vrp路径规划 python
时间: 2023-05-15 15:02:48 浏览: 215
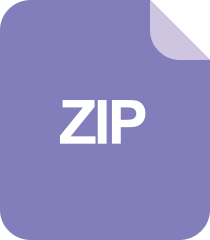
python毕设路径规划

VRP(Vehicle Routing Problem)路径规划是指在物流或快递配送等领域中,利用客户需求、车辆载重、时间窗口等因素,对车辆路线进行最优化规划的问题。Python是一种高级编程语言,广泛应用于科学、数据分析和人工智能等领域。
在Python中进行VRP路径规划可以应用多种算法,如贪心算法、遗传算法、模拟退火算法、蚁群算法等。其中,蚁群算法应用广泛,是一种模拟自然界蚂蚁群体寻食行为的搜索算法,能够有效地解决VRP路径规划问题。
Python中实现VRP路径规划需要注意以下几点:
1.数据输入
需要输入客户需求、车辆容量、时间窗口等相关数据,并将其存储在数组或字典等数据结构中。
2.计算距离和时间
需要计算各客户之间的距离和时间,可以使用OpenStreetMap等地图API进行计算。
3.编写优化函数
需要编写优化函数,将输入数据和计算结果进行处理,得到最优路径规划方案。
4.可视化结果
可以通过Matplotlib等可视化库将路径规划结果可视化展示,方便用户直观查看。
总之,VRP路径规划是Python应用的一种重要场景,具有广泛应用前景。
阅读全文
相关推荐
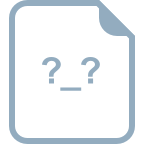
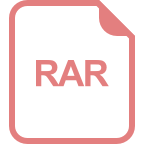
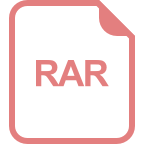
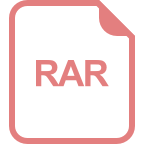
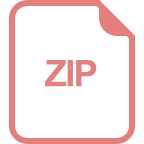
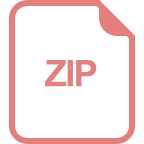
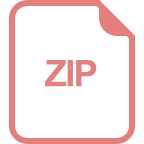




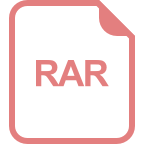




