We also assessed the computational efficiency of our subsampling method. The computations were carried out using R (R Core Team, 2021) on a desktop computer with 64GB memory. We restricted the calculations to access one CPU core and recorded the average CPU time from ten repetitions. Table 5 reports the results for Case I, where the subsample size is r = 1000. The computational speed of the OSP estimator is much faster than that of the full data estimator. Particularly, the computational burden of the full data method gets heavier as the increase of full data sample size. The computation time of the full data estimator increases in a polynomial order of n which agrees with the theoretical time complexity of O(n2). This full data time complexity is different from linear models (Wang et al., 2019) or logistic regression models (Wang et al., 2018), for which the time complexity is linear in sample size, i.e., O(n). In other words, subsampling is more desirable in Cox’s regression because it reduce the computational cost more significantly. The UNIF estimator is faster to compute than the OSP estimator, because it does not need the step of calculating the sampling probabilities, but it has a lower estimation efficiency as we have seen in previous results
时间: 2023-02-22 18:55:19 浏览: 123
我们还评估了我们的采样方法的计算效率。计算是使用R (R Core Team, 2021)在具有64GB内存的台式电脑上进行的。我们将计算限制为访问一个CPU核心,并记录了十次重复的平均CPU时间。表5报告了Case I的结果,其中子样本大小为r=1000。 OSP估计量的计算速度比全数据估计量快得多。特别是,随着全数据样本大小的增加,全数据方法的计算负担变得更重。全数据估计量的计算时间以n的多项式顺序增加,这符合O(n^2)的理论时间复杂度。这与线性模型(Wang et al., 2019)或逻辑回归模型(Wang et al., 2018)的全数据时间复杂度不同,其全数据时间复杂度是样本大小的线性,即O(n)。换句话说,在Cox回归中,抽样更可取,因为它可以更显着地降低计算成本。 UNIF估计量比OSP估计量计算速度快,因为它不需要计算抽样概率的步骤,但由于我们在以前的结果中看到的低估计效率,因此它的估计效率较低。
相关问题
Then, the median absolute deviations (MAD) was calculated for each remained feature21. Features with MAD equal to zero were discarded, as these features were considered as non-informative. After this step, 33881 features were left. Next, we further selected features with prognostic value. Here the prognostic performance is assessed using the concordance index (C-index), a generalization of the area under the receiver operating characteristic (ROC) curve (AUC)22. The C-index for each feature was calculated. Features with C-index ≥ 0.580 are considered as predictive factors. After prognostic performance analysis, 1581 features remained. Then, we further reduced the data dimension by removing highly correlated features. Here the correlation coefficient between each pair of features is calculated. For feature pair with correlated coefficient ≥0.90, the more prognostic feature is retained and the other feature is removed. Finally, the remained 150 image features are selected and regarded as robust, predictive and nonredundant. 解释
该段文字描述了一个数据特征选择的过程。首先,对于所有特征,计算其中位数绝对偏差(MAD),并移除MAD等于零的特征,因为这些特征被认为是非信息性的。经过此步骤,剩下33881个特征。然后,使用协调指数(C-index)对这些特征进行预测价值分析,C-index是接收者操作特征(ROC)曲线下面积(AUC)的推广。具有C-index≥0.580的特征被视为预测性因素。经过预测性能分析后,剩下1581个特征。接下来,通过计算特征之间的相关系数,进一步减少数据维度。对于相关系数≥0.90的特征对,保留更具预测性的特征,移除另一个特征。最后,剩下150个图像特征被选为具有稳健性、预测性和非冗余性的特征。
请以On the Criterion of Substantial Similarity of Artistic Works in American Law and the Controversy of Its Infringement Boundary为题用英语写一篇论文
On the Criterion of Substantial Similarity of Artistic Works in American Law and the Controversy of Its Infringement BoundaryAmerican law has long recognized that copyright protects creators from the unauthorized use of their artistic works. The law requires that there be a substantial similarity between the original work and any potential infringing work before the infringing work can be held liable for copyright infringement. In this paper, I will discuss the criteria used to determine substantial similarity in American law, the controversy surrounding its infringement boundary, and the implications of these issues for creators.To determine substantial similarity in American law, courts consider a variety of factors, including the purpose and character of the work, the similarity of the works, and the amount of copying that has taken place. The purpose and character of the work is generally determined by examining the source material, the original expression of ideas, and the similarities between the two works. The similarity of the works is assessed by considering the amount of copying that has occurred, the similarities in the subject matter, and the degree of similarity. Finally, the amount of copying is weighed by considering whether the work's substantial elements were copied, and how much of the work was copied.The controversy surrounding the substantial similarity of artistic works in American law is largely due to the fact that it is difficult to determine where to draw the line between legal and illegal copying. This is especially problematic for creators as it is often difficult to prove that their work has been copied by another artist. Additionally, the amount of copying that is considered to be infringing can vary from case to case, resulting in inconsistencies in the law.The implications of the substantial similarity of artistic works in American law are far-reaching. On one hand, it allows creators to protect their works by preventing others from using their ideas without permission. On the other hand, it can be used to stifle creativity by preventing new works from being created. Additionally, it can be used to prevent the dissemination of information, which can have a negative impact on the public's right to access knowledge and information.In conclusion, the substantial similarity of artistic works in American law is an important and complex issue. It is important for creators to be aware of the criteria used to determine substantial similarity and the controversy surrounding its infringement boundary. Additionally, they should be mindful of the implications of the substantial similarity of artistic works in American law and take steps to protect their works from potential infringement.
相关推荐
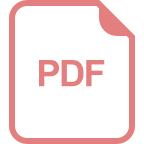
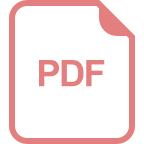
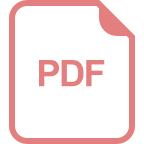







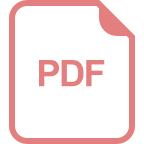
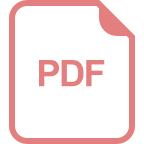
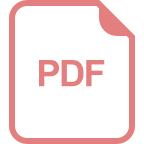
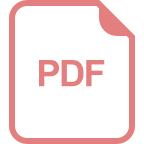
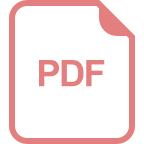
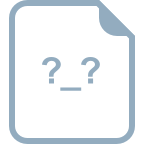