list_name = 'list_' + str(i) list_name = []
时间: 2024-03-19 17:45:31 浏览: 25
这段代码中有两行,第一行是 `list_name = 'list_' + str(i)`,第二行是 `list_name = []`。这两行代码的意义是不同的。
第一行代码将字符串 `'list_'` 和整数 `i` 拼接起来,得到一个新的字符串,赋值给变量 `list_name`。例如,如果 `i` 的值为 `3`,则执行这行代码后 `list_name` 的值为 `'list_3'`。这里的目的是为了创建一个变量名,以便后续使用。
第二行代码将一个空列表赋值给变量 `list_name`。这里的作用是创建一个新的列表,并将其赋值给变量 `list_name`,以便后续使用。这个新的列表和变量名 `list_name` 与第一行代码中创建的字符串没有任何关系,它们是完全独立的。因此,如果想要将这个新的列表命名为 `'list_' + str(i)`,可以使用字典来实现:
```
lists = {}
i = 3
lists['list_' + str(i)] = []
```
这里使用了一个空的字典 `lists`,将变量名 `'list_' + str(i)` 作为字典的键,将空列表作为字典的值,将它们关联起来并存储在字典中。这样就实现了将一个新的列表命名为 `'list_' + str(i)` 的功能。
相关问题
优化下列python代码中的递归函数:def words_divi(words, n): division_words = words / n print(division_words) if division_words <= words_max: if isinstance(division_words, int): for i in range(n): sram_list.append(str(division_words) + 'x' + str(bits_max)) else: round(division_words) if division_words in words_list: for i in range(n): sram_list.append(str(division_words) + 'x' + str(bits_max)) else: while division_words < words_max: division_words = division_words + 1 if division_words in words_list: for i in range(n): sram_list.append(str(division_words) + 'x' + str(bits_max)) else: n = n + 1 print(n) words_divi(words, n) if __name__ == "__main__": words_list = [] bits_list = [] words_list.append(list(range(32, 1025, 16)) + list(range(1056, 8193, 16))) bits_list.append(list(range(16, 145, 1))) words = 16388 bits = 148 words_max = max(words_list[0]) bits_max = max(bits_list[0]) words_min = min(words_list[0]) bits_min = min(bits_list[0]) sram_list = [] num = 2 words_divi(words, num) print(sram_list)
可以使用尾递归优化来优化该递归函数,避免栈溢出的问题。具体实现方法是将递归函数中的参数改为可变参数,并将递归调用改为参数的更新,直到满足递归终止条件。以下是优化后的代码:
def words_divi(words, n, sram_list):
division_words = words / n
print(division_words)
if division_words <= words_max:
if isinstance(division_words, int):
for i in range(n):
sram_list.append(str(division_words) + 'x' + str(bits_max))
else:
round(division_words)
if division_words in words_list:
for i in range(n):
sram_list.append(str(division_words) + 'x' + str(bits_max))
else:
while division_words < words_max:
division_words = division_words + 1
if division_words in words_list:
for i in range(n):
sram_list.append(str(division_words) + 'x' + str(bits_max))
return sram_list
else:
return words_divi(words, n+1, sram_list)
if __name__ == "__main__":
words_list = []
bits_list = []
words_list.append(list(range(32, 1025, 16)) + list(range(1056, 8193, 16)))
bits_list.append(list(range(16, 145, 1)))
words = 16388
bits = 148
words_max = max(words_list[0])
bits_max = max(bits_list[0])
words_min = min(words_list[0])
bits_min = min(bits_list[0])
sram_list = []
num = 2
sram_list = words_divi(words, num, sram_list)
print(sram_list)
def run_gevent(ip_list): issue_device = [] try: hwconfig = get_config(ip_list) write_config_to_file(hwconfig,ip_list) except (AuthenticationException): issue_message = (ip_list + ': 认证错误 ') issue_device.append(issue_message) except NetMikoTimeoutException: issue_message = (ip_list + ': 网络不可达 ') issue_device.append(issue_message) except (SSHException): issue_message = (ip_list +': SSH端口异常 ') issue_device.append(issue_message) except Exception as unknown_error: issue_message = (ip_list +': 发生未知错误: ') issue_device.append(issue_message+str(unknown_error)) finally: write_issue_device(issue_device) def main(): starting_time = time() ip_list = read_device_excel() pool = Pool(50) pool.map(run_gevent,ip_list) #map(func, iterable) pool.join() print ('\n---- End get config threading, elapsed time=', time() - starting_time) 这里之前会产生报错TypeError: can only concatenate tuple (not "str") to tuple请问如何修改,其中ip_list.append((ipaddr,ntos,name,pswd,epswd))
这个错误是因为你在以下代码中尝试将字符串和元组连接:
```
issue_device.append(issue_message+str(unknown_error))
```
要解决这个问题,可以将 `issue_message` 从字符串转换为元组:
```
issue_message = (ip_list +': 发生未知错误: ',)
```
然后在连接时使用元组:
```
issue_device.append(issue_message + (str(unknown_error),))
```
这应该可以解决报错问题。关于另一个问题,如果你想将 `(ipaddr, ntos, name, pswd, epswd)` 添加到 `ip_list` 中,你可以使用以下代码:
```
ip_list.append((ipaddr, ntos, name, pswd, epswd))
```
这将把这个元组添加到 `ip_list` 中。
相关推荐
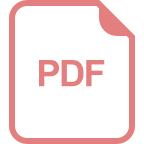
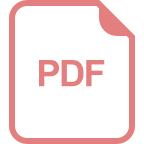
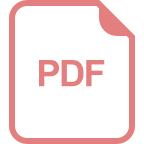













