激活名为unet的pyhon环境怎么操作
时间: 2024-01-09 10:03:30 浏览: 59
你可以按照以下步骤激活名为unet的python环境:
1. 打开终端(Windows用户打开命令提示符或PowerShell)
2. 输入以下命令:`conda activate unet`
3. 如果你使用的是虚拟环境而不是conda,输入以下命令:`source activate unet`
这样就可以激活名为unet的python环境了。请注意,你需要先安装conda或虚拟环境才能执行这些命令。
相关问题
现有一个unet网络名为model
好的,你现在已经有一个名为model的UNET网络。接下来你可以使用该网络对图像进行分割,代码示例如下:
```python
# 导入必要的库
import torch
from torchvision.transforms import ToTensor
from PIL import Image
# 加载图像并进行预处理
image = Image.open('test.jpg')
image_tensor = ToTensor()(image)
image_tensor = image_tensor.unsqueeze(0)
# 加载UNET网络模型
model = UNet()
model.load_state_dict(torch.load('model.pth'))
# 进行图像分割
with torch.no_grad():
output = model(image_tensor)
# 可视化分割结果
output = torch.argmax(output, dim=1)
output = output.squeeze().cpu().numpy()
plt.imshow(output, cmap='gray')
plt.show()
```
在这个示例中,我们首先导入了必要的库,并加载了一个待分割的图像。然后我们将图像转换为张量,并将其作为输入传递给UNET网络模型。接着我们使用``torch.no_grad()``上下文管理器来关闭梯度计算,以加速图像分割过程。最后我们将分割结果可视化展示出来。需要注意的是,这里我们假设使用的是一个名为``model.pth``的预训练模型,你需要将其替换成你自己的模型文件名。
unet图像去噪python
UNet是一种用于图像分割的深度学习网络,也可以用于图像去噪任务。在图像去噪任务中,UNet可以通过学习输入图像中的噪声分布,从而对噪声进行去除,保留图像中的有效信息。Python中可以使用TensorFlow或PyTorch等深度学习框架实现UNet图像去噪。
以下是一个基于PyTorch实现UNet图像去噪的简单示例:
```python
import torch
import torch.nn as nn
class UNet(nn.Module):
def __init__(self):
super(UNet, self).__init__()
# 定义编码器
self.encoder1 = nn.Sequential(
nn.Conv2d(1, 64, kernel_size=3, stride=1, padding=1),
nn.BatchNorm2d(64),
nn.ReLU(inplace=True),
nn.Conv2d(64, 64, kernel_size=3, stride=1, padding=1),
nn.BatchNorm2d(64),
nn.ReLU(inplace=True)
)
self.encoder2 = nn.Sequential(
nn.MaxPool2d(kernel_size=2, stride=2),
nn.Conv2d(64, 128, kernel_size=3, stride=1, padding=1),
nn.BatchNorm2d(128),
nn.ReLU(inplace=True),
nn.Conv2d(128, 128, kernel_size=3, stride=1, padding=1),
nn.BatchNorm2d(128),
nn.ReLU(inplace=True)
)
# 定义解码器
self.decoder1 = nn.Sequential(
nn.ConvTranspose2d(128, 64, kernel_size=2, stride=2),
nn.Conv2d(128, 64, kernel_size=3, stride=1, padding=1),
nn.BatchNorm2d(64),
nn.ReLU(inplace=True),
nn.Conv2d(64, 64, kernel_size=3, stride=1, padding=1),
nn.BatchNorm2d(64),
nn.ReLU(inplace=True)
)
self.decoder2 = nn.Sequential(
nn.ConvTranspose2d(64, 32, kernel_size=2, stride=2),
nn.Conv2d(64, 32, kernel_size=3, stride=1, padding=1),
nn.BatchNorm2d(32),
nn.ReLU(inplace=True),
nn.Conv2d(32, 32, kernel_size=3, stride=1, padding=1),
nn.BatchNorm2d(32),
nn.ReLU(inplace=True)
)
self.final_layer = nn.Conv2d(32, 1, kernel_size=1)
def forward(self, x):
# 编码器部分
x1 = self.encoder1(x)
x2 = self.encoder2(x1)
# 解码器部分
x = self.decoder1(torch.cat([x1, x2], dim=1))
x = self.decoder2(x)
# 输出结果
x = self.final_layer(x)
return x
```
相关问题:
1. 什么是UNet网络?
2. UNet网络可以用于哪些图像处理任务?
3. 如何在Python中实现UNet图像去噪?
4. UNet网络中的编码器和解码器分别有什么作用?
阅读全文
相关推荐
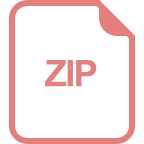
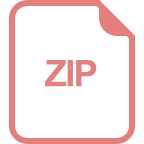
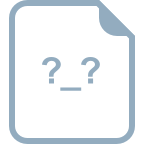













