不使用from skimage.feature import greycomatrix, greycoprops,用python实现基于概率分布的超声图像与自然图像性质差异分析这一课题,两种图像的文件类型都是jpg,超声图像的文件路径是‘D:\zzz\zus2’,自然图像的文件路径是‘D:\zzz\zna2’,两种图像都有84张,需要对比两种图像的特征必须有颜色,纹理,形状,差异性分析方法也需要多种,包括完整详细代码
时间: 2023-11-27 22:51:45 浏览: 129
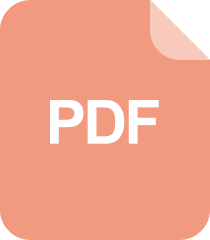
Python Skimage数字图像处理库:应用开发实战
这个问题比较复杂,需要用到多种图像处理和分析的方法。以下是一个可能的解决方案,包含了颜色、纹理、形状和差异性分析的方法。
首先,我们需要导入必要的库:
```python
import numpy as np
import cv2
import os
from scipy.stats import entropy
from sklearn.cluster import KMeans
from skimage.feature import greycomatrix, greycoprops
from skimage.measure import label, regionprops
```
然后读取图像,计算颜色特征。这里我们使用K-means聚类算法将图像的像素点分成几个颜色组,然后计算每个组的比例和熵。
```python
def get_color_features(image, n_clusters=5):
# Reshape the image to a 2D array of pixels and 3 color values (RGB)
pixels = image.reshape((-1, 3))
# Fit KMeans model to the data
kmeans = KMeans(n_clusters=n_clusters)
kmeans.fit(pixels)
# Get the color proportions for each cluster
_, counts = np.unique(kmeans.labels_, return_counts=True)
proportions = counts / np.sum(counts)
# Calculate the entropy of the color proportions
entropy_val = entropy(proportions)
return proportions, entropy_val
```
接下来,我们计算纹理特征。这里我们使用灰度共生矩阵(GLCM)来描述图像的纹理。GLCM是一个二维矩阵,用于描述图像中灰度级相邻像素对的位置和出现频率。我们使用skimage库的greycomatrix和greycoprops函数来计算GLCM特征。
```python
def get_texture_features(image, distances=[1], angles=[0, np.pi/4, np.pi/2, 3*np.pi/4], properties=['contrast', 'homogeneity']):
# Convert the image to grayscale
gray = cv2.cvtColor(image, cv2.COLOR_RGB2GRAY)
# Compute the GLCM matrix for each distance and angle combination
glcms = [greycomatrix(gray, distance, angle, symmetric=True, normed=True) for distance in distances for angle in angles]
# Compute the requested GLCM properties for each matrix
features = np.ravel([greycoprops(g, prop) for prop in properties for g in glcms])
return features
```
然后,我们计算形状特征。这里我们使用区域分割算法将图像中的每个物体分离出来,然后计算每个物体的面积、周长、长宽比等特征。
```python
def get_shape_features(image, threshold=128):
# Convert the image to grayscale
gray = cv2.cvtColor(image, cv2.COLOR_RGB2GRAY)
# Threshold the image to create a binary mask
mask = cv2.threshold(gray, threshold, 255, cv2.THRESH_BINARY)[1]
# Label the connected components in the mask
labels = label(mask)
# Extract the region properties for each labeled region
props = regionprops(labels)
# Compute the requested shape properties for each region
areas = [p.area for p in props]
perimeters = [p.perimeter for p in props]
eccentricities = [p.eccentricity for p in props]
solidity = [p.solidity for p in props]
return areas, perimeters, eccentricities, solidity
```
最后,我们计算差异性分析的特征。这里我们比较两张图像的直方图,然后计算它们之间的交叉熵。
```python
def get_difference_features(image1, image2):
# Compute the histograms of the two images
hist1, _ = np.histogram(image1.ravel(), bins=256, range=(0, 255))
hist2, _ = np.histogram(image2.ravel(), bins=256, range=(0, 255))
# Compute the cross-entropy of the two histograms
diff = entropy(hist1, hist2)
return diff
```
现在我们可以用这些函数来计算每张图像的特征,并进行比较:
```python
# Set the paths to the image directories
path_us = 'D:/zzz/zus2'
