def file2matrix(filename):
时间: 2024-10-01 08:10:59 浏览: 29
`file2matrix`函数通常用于文本文件到二维数组的转换,常见于机器学习的数据预处理步骤中。这个函数的作用是从指定的文件中读取每一行的内容,并将其解析成一维向量,然后将所有行组合成一个矩阵。这里的"filename"参数就是你要处理的文件名。
以下是`file2matrix`函数的一个简单示例,假设文件是以空格分隔的逗号值对(如CSV文件):
```python
def file2matrix(filename):
# 打开文件并逐行读取
with open(filename, 'r') as f:
lines = f.readlines()
# 初始化一个空矩阵
data_matrix = []
# 对于文件中的每一行
for line in lines:
# 删除行尾的换行符,并分割成元素
row_data = line.strip().split(',')
# 将元素添加到当前行列表
data_matrix.append([float(element) for element in row_data])
return np.array(data_matrix)
```
这个函数返回的是一个numpy数组,方便进一步的数据分析和模型构建。
相关问题
def file2matrix(filename): fr = open(filename) numberOfLines = len(fr.readlines()) # get the number of lines in the file returnMat = zeros((numberOfLines, 3)) # prepare matrix to return classLabelVector = [] # prepare labels return fr = open(filename) index = 0 for line in fr.readlines(): line = line.strip() listFromLine = line.split('\t') returnMat[index, :] = listFromLine[0:3] classLabelVector.append(int(listFromLine[-1])) index += 1 return returnMat, classLabelVector解释一下这段代码
这段代码实现了从文本文件中读取数据,将数据存储到矩阵中,并返回矩阵和标签向量的函数。具体解释如下:
- `filename`:表示要读取的文件名。
- `fr = open(filename)`:打开文件。
- `numberOfLines = len(fr.readlines())`:读取文件中的所有行,并计算行数。
- `returnMat = zeros((numberOfLines, 3))`:根据行数创建一个大小为`(numberOfLines,3)`的零矩阵,用于存储数据。
- `classLabelVector = []`:创建一个空列表,用于存储标签向量。
- `fr = open(filename)`:再次打开文件。
- `index = 0`:初始化索引值为0。
- `for line in fr.readlines():`:遍历文件中的每一行。
- `line = line.strip()`:去掉行末的换行符。
- `listFromLine = line.split('\t')`:将当前行按制表符`\t`分割成一个列表。
- `returnMat[index, :] = listFromLine[0:3]`:将当前行的前三个元素存储到矩阵`returnMat`的第`index`行中。
- `classLabelVector.append(int(listFromLine[-1]))`:将当前行的最后一个元素(标签)转换成整数类型,并添加到标签向量`classLabelVector`中。
- `index += 1`:将索引值加1。
- `return returnMat, classLabelVector`:返回存储数据的矩阵`returnMat`和标签向量`classLabelVector`。
import numpy as np def replacezeroes(data): min_nonzero = np.min(data[np.nonzero(data)]) data[data == 0] = min_nonzero return data # Change the line below, based on U file # Foundation users set it to 20, ESI users set it to 21 LINE = 20 def read_scalar(filename): # Read file file = open(filename, 'r') lines_1 = file.readlines() file.close() num_cells_internal = int(lines_1[LINE].strip('\n')) lines_1 = lines_1[LINE + 2:LINE + 2 + num_cells_internal] for i in range(len(lines_1)): lines_1[i] = lines_1[i].strip('\n') field = np.asarray(lines_1).astype('double').reshape(num_cells_internal, 1) field = replacezeroes(field) return field def read_vector(filename): # Only x,y components file = open(filename, 'r') lines_1 = file.readlines() file.close() num_cells_internal = int(lines_1[LINE].strip('\n')) lines_1 = lines_1[LINE + 2:LINE + 2 + num_cells_internal] for i in range(len(lines_1)): lines_1[i] = lines_1[i].strip('\n') lines_1[i] = lines_1[i].strip('(') lines_1[i] = lines_1[i].strip(')') lines_1[i] = lines_1[i].split() field = np.asarray(lines_1).astype('double')[:, :2] return field if __name__ == '__main__': print('Velocity reader file') heights = [2.0, 1.5, 0.5, 0.75, 1.75, 1.25] total_dataset = [] # Read Cases for i, h in enumerate(heights, start=1): U = read_vector(f'U_{i}') nut = read_scalar(f'nut_{i}') cx = read_scalar(f'cx_{i}') cy = read_scalar(f'cy_{i}') h = np.ones(shape=(np.shape(U)[0], 1), dtype='double') * h temp_dataset = np.concatenate((U, cx, cy, h, nut), axis=-1) total_dataset.append(temp_dataset) total_dataset = np.reshape(total_dataset, (-1, 6)) print(total_dataset.shape) # Save data np.save('Total_dataset.npy', total_dataset) # Save the statistics of the data means = np.mean(total_dataset, axis=0).reshape(1, np.shape(total_dataset)[1]) stds = np.std(total_dataset, axis=0).reshape(1, np.shape(total_dataset)[1]) # Concatenate op_data = np.concatenate((means, stds), axis=0) np.savetxt('means', op_data, delimiter=' ') # Need to write out in OpenFOAM rectangular matrix format print('Means:') print(means) print('Stds:') print(stds)解析python代码,说明读取的数据文件格式
这段代码是一个用于读取数据文件的 Python 脚本。它包含了两个函数:`read_scalar(filename)` 和 `read_vector(filename)`,并使用了 `replacezeroes(data)` 函数来替换数组中的零值。脚本读取名为 `U_1`, `U_2`, ..., `U_6` 的文件,并根据文件中的数据生成一个包含各个高度的数据集。
函数 `read_scalar(filename)` 用于读取标量数据文件。它打开给定的文件,读取指定行数的数据,并将其存储在一个 NumPy 数组中。函数 `read_vector(filename)` 用于读取包含 x 和 y 分量的矢量数据文件。它也打开给定的文件,读取指定行数的数据,并将其存储在一个 NumPy 数组中。
在脚本的主程序中,一共读取了 6 个名为 `U`, `nut`, `cx`, `cy`, `h` 的文件,并将它们的数据分别存储在 `total_dataset` 列表中。然后,通过使用 NumPy 的函数将列表中的数据合并成一个包含 6 列的数组。最后,将合并后的数据保存为 `Total_dataset.npy` 文件,并计算并保存数据的均值和标准差。
这段代码假设数据文件的格式为文本文件,每行包含一个数据值。
阅读全文
相关推荐
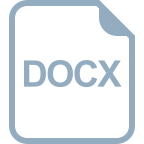
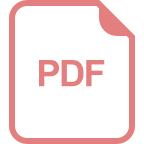
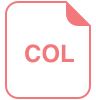
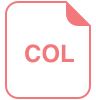
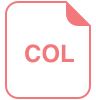
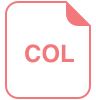
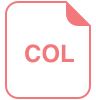
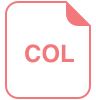
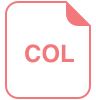
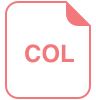
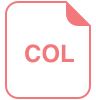





