mg_crop = img_read.crop((x, y, x + w, y + h)) image_save_name = image_path_name.split ('/')[-2] + '_' + \ image_path_name.split ('/')[-1].split ('.')[0] image_save_path = dataset_image_path.rsplit('/', 1)[0] image_save_path_name = image_save_path + '/' + image_save_name + \ '_crop_' + str ( x) + '-' + str (y) + '-' + str (x + w) + '-' + str ( y + h) + '_iou_' + str (iou) + '.jpg' logging.debug('image_save_path_name {}'.format(image_save_path_name)) img_crop.save(image_save_path_name) logging.debug('img_crop {} {} {}'.format(img_crop.format, img_crop.size, img_crop.mode)) 报错:ValueError: cannot write empty image as JPEG
时间: 2024-02-23 15:00:06 浏览: 23
这个错误通常是因为你尝试将一个空的图像保存为JPEG格式。这可能是由于你在裁剪过程中没有正确处理图像数据导致的。你可以在保存图像之前,加上以下代码来检查图像是否为空:
```
if img_crop.mode != "RGB" or img_crop.size[0] == 0 or img_crop.size[1] == 0:
print("Error: empty or invalid image")
else:
img_crop.save(image_save_path_name)
```
这样可以避免保存空图像的情况。如果问题仍然存在,你可以检查一下你的图像处理代码,确保它能够正确地加载和处理图像数据。
相关问题
left_img = img.copy().crop(left_roi)
这是一个关于图像处理的问题,left_img 是一个从 img 中裁剪出来的左侧区域的图像,crop() 方法用于裁剪图像,left_roi 是一个表示左侧区域的矩形框。如果需要完整的代码,需要了解 img 和 left_roi 的具体定义和实现方式。
img_crop = img_read.crop( (x1, y1, x2, y2))
这段代码是用于裁剪图片。代码中使用了 Image.crop() 函数来对图片进行裁剪,该函数需要传入一个元组作为参数,表示裁剪的区域。元组中的四个值分别表示左上角坐标的 x 和 y 值,以及右下角坐标的 x 和 y 值,即 (x1, y1, x2, y2)。通过这样的方式,代码可以将图片裁剪成边界框所包含的区域。裁剪后得到的图像保存在 img_crop 变量中。
相关推荐
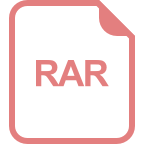











