文献里出现了以下语句:(a) the complexity of the population level value function θ that is being estimated 请翻译这句话并用几个例子解释这里的population是指什么含义
时间: 2024-04-15 07:26:42 浏览: 121
翻译:(a) 正在估计的种群级值函数 θ 的复杂性。
解释:在这个语句中,"population" 指的是一个特定的群体、总体或者整体。它可以表示一个整个国家的人口,一个研究中的参与者群体,或者是一个特定的样本群体。
这里的 "population level value function" 指的是一种在整个群体中估计的值函数。该值函数描述了群体中每个个体的某种属性或特征的变化。通过对整个群体进行分析,我们可以获得关于群体整体行为或特征的信息。
下面是几个例子来解释这个概念:
1. 假设有一个研究人员想要估计某个城市的平均收入水平。这个 "population" 可以是整个城市的人口,而 "population level value function" 就是用来估计该城市人口平均收入水平的函数。
2. 在医学研究中,研究人员可能对某种疾病在某个地区的传播速度感兴趣。这里的 "population" 可能是该地区的居民总体,而 "population level value function" 可能是用来估计该地区每日新增感染病例数的函数。
3. 在市场调研中,研究人员可能对某种产品在整个国家的市场份额感兴趣。这里的 "population" 可能是整个国家的人口,而 "population level value function" 可能是用来估计该产品在不同地区的销售量的函数。
总之,"population level value function" 是用来描述在整个群体中估计某种属性或特征的函数,而 "population" 则是指被研究的整体群体。
相关问题
It looks like you are adding an Embedding layer to your model using Keras. This layer will be used to convert your input text data into a dense vector representation that can be processed by your model. Here's what each of the arguments you've provided to the Embedding layer mean: input_dim: This specifies the size of the vocabulary, or the number of unique words in your input data. It should be set to MAX_WORDS_NUM+1, which ensures that all words in your data are assigned a unique index. output_dim: This specifies the size of the embedding vector for each word. Typically, this is set to a value between 50 and 300, depending on the size of your dataset and complexity of the task. input_length: This specifies the length of each input sequence, or the number of words in each document. It should be set to MAX_SEQUENCE_LEN, which ensures that all input sequences have the same length and can be processed by your model. By adding the Embedding layer to your model, you are essentially creating a lookup table that maps each word in your vocabulary to a dense vector representation. This allows your model to learn meaningful relationships between words and make predictions based on the context of the input data.翻译
看起来你正在使用Keras向你的模型添加一个Embedding层。这个层将被用来将你的输入文本数据转换成一个密集的向量表示,可以被你的模型处理。下面是你提供给Embedding层的每个参数的含义:
- input_dim: 这个参数指定了词汇表的大小,或者说是输入数据中唯一单词的数量。它应该设置为MAX_WORDS_NUM+1,这样确保所有单词都被分配了一个唯一的索引。
- output_dim: 这个参数指定了每个单词的嵌入向量的大小。通常,这个值设置为50到300之间的一个值,取决于数据集的大小和任务的复杂性。
- input_length: 这个参数指定了每个输入序列的长度,或者说是每个文档中单词的数量。它应该设置为MAX_SEQUENCE_LEN,这样确保所有输入序列都具有相同的长度,可以被你的模型处理。
通过向你的模型添加Embedding层,你实际上创建了一个查找表,将词汇表中的每个单词映射到一个密集的向量表示。这样,你的模型可以学习单词之间的有意义的关系,并根据输入数据的上下文进行预测。
继上To be clear, the relevance of the kernel complexity function C on the left-hand side (CI(ζ)) is well-known from past work on kernel ridge regression; 请解释kernel complexity function并解释这句话
在这句话中,提到了核复杂度函数(kernel complexity function)以及它在过去的核岭回归工作中的重要性。
核复杂度函数是用来度量核方法中模型的复杂度的函数。在核岭回归中,我们使用核函数将输入数据映射到RKHS,并在RKHS中进行回归分析。为了控制模型的复杂度,我们引入了一个正则化项,通常被称为核复杂度。
核复杂度函数表示了正则化项的大小和模型复杂度之间的关系。它通常与核函数的性质和数据分布有关。通过调整核复杂度函数的值,可以平衡模型的拟合优度和模型的复杂度,避免过拟合。
在过去的核岭回归工作中,研究人员已经深入研究了核复杂度函数的性质和作用。这些研究揭示了核复杂度函数对模型的影响以及如何选择合适的核复杂度值以获得最佳的回归结果。
因此,文中提到的这句话意味着核复杂度函数在过去的核岭回归研究中已经被广泛认识和研究,并且对于理解和优化模型的性能具有重要的意义。
阅读全文
相关推荐


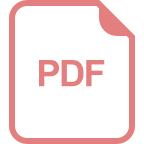













