{ "device_ids": [1000, 1001] // 服务器上终端的id, 可选参数device_ids和device_code 至少需要1个 "device_codes": ["7EC591C9"] // 终端code,可选参数device_ids和device_code至少需 要1个 "url": "http://121.37.139.172:8080/music/0117f14490b6bd0780f785756e078e5f.mp3", "sync": false, "queue": true, "volume": 50, // 播放⾳量:取值【1~100】 "loop": { ... }, "prompt": false }
时间: 2023-07-12 11:56:30 浏览: 40
这段代码是一个JSON格式的请求参数,包含以下字段:
- device_ids: 服务器上终端的id,类型为数组,可选参数,至少需要一个。
- device_codes: 终端code,类型为数组,可选参数,至少需要一个。
- url: 播放音频的URL地址。
- sync: 是否同步播放,类型为布尔值。
- queue: 是否加入播放队列,类型为布尔值。
- volume: 播放音量,取值范围为1-100。
- loop: 循环播放的参数,类型为对象。
- prompt: 是否播放提示音,类型为布尔值。
这些参数可以通过APIPOST等工具进行封装,以便在API中使用。
相关问题
for (DeviceId ids:deviceIds){ deviceTransferEntity.setDeviceId(ids.getId()); deviceTransferEntity.setDeviceProfileId(deviceProfile.getId().getId()); deviceTransferEntity.setTenantId(tenantId.getId()); deviceTransferEntity.setAuthorizationCode(authorizationCode); deviceTransferRepository.save(deviceTransferEntity); }为什么只插入了一条记录
可能是因为您的代码中创建了一个 `deviceTransferEntity` 对象,循环将其属性赋值后,仅仅保存了一次。因此,您需要在循环内部创建一个新的 `deviceTransferEntity` 对象。您可以尝试以下代码:
```
for (DeviceId ids : deviceIds) {
DeviceTransferEntity deviceTransferEntity = new DeviceTransferEntity();
deviceTransferEntity.setDeviceId(ids.getId());
deviceTransferEntity.setDeviceProfileId(deviceProfile.getId().getId());
deviceTransferEntity.setTenantId(tenantId.getId());
deviceTransferEntity.setAuthorizationCode(authorizationCode);
deviceTransferRepository.save(deviceTransferEntity);
}
```
这样,每次循环时都会创建一个新的 `deviceTransferEntity` 对象,并将其保存到数据库中。
from transformers import AutoTokenizer, AutoModel import torch import os # 设置代理环境变量 os.environ["HTTP_PROXY"] = "http://127.0.0.1:7890" os.environ["HTTPS_PROXY"] = "http://127.0.0.1:7890" # 选择一个预训练模型和对应的tokenizer model_name = "THUDM/chatglm2-6b-int4" tokenizer = AutoTokenizer.from_pretrained(model_name, trust_remote_code=True) model = AutoModel.from_pretrained(model_name, trust_remote_code=True) if tokenizer is None or model is None: raise ValueError("Failed to load tokenizer or model.") # 设置模型运行的设备 device = torch.device("cuda" if torch.cuda.is_available() else "cpu") model.to(device) while True: input_text = input("请输入您的问题:") answer = model.generate(tokenizer, input_text) print(f"答案是:{answer}")
感谢提供代码,现在我可以更好地理解问题了。
在你的代码中,你对预训练模型使用了 `model.generate()` 方法,这是正确的方法来生成对话回复。但是,在调用 `model.generate()` 时,你传递了 `tokenizer` 作为参数,这是不正确的。
`model.generate()` 方法接受一个输入序列的张量作为参数,而不是一个 tokenizer 对象。所以你需要先将输入文本编码为张量,然后再将其传递给 `model.generate()` 方法。
以下是修正后的代码:
```python
from transformers import AutoTokenizer, AutoModelForCausalLM
import torch
import os
# 设置代理环境变量
os.environ["HTTP_PROXY"] = "http://127.0.0.1:7890"
os.environ["HTTPS_PROXY"] = "http://127.0.0.1:7890"
# 选择一个预训练模型和对应的tokenizer
model_name = "THUDM/chatglm2-6b-int4"
tokenizer = AutoTokenizer.from_pretrained(model_name, trust_remote_code=True)
model = AutoModelForCausalLM.from_pretrained(model_name, trust_remote_code=True)
if tokenizer is None or model is None:
raise ValueError("Failed to load tokenizer or model.")
# 设置模型运行的设备
device = torch.device("cuda" if torch.cuda.is_available() else "cpu")
model.to(device)
while True:
input_text = input("请输入您的问题:")
input_ids = tokenizer.encode(input_text, return_tensors='pt').to(device)
outputs = model.generate(input_ids)
generated_text = tokenizer.decode(outputs[0], skip_special_tokens=True)
print(f"答案是:{generated_text}")
```
在修正后的代码中,我们首先使用 `tokenizer.encode()` 将输入文本编码为张量,然后使用 `model.generate()` 生成对话回复的张量。最后,我们使用 `tokenizer.decode()` 将生成的张量解码为可读文本。
请注意,`AutoModel` 类被替换为 `AutoModelForCausalLM` 类,因为 chatglm2-6b-int4 模型被定义为一个语言模型(Language Model)。
相关推荐
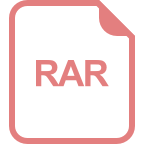
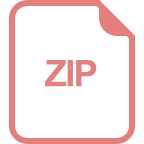
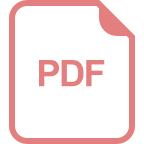




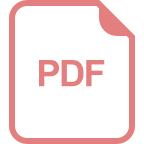
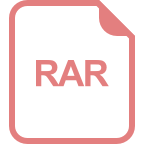
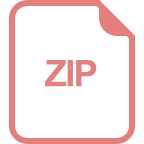
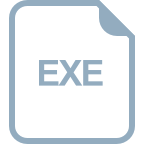
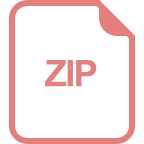
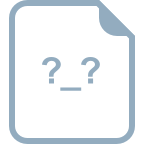
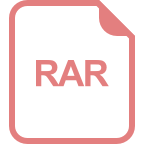
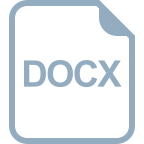