pandas对一个excel中的多个sheet做筛选字段操作
时间: 2023-05-11 19:06:43 浏览: 143
可以使用pandas的read_excel函数读取excel文件,并使用sheet_name参数指定要读取的sheet名称或索引。然后,使用pandas的DataFrame的filter函数对数据进行筛选操作。例如,可以使用以下代码对名为Sheet1和Sheet2的两个sheet中的数据进行筛选操作:
import pandas as pd
# 读取excel文件
df1 = pd.read_excel('example.xlsx', sheet_name='Sheet1')
df2 = pd.read_excel('example.xlsx', sheet_name='Sheet2')
# 筛选操作
df1_filtered = df1.filter(items=['字段1', '字段2'])
df2_filtered = df2.filter(items=['字段3', '字段4'])
# 输出结果
print(df1_filtered)
print(df2_filtered)
相关问题
pandas批量对一个excel中的多个sheet做筛选字段操作
可以使用pandas库中的read_excel方法读取excel文件,然后使用pandas的筛选函数对每个sheet进行操作,最后将结果保存回excel文件中。以下是示例代码:
```python
import pandas as pd
# 读取excel文件
file_path = 'example.xlsx'
excel_file = pd.ExcelFile(file_path)
# 遍历每个sheet
for sheet_name in excel_file.sheet_names:
# 读取sheet数据
df = pd.read_excel(excel_file, sheet_name)
# 筛选字段操作
df = df[['列名1', '列名2', '列名3']]
# 保存结果到excel文件
with pd.ExcelWriter(file_path, mode='a') as writer:
df.to_excel(writer, sheet_name=sheet_name, index=False)
```
注意:需要安装pandas库才能运行以上代码。
pandas对同一excel中的多个表执行筛选并输出
可以使用 pandas 的 read_excel 函数读取 excel 文件,然后使用 pandas 的 filter 函数对多个表进行筛选,最后使用 pandas 的 to_excel 函数输出筛选后的结果到新的 excel 文件中。具体代码如下:
```python
import pandas as pd
# 读取 excel 文件
excel_file = pd.ExcelFile('your_excel_file.xlsx')
# 遍历 excel 文件中的每个表格
for sheet_name in excel_file.sheet_names:
# 读取表格数据
df = pd.read_excel(excel_file, sheet_name=sheet_name)
# 筛选数据
filtered_df = df.filter(['column1', 'column2', ...])
# 输出筛选后的结果到新的 excel 文件中
filtered_df.to_excel('filtered_' + sheet_name + '.xlsx', index=False)
```
其中,'column1', 'column2', ... 是需要筛选的列名,可以根据实际情况修改。
相关推荐
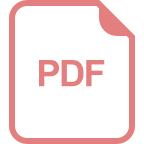
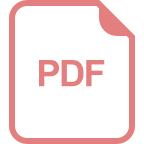












