self.transform is not None:
img = self.transform(img)
return img, label
```
接下来,我们需要定义模型:
```python
class MyModel(nn.Module):
def __init__(self):
super(MyModel, self).__init__()
self.conv1 = nn.Conv2d(3, 64, kernel_size=3, stride=1, padding=1)
self.bn1 = nn.BatchNorm2d(64)
self.relu1 = nn.ReLU(inplace=True)
self.conv2 = nn.Conv2d(64, 128, kernel_size=3, stride=1, padding=1)
self.bn2 = nn.BatchNorm2d(128)
self.relu2 = nn.ReLU(inplace=True)
self.conv3 = nn.Conv2d(128, 256, kernel_size=3, stride=1, padding=1)
self.bn3 = nn.BatchNorm2d(256)
self.relu3 = nn.ReLU(inplace=True)
self.conv4 = nn.Conv2d(256, 512, kernel_size=3, stride=1, padding=1)
self.bn4 = nn.BatchNorm2d(512)
self.relu4 = nn.ReLU(inplace=True)
self.pool = nn.MaxPool2d(kernel_size=2, stride=2)
self.fc1 = nn.Linear(512 * 12 * 12, 1024)
self.drop1 = nn.Dropout(p=0.5)
self.relu5 = nn.ReLU(inplace=True)
self.fc2 = nn.Linear(1024, 7)
def forward(self, x):
x = self.conv1(x)
x = self.bn1(x)
x = self.relu1(x)
x = self.conv2(x)
x = self.bn2(x)
x = self.relu2(x)
x = self.conv3(x)
x = self.bn3(x)
x = self.relu3(x)
x = self.conv4(x)
x = self.bn4(x)
x = self.relu4(x)
x = self.pool(x)
x = x.view(-1, 512 * 12 * 12)
x = self.fc1(x)
x = self.drop1(x)
x = self.relu5(x)
x = self.fc2(x)
return x
```
最后,我们需要定义训练和测试函数:
```python
def train(model, train_loader, optimizer, criterion, alpha):
model.train()
train_loss = 0
train_correct = 0
train_total = 0
for i, (inputs, targets) in enumerate(train_loader):
inputs, targets_a, targets_b, lam = mixup_data(inputs, targets, alpha=alpha)
inputs, targets_a, targets_b = map(Variable, (inputs, targets_a, targets_b))
optimizer.zero_grad()
outputs = model(inputs)
loss = criterion(outputs, targets_a) * lam + criterion(outputs, targets_b) * (1 - lam)
loss.backward()
optimizer.step()
train_loss += loss.item()
_, predicted = torch.max(outputs.data, 1)
train_total += targets.size(0)
train_correct += (lam * predicted.eq(targets_a.data).cpu().sum().float()
+ (1 - lam) * predicted.eq(targets_b.data).cpu().sum().float())
train_acc = train_correct / train_total
train_loss = train_loss / len(train_loader)
return train_acc, train_loss
def test(model, test_loader, criterion):
model.eval()
test_loss = 0
test_correct = 0
test_total = 0
with torch.no_grad():
for inputs, targets in test_loader:
inputs, targets = Variable(inputs), Variable(targets)
outputs = model(inputs)
loss = criterion(outputs, targets)
test_loss += loss.item()
_, predicted = torch.max(outputs.data, 1)
test_total += targets.size(0)
test_correct += predicted.eq(targets.data).cpu().sum().float()
test_acc = test_correct / test_total
test_loss = test_loss / len(test_loader)
return test_acc, test_loss
```
最后,我们需要定义主函数:
```python
if __name__ == '__main__':
# 设置随机种子,确保实验的可重复性
torch.manual_seed(233)
np.random.seed(233)
random.seed(233)
# 定义训练参数
batch_size = 64
num_epochs = 100
lr = 0.1
alpha = 1.0
cls_num_list = [2000, 2000, 2000, 2000, 2000, 2000, 2000]
train_data_path = 'train.txt'
test_data_path = 'test.txt'
# 定义数据增强和数据集
transform_train = transforms.Compose([
transforms.RandomCrop(44),
transforms.RandomHorizontalFlip(),
transforms.ToTensor(),
transforms.Normalize(mean=[0.5, 0.5, 0.5], std=[0.5, 0.5, 0.5])
])
transform_test = transforms.Compose([
transforms.CenterCrop(44),
transforms.ToTensor(),
transforms.Normalize(mean=[0.5, 0.5, 0.5], std=[0.5, 0.5, 0.5])
])
train_dataset = RAFDataset(train_data_path, transform=transform_train)
test_dataset = RAFDataset(test_data_path, transform=transform_test)
