using dataset:grazing of R 4. Show that overdispersion exists. 5. Fit a Quasi-Poisson model and fit a negative binomial GLM. 6. Compare the estimated coefficients, standard errors, significant tests of all three fitted models.
时间: 2024-03-23 17:39:36 浏览: 53
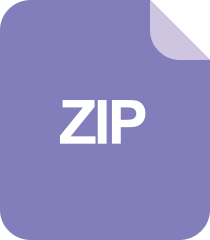
jcommon-1.0.23.jar_
Sure, here's how you can complete the remaining parts of your question:
4. To show that overdispersion exists in the 'grazing' dataset in R, you can fit a Poisson regression model to the data and then calculate the dispersion parameter. If the dispersion parameter is greater than 1, then overdispersion exists. Here's an example code:
```
library(MASS)
data(grazing)
# Fit a Poisson model
model_pois <- glm(count ~ period + offset(log(area)), data = grazing, family = poisson)
# Calculate dispersion parameter
dispersion <- sum(resid(model_pois, type = "pearson")^2) / df.residual(model_pois)
dispersion
```
If the dispersion parameter is greater than 1, then overdispersion exists.
5. To fit a Quasi-Poisson model and a negative binomial GLM, you can use the following code:
```
# Fit a Quasi-Poisson model
model_quasi <- glm(count ~ period + offset(log(area)), data = grazing, family = quasi(link = "log", variance = "mu"))
# Fit a negative binomial model
model_nb <- glm.nb(count ~ period + offset(log(area)), data = grazing)
```
6. To compare the estimated coefficients, standard errors, and significant tests of all three fitted models, you can use the `summary()` function to obtain the results and compare them. Here's an example code:
```
# Compare the three models
summary(model_pois)
summary(model_quasi)
summary(model_nb)
```
You can compare the estimated coefficients, standard errors, and p-values for each model to determine which one is the best fit for the data.
7. To produce diagnostic plots for the final chosen model, you can use the `plot()` function on the model object. Here's an example code:
```
# Diagnostic plots for the negative binomial model
plot(model_nb)
```
This will produce diagnostic plots for the negative binomial model, including a residual vs. fitted plot, a Q-Q plot, and a plot of deviance residuals vs. fitted values. You can use these plots to assess the goodness of fit of the model.
阅读全文
相关推荐
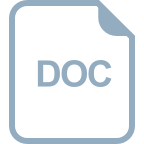
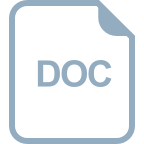



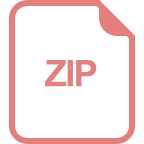
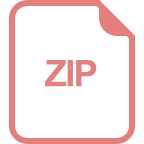
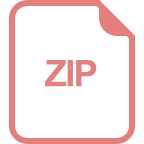
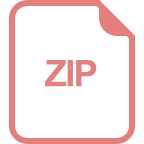

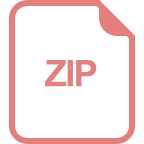
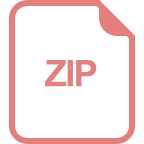
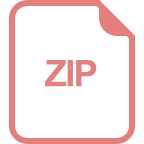
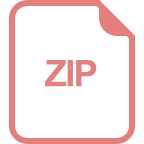
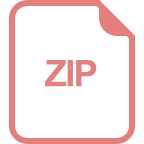
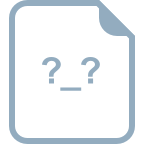
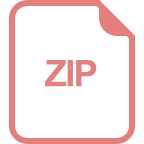
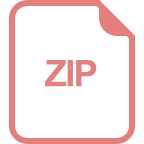
