为什么对ResNet34模型单独使用large-batch training, learning rate warmup, no bias decay, low-precision training和共同使用large-batch training与learning rate warmup和large-batch training与low-precision training时模型性能会得到提升,
时间: 2023-11-04 21:04:25 浏览: 53
单独使用large-batch training可以增加模型的训练效率和稳定性,这是因为在大批量数据的情况下,梯度下降的方向更加准确,能够更快地收敛到最优解。同时,使用large-batch training还可以减少模型的震荡、提升泛化能力、加速模型训练的过程。而learning rate warmup可以在模型训练初期逐渐增加学习率,从而避免在训练初期梯度过大导致模型无法收敛的问题。no bias decay可以减少模型的过拟合,从而提高模型的泛化能力。low-precision training则可以在不影响模型精度的情况下,减少计算和内存开销,加速模型训练过程。
共同使用large-batch training与learning rate warmup和large-batch training与low-precision training可以相互配合,进一步提高模型的训练效率和准确性。例如,使用learning rate warmup可以使模型更快地收敛,而使用low-precision training可以减少计算和内存开销,降低训练时间和成本。同时,large-batch training可以提高模型的训练效率和稳定性,从而进一步提高模型的准确性。因此,这些技巧和策略的相互配合可以帮助我们更好地训练深度学习模型,提高模型的性能。
相关问题
为什么对ResNet34模型单独使用Linear scaling learning rate和共同使用Large-batch training与 Linear scaling learning rate和Large-batch training与No bias decay时模型性能反而下降
单独使用Linear scaling learning rate时,会使学习率随着batch size的增加而线性增加,这样可以有效地缓解large-batch training可能带来的梯度消失或梯度爆炸等问题,从而进一步提高模型的性能。然而,如果仅仅使用Linear scaling learning rate而没有配合使用其他技巧,可能会导致模型的过拟合和训练不稳定等问题,从而降低模型的性能。
共同使用Large-batch training与 Linear scaling learning rate时,会使学习率与batch size成正比例关系,并且随着batch size的增加而逐渐增大,这样可以更加有效地缓解large-batch training所带来的问题,同时也可以加速模型的训练过程,提高模型的性能。然而,如果同时使用No bias decay,可能会导致模型过拟合,并且使模型的泛化能力下降,从而降低模型的性能。
因此,在实践中,需要根据具体情况选择适当的技巧和策略,并进行细致的实验和分析,以获得最佳的模型性能。
为什么对ResNet34模型分别单独使用cosine learning rate decay, mixup training时,模型性能下降
对于ResNet34模型,单独使用cosine learning rate decay和mixup training可能会导致模型性能下降的原因可能是以下几点:
1. 学习率调度和数据增强策略的超参数选择不当。例如,学习率调度中的最大学习率和最小学习率设置过高或过低,或者mixup的参数设置不合适,都可能会影响模型的性能。
2. ResNet34模型本身的特性。由于模型的结构和参数特点,某些训练策略可能对其效果不明显甚至会产生负面影响。
3. 模型训练时存在的其他因素。例如,数据集的质量、训练集和验证集的划分等都可能会影响模型的性能。
因此,需要进行更加深入的实验和分析才能确定具体原因。同时,建议在使用不同的训练策略时,要进行仔细的实验设计和结果分析,以确定最佳的训练策略。
相关推荐
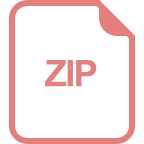