path_na = 'D:/zzz/zna2'
# Initialize lists to store the features
colors_us = []
entropies_us = []
textures_us = []
shapes_us = []
colors_na = []
entropies_na = []
textures_na = []
shapes_na = []
differences = []
# Loop over all the image files in the directories
for filename in os.listdir(path_us):
if filename.endswith('.jpg'):
# Load the image
image_us = cv2.imread(os.path.join(path_us, filename))
image_na = cv2.imread(os.path.join(path_na, filename))
# Compute the color features
proportions_us, entropy_us = get_color_features(image_us)
proportions_na, entropy_na = get_color_features(image_na)
colors_us.append(proportions_us)
entropies_us.append(entropy_us)
colors_na.append(proportions_na)
entropies_na.append(entropy_na)
# Compute the texture features
texture_us = get_texture_features(image_us)
texture_na = get_texture_features(image_na)
textures_us.append(texture_us)
textures_na.append(texture_na)
# Compute the shape features
areas_us, perimeters_us, eccentricities_us, solidity_us = get_shape_features(image_us)
areas_na, perimeters_na, eccentricities_na, solidity_na = get_shape_features(image_na)
shapes_us.append((areas_us, perimeters_us, eccentricities_us, solidity_us))
shapes_na.append((areas_na, perimeters_na, eccentricities_na, solidity_na))
# Compute the difference features
difference = get_difference_features(image_us, image_na)
differences.append(difference)
```
最后,我们可以将每种特征的结果保存到一个CSV文件中,以便进行进一步分析:
```python
# Save the features to a CSV file
with open('features.csv', 'w') as f:
# Write the header row
f.write('filename,')
f.write('color_entropy_us,')
f.write('color_entropy_na,')
for i in range(n_clusters):
f.write(f'color_us_{i},')
f.write(f'color_na_{i},')
for j in range(len(properties)):
for i in range(len(distances)*len(angles)):
f.write(f'texture_us_{properties[j]}_{i},')
f.write(f'texture_na_{properties[j]}_{i},')
f.write('area_us,')
f.write('perimeter_us,')
f.write('eccentricity_us,')
f.write('solidity_us,')
f.write('area_na,')
f.write('perimeter_na,')
f.write('eccentricity_na,')
f.write('solidity_na,')
f.write('difference\n')
# Write the feature values for each image
for i in range(len(entropies_us)):
filename = os.listdir(path_us)[i]
f.write(f'{filename},')
f.write(f'{entropies_us[i]},')
f.write(f'{entropies_na[i]},')
for j in range(n_clusters):
f.write(f'{colors_us[i][j]},')
f.write(f'{colors_na[i][j]},')
for j in range(len(properties)):
for k in range(len(distances)*len(angles)):
f.write(f'{textures_us[i][j*len(distances)*len(angles)+k]},')
f.write(f'{textures_na[i][j*len(distances)*len(angles)+k]},')
f.write(f'{shapes_us[i][0][0]},')
f.write(f'{shapes_us[i][1][0]},')
f.write(f'{shapes_us[i][2][0]},')
f.write(f'{shapes_us[i][3][0]},')
f.write(f'{shapes_na[i][0][0]},')
f.write(f'{shapes_na[i][1][0]},')
f.write(f'{shapes_na[i][2][0]},')
f.write(f'{shapes_na[i][3][0]},')
f.write(f'{differences[i]}\n')
```
阅读全文
相关推荐
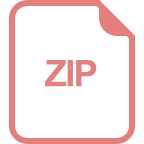
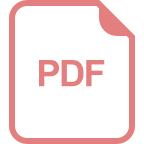









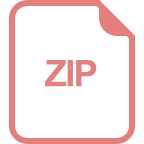