keras跟fastapi比较
时间: 2024-07-22 21:01:40 浏览: 98
Keras和FastAPI是两个完全不同的工具,分别服务于不同的领域。
Keras是一个高级神经网络 API,它允许用户快速构建和实验深度学习模型。它是基于Python的,并且通常与像TensorFlow或Theano这样的低级深度学习库结合使用。Keras专注于简化模型的定义、训练和评估过程,适用于机器学习任务,如图像分类、自然语言处理等。
FastAPI则是用于构建现代高性能web应用的Python框架。它专注于提供简洁、易用的API路由系统,以及快速的数据处理功能。FastAPI主要用于后端服务开发,特别是RESTful API的设计,使得开发者能够高效地构建和部署轻量级Web服务。
两者之间的区别在于:
1. Keras是数据科学库,而FastAPI是Web开发工具。
2. Keras关注的是模型构建,FastAPI关注的是HTTP请求的处理和响应。
3. 使用场景:Keras常用于构建复杂的机器学习模型,而FastAPI用于构建API驱动的应用程序。
相关问题
清除fastapi占用的显存
如果您在使用 FastAPI 期间遇到了显存占用过高的问题,可以尝试以下方法来清除 FastAPI 占用的显存:
1. 尝试使用 `del` 命令删除不再需要的变量和对象,以释放显存。
2. 在每个请求处理程序的末尾,尝试使用 `gc.collect()` 命令强制 Python 垃圾回收机制运行,以释放一些显存。
3. 如果您使用的是 TensorFlow 或 PyTorch 等深度学习框架,可以尝试使用 `tf.keras.backend.clear_session()` 或 `torch.cuda.empty_cache()` 命令来清除框架占用的显存。
如果以上方法都无法解决问题,您可能需要考虑使用更高性能的硬件,例如更多显存的 GPU 或 TPU。
keras summary
Keras is an open-source neural network library written in Python. It is designed to enable fast experimentation with deep neural networks and easy deployment to production. Keras provides a high-level API for building and training deep learning models.
The Keras summary method is used to provide a summary of the model architecture, including the number of parameters and the output shape of each layer. This summary can be useful for debugging and optimizing the model, as well as understanding its structure and behavior.
The Keras summary method takes an optional argument called "line_length," which specifies the maximum length of each line in the summary output. If the line length is too short, the summary may be split across multiple lines, making it difficult to read. If the line length is too long, the summary may become too wide to fit on the screen.
To use the Keras summary method, first create a Keras model by defining its layers and compiling it with an optimizer and loss function. Then, call the summary method on the model object:
```
from keras.models import Sequential
from keras.layers import Dense
# Define a simple Keras model
model = Sequential()
model.add(Dense(64, activation='relu', input_dim=100))
model.add(Dense(1, activation='sigmoid'))
# Compile the model with an optimizer and loss function
model.compile(optimizer='rmsprop',
loss='binary_crossentropy',
metrics=['accuracy'])
# Print a summary of the model architecture
model.summary()
```
The output of the summary method will look something like this:
```
Model: "sequential_1"
_________________________________________________________________
Layer (type) Output Shape Param #
=================================================================
dense_1 (Dense) (None, 64) 6464
_________________________________________________________________
dense_2 (Dense) (None, 1) 65
=================================================================
Total params: 6,529
Trainable params: 6,529
Non-trainable params: 0
_________________________________________________________________
```
This summary shows that the model has two layers, one with 64 neurons and one with 1 neuron, and a total of 6,529 parameters. It also shows the output shape of each layer, which is (None, 64) for the first layer and (None, 1) for the second layer. Finally, it shows the total number of trainable parameters and non-trainable parameters in the model.
阅读全文
相关推荐
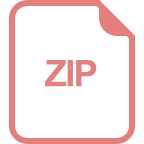
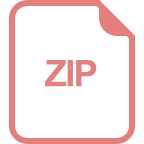
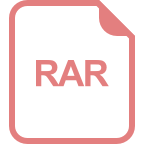













