将神经网络部署到网页中需要什么样的方法?请给我具体的详细流程
时间: 2024-03-06 13:51:33 浏览: 18
将神经网络部署到网页中的方法可以分为以下几个步骤:
1.训练模型:首先需要使用神经网络框架(例如TensorFlow、PyTorch等)训练模型,得到可以用于预测的权重和参数。
2.导出模型:将训练好的模型导出为可用于推理的格式,例如TensorFlow.js的SavedModel或Keras的HDF5格式。
3.加载模型:在网页中使用JavaScript加载训练好的模型。
4.预处理输入:将用户输入的数据进行预处理,使其符合模型的输入格式。
5.进行推理:使用加载的模型对预处理后的输入进行推理,并得到模型的输出结果。
6.后处理输出:对模型的输出结果进行后处理,例如将数字转换为标签或可视化输出结果。
具体的流程如下:
1.训练模型:使用神经网络框架训练模型,得到可以用于预测的权重和参数。
2.导出模型:将训练好的模型导出为可用于推理的格式,例如TensorFlow.js的SavedModel或Keras的HDF5格式。
3.加载模型:使用JavaScript加载导出的模型。可以通过以下代码加载TensorFlow.js模型:
```javascript
async function loadModel() {
const model = await tf.loadLayersModel('model.json');
return model;
}
```
4.预处理输入:使用JavaScript将用户输入的数据进行预处理,使其符合模型的输入格式。例如,如果要使用图像分类模型,可以使用以下代码将图像转换为模型所需的张量格式:
```javascript
function preprocessImage(image) {
// Resize the image to 224x224 and convert to a tensor
const tensor = tf.browser.fromPixels(image)
.resizeNearestNeighbor([224, 224])
.toFloat();
// Normalize the pixel values
const offset = tf.scalar(255.0);
const normalized = tensor.div(offset);
// Add a batch dimension and return
return normalized.expandDims();
}
```
5.进行推理:使用加载的模型对预处理后的输入进行推理,并得到模型的输出结果。例如,使用以下代码对预处理后的图像进行分类:
```javascript
async function predictImage(image, model) {
// Preprocess the image
const tensor = preprocessImage(image);
// Make the prediction
const prediction = await model.predict(tensor).data();
// Get the top predicted class and confidence
const topClass = prediction.indexOf(Math.max(...prediction));
const confidence = prediction[topClass];
// Return the result
return { classIndex: topClass, confidence: confidence };
}
```
6.后处理输出:对模型的输出结果进行后处理,例如将数字转换为标签或可视化输出结果。
以上是将神经网络部署到网页中的简要流程,具体实现可能会因模型和应用场景的不同而有所差异。
相关推荐
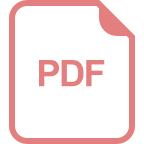
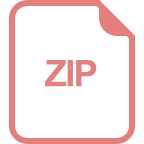
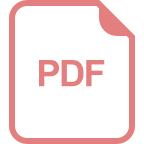














