使用spark java类实现一个基于随机梯度下降算法的线性回归,不可以使用mllib包,其中输入数据是JavaRDD<Data> data类型,data中包含double list类型的x和double类型的y。实现两个函数,主函数public double[] iteration(JavaRDD<Data> data, int dimension, int epoch, double threshold)控制整个过程,其中data为输入数据,javaRDD<Data>类型,其中包括double []x和y,主方法中调用public double[] runStep(JavaRDD<Data> data, double[] weight, long count)实现每一轮的随机梯度下降
时间: 2024-02-28 15:52:02 浏览: 73
好的,以下是基于随机梯度下降算法的线性回归的实现代码,使用了Spark的Java API:
```java
import org.apache.spark.api.java.JavaRDD;
import org.apache.spark.api.java.function.Function;
import java.util.Random;
public class LinearRegressionSGD {
private static final Random random = new Random();
// 主函数,控制整个过程
public double[] iteration(JavaRDD<Data> data, int dimension, int epoch, double threshold) {
double[] weight = new double[dimension + 1];
long count = data.count();
double[] gradient = new double[dimension + 1];
for (int i = 0; i < epoch; i++) {
weight = runStep(data, weight, count);
gradient = computeGradient(data, weight, count);
// 判断梯度是否小于阈值
double norm = euclideanNorm(gradient);
if (norm < threshold) {
System.out.println("Converged after " + i + " iterations");
break;
}
}
return weight;
}
// 每一轮的随机梯度下降
public double[] runStep(JavaRDD<Data> data, double[] weight, long count) {
double[] newWeight = new double[weight.length];
// 遍历每个数据点
data.foreach((Function<Data, Void>) datum -> {
double[] x = datum.getX();
double y = datum.getY();
double prediction = dotProduct(x, weight);
double error = prediction - y;
// 更新权重
for (int i = 0; i < x.length; i++) {
newWeight[i] = weight[i] - error * x[i] / count;
}
newWeight[newWeight.length - 1] = weight[newWeight.length - 1] - error / count;
return null;
});
return newWeight;
}
// 计算梯度
public double[] computeGradient(JavaRDD<Data> data, double[] weight, long count) {
double[] gradient = new double[weight.length];
// 遍历每个数据点
data.foreach((Function<Data, Void>) datum -> {
double[] x = datum.getX();
double y = datum.getY();
double prediction = dotProduct(x, weight);
double error = prediction - y;
// 累加梯度
for (int i = 0; i < x.length; i++) {
gradient[i] += error * x[i] / count;
}
gradient[gradient.length - 1] += error / count;
return null;
});
return gradient;
}
// 计算点积
private double dotProduct(double[] a, double[] b) {
double result = 0.0;
for (int i = 0; i < a.length; i++) {
result += a[i] * b[i];
}
return result;
}
// 计算欧几里得范数
private double euclideanNorm(double[] a) {
double sum = 0.0;
for (double value : a) {
sum += value * value;
}
return Math.sqrt(sum);
}
// 数据类
public static class Data {
private double[] x;
private double y;
public Data(double[] x, double y) {
this.x = x;
this.y = y;
}
public double[] getX() {
return x;
}
public double getY() {
return y;
}
}
}
```
其中,主函数 `iteration` 控制整个过程。每一轮的随机梯度下降通过函数 `runStep` 实现,计算梯度通过函数 `computeGradient` 实现。在计算梯度时,我们遍历每个数据点并累加梯度,最后返回梯度向量。在每一轮的随机梯度下降中,我们遍历每个数据点并更新权重。在更新权重时,我们计算每个数据点对于权重的贡献,并累加更新权重,最后返回新的权重向量。对于计算点积和欧几里得范数的函数,我们使用了简单的循环实现。
阅读全文
相关推荐



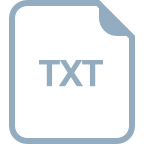
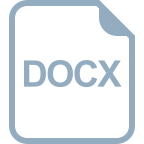
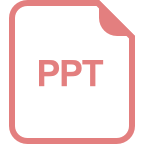
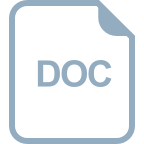
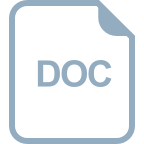
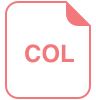
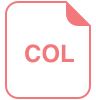
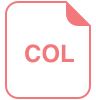
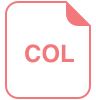
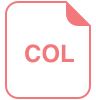
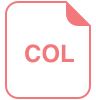
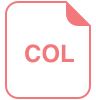
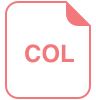
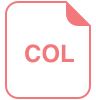