用python写一个能够识别手写字母的程序
时间: 2024-10-25 22:12:50 浏览: 25
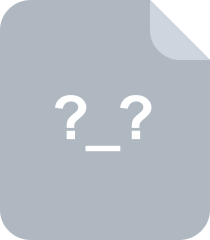
卷积神经网络实例(手写字母识别)--PyTorch

创建一个能识别手写字母的Python程序通常涉及到图像处理、机器学习和深度学习技术,特别是使用卷积神经网络(CNN)。这里是一个简单的概述:
1. **数据预处理**:首先需要大量的手写字母图片数据集,比如MNIST或EMNIST等。然后对图片进行灰度化、归一化等操作。
```python
from PIL import Image
def preprocess_image(img_path):
img = Image.open(img_path).convert('L') # 转为灰度
img = img.resize((28, 28)) # 缩放到固定大小
return img
```
2. **模型选择**:使用TensorFlow或Keras库构建一个CNN模型,如LeNet、VGG16或更复杂的架构。
```python
import tensorflow as tf
from tensorflow.keras.models import Sequential
from tensorflow.keras.layers import Conv2D, MaxPooling2D, Flatten, Dense
model = Sequential([
Conv2D(32, (3, 3), activation='relu', input_shape=(28, 28, 1)),
MaxPooling2D((2, 2)),
Conv2D(64, (3, 3), activation='relu'),
MaxPooling2D((2, 2)),
Flatten(),
Dense(128, activation='relu'),
Dense(26, activation='softmax') # 26因为包括了数字0-9和大写英文字母A-Z
])
```
3. **训练模型**:加载数据集,将图片转换为张量,并进行训练。
```python
(x_train, y_train), (x_test, y_test) = tf.keras.datasets.mnist.load_data()
# 对输入做预处理
x_train = x_train.reshape(-1, 28, 28, 1) / 255
x_test = x_test.reshape(-1, 28, 28, 1) / 255
model.compile(optimizer='adam', loss='sparse_categorical_crossentropy', metrics=['accuracy'])
model.fit(x_train, y_train, epochs=10, validation_data=(x_test, y_test))
```
4. **预测**:使用训练好的模型对新的手写字母图片进行分类。
```python
def predict_letter(image_path):
image = preprocess_image(image_path)
prediction = model.predict(np.array([image])) # 归一化并预测
predicted_label = np.argmax(prediction) # 获取最高概率类别
return chr(predicted_label + ord('A')) # 返回预测的大写英文字母
```
阅读全文
相关推荐
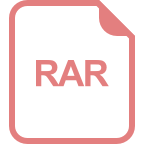



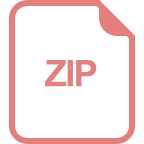
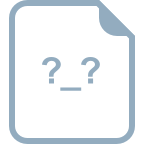









