猫狗图像分类python代码
时间: 2023-08-23 21:07:01 浏览: 52
以下是使用Python编写的猫狗图像分类代码:
首先需要导入必要的包:
```python
import numpy as np
import matplotlib.pyplot as plt
import os
import cv2
from sklearn.model_selection import train_test_split
from keras.utils import to_categorical
from keras.models import Sequential, load_model
from keras.layers import Conv2D, MaxPool2D, Dense, Flatten, Dropout
```
接着,我们需要读入数据集:
```python
DATADIR = "dataset"
CATEGORIES = ["cats", "dogs"]
IMG_SIZE = 50
training_data = []
def create_training_data():
for category in CATEGORIES:
path = os.path.join(DATADIR, category)
class_num = CATEGORIES.index(category)
for img in os.listdir(path):
try:
img_array = cv2.imread(os.path.join(path, img), cv2.IMREAD_GRAYSCALE)
new_array = cv2.resize(img_array, (IMG_SIZE, IMG_SIZE))
training_data.append([new_array, class_num])
except Exception as e:
pass
create_training_data()
X = []
y = []
for features, label in training_data:
X.append(features)
y.append(label)
X = np.array(X).reshape(-1, IMG_SIZE, IMG_SIZE, 1)
X = X/255.0
y = to_categorical(y, num_classes=2)
X_train, X_test, y_train, y_test = train_test_split(X, y, test_size=0.2, random_state=42)
```
在这里,我们使用了OpenCV库来读取和处理图像,然后将它们添加到训练数据中。我们还使用了sklearn库中的train_test_split函数来将数据集划分为训练集和测试集。最后,我们将图像和标签转换成numpy数组,并将像素值缩放到0到1之间。
接下来,我们可以构建模型:
```python
model = Sequential()
model.add(Conv2D(32, (3,3), activation='relu', input_shape=X_train.shape[1:]))
model.add(MaxPool2D(pool_size=(2,2)))
model.add(Dropout(0.25))
model.add(Conv2D(64, (3,3), activation='relu'))
model.add(MaxPool2D(pool_size=(2,2)))
model.add(Dropout(0.25))
model.add(Flatten())
model.add(Dense(128, activation='relu'))
model.add(Dropout(0.5))
model.add(Dense(2, activation='softmax'))
model.compile(optimizer='adam', loss='categorical_crossentropy', metrics=['accuracy'])
```
这个模型使用了卷积神经网络的结构,包含两个卷积层和一个全连接层。我们使用了ReLU激活函数和MaxPooling层来增加模型的非线性能力。Dropout层用于减少过拟合。
最后,我们可以训练模型并评估其性能:
```python
history = model.fit(X_train, y_train, batch_size=32, epochs=20, validation_data=(X_test, y_test))
model.save('cat_dog_classifier.h5')
test_loss, test_acc = model.evaluate(X_test, y_test)
print('Test loss:', test_loss)
print('Test accuracy:', test_acc)
```
这里我们使用了keras库来训练模型。我们还保存了训练好的模型,并计算了模型在测试集上的准确率。
相关推荐
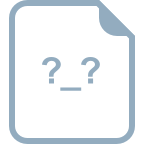
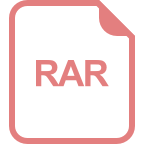
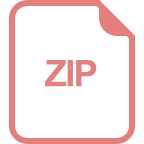














