vanishing gradient problem
时间: 2023-04-29 11:06:29 浏览: 225
Vanishing Gradient Problem(梯度消失问题)是指在深度神经网络中,由于反向传播算法的特性,随着反向传播的深入,梯度会逐渐变得非常小,甚至趋近于零,导致神经网络无法继续学习或学习非常缓慢。这个问题通常出现在有很多层的神经网络中,而深度神经网络的优势往往正是在于其层数的增加,因此解决Vanishing Gradient Problem是深度学习中需要关注的重要问题之一。
相关问题
Compared with homogeneous network-based methods, het- erogeneous network-based treatment is closer to reality, due to the different kinds of entities with various kinds of relations [22– 24]. In recent years, knowledge graph (KG) has been utilized for data integration and federation [11, 17]. It allows the knowledge graph embedding (KGE) model to excel in the link prediction tasks [18, 19]. For example, Dai et al. provided a method using Wasser- stein adversarial autoencoder-based KGE, which can solve the problem of vanishing gradient on the discrete representation and exploit autoencoder to generate high-quality negative samples [20]. The SumGNN model proposed by Yu et al. succeeds in inte- grating external information of KG by combining high-quality fea- tures and multi-channel knowledge of the sub-graph [21]. Lin et al. proposed KGNN to predict DDI only based on triple facts of KG [66]. Although these methods have used KG information, only focusing on the triple facts or simple data fusion can limit performance and inductive capability [69]. Su et al. successively proposed two DDIs prediction methods [55, 56]. The first one is an end-to-end model called KG2ECapsule based on the biomedical knowledge graph (BKG), which can generate high-quality negative samples and make predictions through feature recursively propagating. Another one learns both drug attributes and triple facts based on attention to extract global representation and obtains good performance. However, these methods also have limited ability or ignore the merging of information from multiple perspectives. Apart from the above, the single perspective has many limitations, such as the need to ensure the integrity of related descriptions, just as network-based methods cannot process new nodes [65]. So, the methods only based on network are not inductive, causing limited generalization [69]. However, it can be alleviated by fully using the intrinsic property of the drug seen as local information, such as chemical structure (CS) [40]. And a handful of existing frameworks can effectively integrate multi-information without losing induction [69]. Thus, there is a necessity for us to propose an effective model to fully learn and fuse the local and global infor- mation for improving performance of DDI identification through multiple information complementing.是什么意思
这段文字讨论了异质网络方法相对于同质网络方法更接近现实的原因,并介绍了知识图谱和知识图谱嵌入模型在链接预测任务中的应用。作者提出了一些方法来解决多信息融合和归纳能力限制等问题,包括使用药物的局部信息如化学结构,以及利用多种信息来提高药物相互作用识别的性能。这段文字主要是关于药物相互作用预测领域的研究。
阅读全文
相关推荐
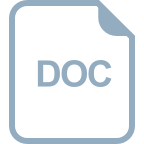
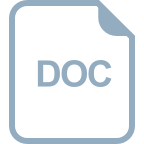
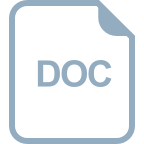
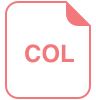
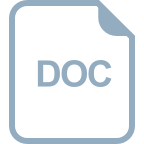
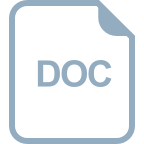
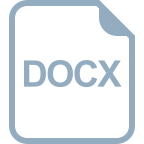
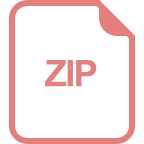
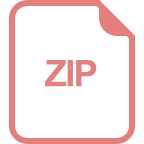
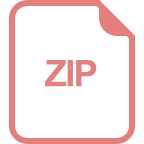
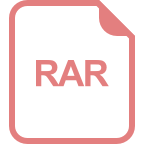
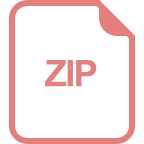
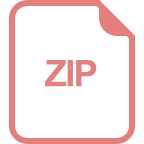
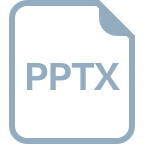
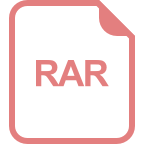