with torch.no_grad
时间: 2023-04-13 10:04:44 浏览: 110
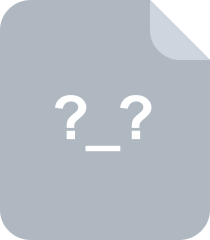
0695-极智开发-解读pytorch中with torch.no-grad()的作用
with torch.no_grad是PyTorch中的一个上下文管理器,用于在评估模型时禁用梯度计算,以减少内存消耗和加速计算。在这个上下文中,所有的张量操作都不会被记录在计算图中,也不会计算梯度。这个上下文管理器通常用于测试和验证模型,以避免不必要的计算和内存消耗。
阅读全文
相关推荐
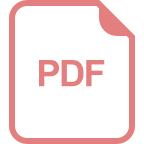
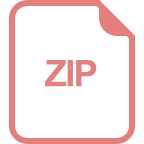






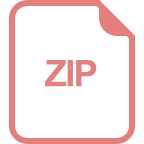
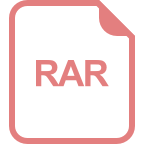
0695-极智开发-解读pytorch中with torch.no-grad()的作用