遗传算法求tsp问题的试验目的
时间: 2024-05-13 07:12:27 浏览: 92
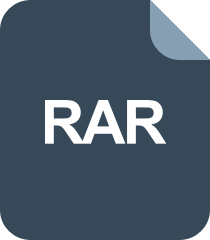
遗传算法解决TSP问题实验
遗传算法是一种基于生物进化思想的优化算法,能够应用于各种优化问题中。TSP问题是指旅行商问题,即如何在所有城市之间找到一条最短的路径,使得旅行商可以在每个城市中仅访问一次并最终回到起点。因此,将遗传算法应用于TSP问题中,就是希望通过模拟自然进化过程,求出一条最短的路径。
试验目的包括但不限于以下几点:
1. 研究遗传算法在TSP问题中的适应性和优化效果;
2. 探究遗传算法各个参数对算法性能的影响,如种群规模、交叉概率、变异概率等;
3. 比较遗传算法与其他求解TSP问题的算法,如蚁群算法、模拟退火算法等,寻找最优解;
4. 探究TSP问题本身的特性以及其对遗传算法的影响,如城市数量、城市分布情况等。
阅读全文
相关推荐
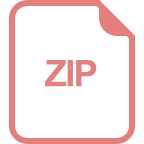
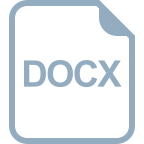
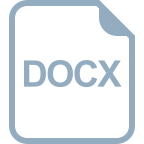
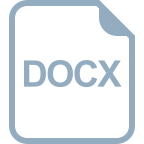
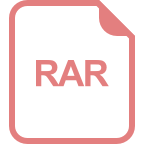
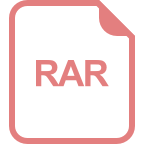
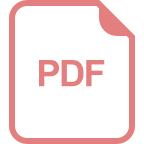
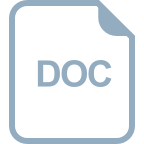
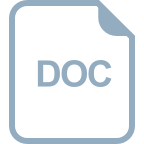
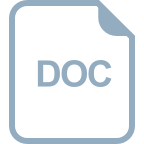
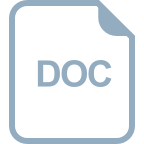
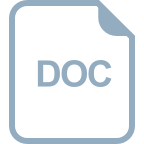
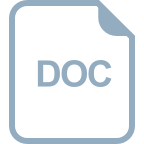
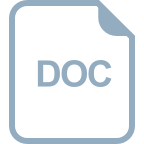
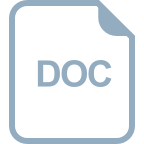