# 定义数据加载器
train_loader = DataLoader(train_dataset, batch_size=batch_size, shuffle=True, num_workers=4)
test_loader = DataLoader(test_dataset, batch_size=batch_size, shuffle=False, num_workers=4)
# 定义模型和优化器
model = MyModel().cuda()
optimizer = optim.SGD(model.parameters(), lr=lr, momentum=0.9, weight_decay=5e-4)
criterion = LDAMLoss(cls_num_list)
# 训练和测试
for epoch in range(num_epochs):
train_acc, train_loss = train(model, train_loader, optimizer, criterion, alpha)
test_acc, test_loss = test(model, test_loader, criterion)
print('Epoch [{}/{}], Train Loss: {:.4f}, Train Acc: {:.4f}, Test Loss: {:.4f}, Test Acc: {:.4f}'
.format(epoch + 1, num_epochs, train_loss, train_acc, test_loss, test_acc))
if (epoch + 1) % 10 == 0:
lr /= 10
optimizer = optim.SGD(model.parameters(), lr=lr, momentum=0.9, weight_decay=5e-4)
```
pytorch计算网络模型flops的代码
可以使用下面的代码计算PyTorch模型的FLOPs(浮点操作次数):
```python
import torch
from torch.autograd import Variable
def print_model_parm_flops(model, input_size, custom_layers):
multiply_adds = 1
params = 0
flops = 0
input = Variable(torch.rand(1, *input_size))
def register_hook(module):
def hook(module, input, output):
class_name = str(module.__class__).split(".")[-1].split("'")[0]
if class_name == 'Conv2d':
out_h, out_w = output.size()[2:]
kernel_h, kernel_w = module.kernel_size
in_channels = module.in_channels
out_channels = module.out_channels
if isinstance(module.padding, int):
pad_h = pad_w = module.padding
else:
pad_h, pad_w = module.padding
if isinstance(module.stride, int):
stride_h = stride_w = module.stride
else:
stride_h, stride_w = module.stride
params += out_channels * (in_channels // module.groups) * kernel_h * kernel_w
flops += out_channels * (in_channels // module.groups) * kernel_h * kernel_w * out_h * out_w / (stride_h * stride_w)
elif class_name == 'Linear':
weight_flops = module.weight.nelement() * input[0].nelement() // module.weight.size(1)
bias_flops = module.bias.nelement()
flops = weight_flops + bias_flops
params = weight_flops + bias_flops
elif class_name in custom_layers:
custom_flops, custom_params = custom_layers[class_name](module, input, output)
flops += custom_flops
params += custom_params
else:
print(f"Warning: module {class_name} not implemented")
if not isinstance(module, torch.nn.Sequential) and \
not isinstance(module, torch.nn.ModuleList) and \
not (module == model):
hooks.append(module.register_forward_hook(hook))
# loop through the model architecture and register hooks for each layer
hooks = []
model.apply(register_hook)
# run the input through the model
model(input)
# remove the hooks
for hook in hooks:
hook.remove()
print(f"Number of parameters: {params}")
print(f"Number of FLOPs: {flops}")
return flops, params
```
调用这个函数需要传入模型、输入大小和一个自定义图层字典,其中字典的键是自定义层的名称,值是一个函数,该函数接受模块,输入和输出,返回FLOPs和参数数量。例如,如果您的模型包含一个名为MyLayer的自定义层,则可以将以下内容添加到字典中:
```python
def my_layer_impl(module, input, output):
# compute FLOPs and params for MyLayer
flops = ...
params = ...
return flops, params
custom_layers = {'MyLayer': my_layer_impl}
```
使用示例:
```python
import torchvision.models as models
model = models.resnet18()
input_size = (3, 224, 224)
custom_layers = {}
flops, params = print_model_parm_flops(model, input_size, custom_layers)
```
该函数将打印出模型的参数数量和FLOPs。
阅读全文
相关推荐
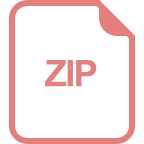
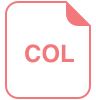
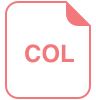
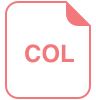
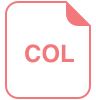
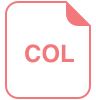









