xgboost中怎么自定义损失函数focal loss
时间: 2023-05-22 14:05:07 浏览: 566
在XGBoost中自定义损失函数Focal Loss的步骤如下:
Step 1:定义Focal Loss的公式,Focal Loss由两部分组成,一部分是二元交叉熵损失,另一部分是focusing参数。
Step 2:用函数定义Focal Loss的代码。
Step 3:在XGBoost中使用Python类似于Lambda函数的自定义函数功能去载入这个Focal Loss函数。这个函数可以自定义为Objective函数。
如果您需要更详细的内容,可以在网上,特别是GitHub上找到关于自定义Objective函数的代码和示例。
相关问题
xgboost 自定义损失函数
XGBoost是一种常用的梯度提升框架,在分类和回归问题中具有广泛的应用。它是一种基于决策树的模型,通过迭代地提高每个决策树的预测能力,最终得到一个强大的集成模型。XGBoost支持自定义损失函数,使得用户可以根据自己的需求来定义损失函数。
在XGBoost中,损失函数的定义是通过构建一个二阶泰勒展开式得到的。具体而言,假设我们要定义一个自定义的损失函数$L(y,\hat{y})$,其中$y$是真实值,$\hat{y}$是预测值。那么,我们可以通过以下方式来构建损失函数:
1. 定义一阶导数和二阶导数
$$
g_i=\frac{\partial L(y_i,\hat{y}_i)}{\partial \hat{y}_i}\\
h_i=\frac{\partial^2 L(y_i,\hat{y}_i)}{\partial \hat{y}_i^2}
$$
其中$i$表示样本的索引,$g_i$是损失函数$L(y_i,\hat{y_i})$在$\hat{y_i}$处的一阶导数,$h_i$是损失函数$L(y_i,\hat{y_i})$在$\hat{y_i}$处的二阶导数。
2. 在XGBoost的目标函数中引入自定义的损失函数
$$
Obj(\theta)=\sum_{i=1}^nl(y_i,\hat{y}_i)+\sum_{i=1}^t\Omega(f_i)+\gamma T
$$
其中$l(y_i,\hat{y}_i)$是样本$i$的损失函数,$\Omega(f_i)$是树$f_i$的正则化项,$\gamma$是正则化参数,$T$是树的数量。对于分类问题,$l(y_i,\hat{y}_i)$可以是对数似然损失函数或指数损失函数等;对于回归问题,$l(y_i,\hat{y}_i)$可以是平方损失函数或绝对损失函数等。
3. 将自定义的损失函数表示成$g_i$和$h_i$的形式
为了将自定义的损失函数$L(y,\hat{y})$表示成$g_i$和$h_i$的形式,我们需要对$L(y,\hat{y})$进行二阶泰勒展开:
$$
L(y,\hat{y})\approx \sum_{i=1}^n\left[L(y_i,\hat{y}_i)+g_i(\hat{y}_i-\hat{y})+\frac{1}{2}h_i(\hat{y}_i-\hat{y})^2\right]
$$
4. 实现自定义的损失函数
将自定义的损失函数表示成$g_i$和$h_i$的形式后,我们可以将它们带入XGBoost的目标函数中,从而实现自定义的损失函数。具体而言,我们需要重载XGBoost中的两个函数:
* \_\_call\_\_(self, preds, labels)
* create\_obj(self)
第一个函数用于计算预测值和真实值的损失函数值,第二个函数用于创建自定义的目标函数。在这两个函数中,我们需要根据自定义的损失函数来计算$g_i$和$h_i$,并将它们传递给XGBoost的目标函数。
下面是一个简单的例子,展示了如何在XGBoost中实现自定义的损失函数:
```python
import xgboost as xgb
import numpy as np
# 定义自定义的损失函数
def my_loss(y_true, y_pred):
diff = y_true - y_pred
grad = -2 * diff
hess = 2 * np.ones_like(y_true)
return grad, hess
# 实现自定义的目标函数
class MyObjective(xgb.core.ObjFunction):
def __call__(self, preds, labels):
grad, hess = my_loss(labels, preds)
return grad, hess
def create_obj(self):
return self
# 模拟数据
X = np.random.normal(size=(100, 10))
y = np.random.normal(size=100)
# 定义模型
params = {
'objective': MyObjective(),
'eval_metric': 'rmse',
'max_depth': 3,
'learning_rate': 0.1,
'n_estimators': 100
}
model = xgb.XGBRegressor(**params)
# 训练模型
model.fit(X, y)
```
在上面的代码中,我们定义了一个自定义的损失函数`my_loss`,它计算每个样本的一阶导数和二阶导数。然后,我们实现了一个自定义的目标函数`MyObjective`,它将自定义的损失函数传递给XGBoost的目标函数。最后,我们使用这个自定义的目标函数来训练一个XGBoost回归模型。
xgboost自定义损失函数
对于XGBoost,可以使用自定义损失函数来训练模型。以下是一个使用自定义损失函数训练XGBoost模型的示例代码:
```python
import xgboost as xgb
这个损失函数必须是可微分的,并且能够计算梯度和二阶导数。你可以根据具体的问题定义你自己的损失函数,例如:
```python
import numpy as np
相关推荐
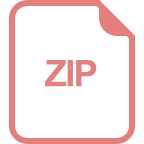
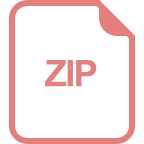
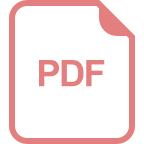
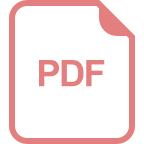
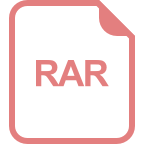
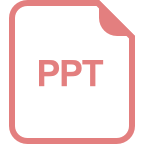
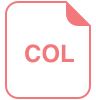
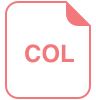
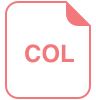
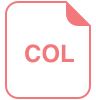
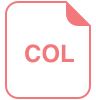




