实现朴素贝叶斯分类器(采用拉普拉斯修正)过滤垃圾邮件代码
时间: 2023-10-26 11:35:08 浏览: 83
好的,以下是一个简单的实现朴素贝叶斯分类器过滤垃圾邮件的 Python 代码(采用拉普拉斯修正):
```python
import os
import re
import math
# 定义垃圾邮件和正常邮件的路径
spam_path = 'spam/'
ham_path = 'ham/'
# 定义停用词列表
stop_words = ['a', 'an', 'the', 'in', 'on', 'at', 'to', 'of', 'for', 'by', 'with', 'from', 'and', 'or', 'not']
# 定义模型的参数
p_spam = 0.0
p_ham = 0.0
p_word_given_spam = {}
p_word_given_ham = {}
# 定义函数:获取文件内容
def get_file_content(file_path):
with open(file_path, 'r', encoding='utf-8', errors='ignore') as f:
content = f.read()
return content
# 定义函数:获取单词列表
def get_word_list(content):
# 去除标点符号和数字
content = re.sub(r'[^\w\s]|\d', ' ', content)
# 转为小写
content = content.lower()
# 分词
word_list = content.split()
# 去除停用词
word_list = [word for word in word_list if word not in stop_words]
return word_list
# 训练模型
def train():
global p_spam, p_ham, p_word_given_spam, p_word_given_ham
# 统计垃圾邮件和正常邮件的数量
spam_count = len(os.listdir(spam_path))
ham_count = len(os.listdir(ham_path))
# 计算先验概率
p_spam = spam_count / (spam_count + ham_count)
p_ham = ham_count / (spam_count + ham_count)
# 统计单词数量
word_count_given_spam = {}
word_count_given_ham = {}
for file_name in os.listdir(spam_path):
file_path = spam_path + file_name
content = get_file_content(file_path)
word_list = get_word_list(content)
for word in word_list:
word_count_given_spam[word] = word_count_given_spam.get(word, 0) + 1
for file_name in os.listdir(ham_path):
file_path = ham_path + file_name
content = get_file_content(file_path)
word_list = get_word_list(content)
for word in word_list:
word_count_given_ham[word] = word_count_given_ham.get(word, 0) + 1
# 计算条件概率
for word in word_count_given_spam.keys():
p_word_given_spam[word] = (word_count_given_spam[word] + 1) / (sum(word_count_given_spam.values()) + len(word_count_given_spam))
for word in word_count_given_ham.keys():
p_word_given_ham[word] = (word_count_given_ham[word] + 1) / (sum(word_count_given_ham.values()) + len(word_count_given_ham))
# 预测邮件类型
def predict(file_path):
content = get_file_content(file_path)
word_list = get_word_list(content)
# 初始化概率
p_spam_given_words = math.log(p_spam)
p_ham_given_words = math.log(p_ham)
# 计算条件概率的对数
for word in word_list:
if word in p_word_given_spam:
p_spam_given_words += math.log(p_word_given_spam[word])
else:
p_spam_given_words += math.log(1 / (sum(p_word_given_spam.values()) + len(p_word_given_spam)))
if word in p_word_given_ham:
p_ham_given_words += math.log(p_word_given_ham[word])
else:
p_ham_given_words += math.log(1 / (sum(p_word_given_ham.values()) + len(p_word_given_ham)))
# 判断邮件类型
if p_spam_given_words > p_ham_given_words:
return 'spam'
else:
return 'ham'
# 训练模型
train()
# 测试模型
result = predict('spam/1.txt')
print(result)
result = predict('ham/1.txt')
print(result)
```
其中,`spam/` 和 `ham/` 分别为存放垃圾邮件和正常邮件的文件夹路径,`stop_words` 是停用词列表,`train()` 函数用于训练模型,`predict(file_path)` 函数用于预测邮件类型。在训练模型时,采用了拉普拉斯修正,避免了概率为 0 的情况。在预测邮件类型时,采用了对数概率,防止概率连乘导致下溢。
阅读全文
相关推荐


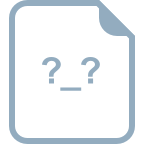
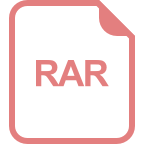
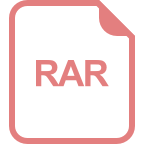
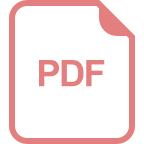
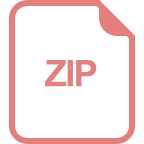
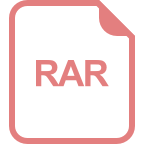
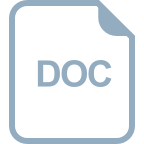
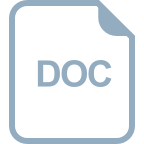
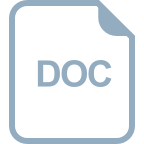
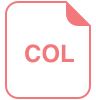



