rrt 机械臂 python
时间: 2023-11-28 21:02:27 浏览: 174
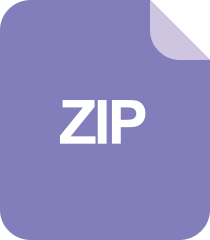
ROS机械臂运动控制(完整项目代码python)

RRt 是机器人路径规划算法中的一种,它的全称是"Rapidly-exploring Random Trees"。机械臂是一种可编程的机械设备,用于执行各种复杂的动作和任务。而Python是一种高级编程语言,被广泛用于机器学习、人工智能和机器人控制等领域。
在机械臂控制领域,RRt 算法可以应用于路径规划,以确定机械臂在给定环境中执行任务时的最佳路径。RRt 算法的特点是通过随机探索的方式构建一个树形结构,以产生一系列可能的路径。通过遍历这个树形结构,RRt 算法可以找到到达目标位置的最佳路径。
Python 是一种非常强大的编程语言,它提供了丰富的库和工具,可以帮助我们实现机械臂控制和路径规划。在使用 Python 进行机械臂控制时,我们可以利用 Python 的库来调用机械臂的驱动程序,并编写相应的控制算法。同时,Python 也提供了一些常用的机器人学和运动规划的库,例如 MoveIt 和 Pybullet,可以方便地实现机械臂的路径规划和运动控制。
综上所述,RRt 算法可以与 Python 结合使用,用于机械臂的路径规划和控制。通过使用 Python,我们可以更方便地开发出高效且灵活的机械臂控制系统,使机械臂能够在复杂的环境中准确地执行各种任务。
阅读全文
相关推荐
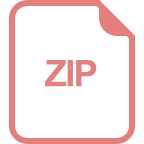
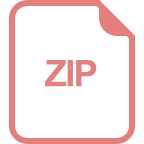
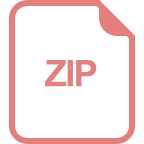
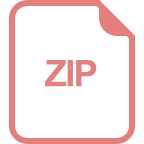
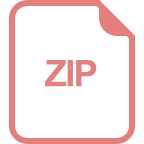
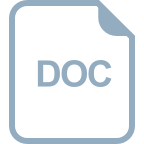

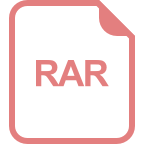
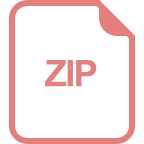
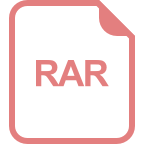
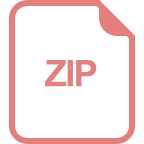
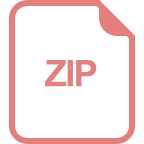
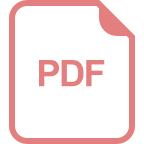
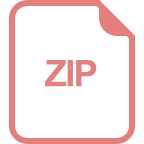
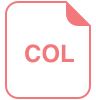

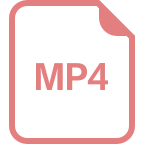
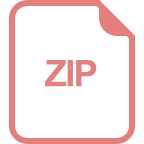