function C.ApplyAnticipation(self, arg, ownerpos, tarPos) if self.mOwner.IsFighter and self.mOwner:IsFighter() then local aim = arg.target or ExtClip.GetLockAim(self.mOwner) local aimaim = ExtClip.GetLockAim(aim) local aimSpeed if aimaim then aimSpeed = aim:GetProperty(PropertyT.Move) else aimSpeed = aim:GetConfig().PatrolSpeed * 0.01 end if ExtClip.GetClip(aim) == ExtClip.Clip.run then if self.mCfg.ChangeSpeed then local Dis = DISTANCExz_V3(ownerpos, aim:GetPosition()) local speed = Dis --暂存 local time = 0 local timeList = self.mCfg.ChangeSpeed.Time local speedList = self.mCfg.ChangeSpeed.Speed local dis for i = 1, #timeList do if i == 1 then dis = timeList[i] * speedList[i] else dis = (timeList[i] - timeList[i - 1]) * (speedList[i] + speedList[i - 1]) end if dis >= Dis then if i == 1 then time = time + Dis / speedList[i] else time = time + Dis / ((speedList[i] + speedList[i - 1]) / 2) end Dis = 0 break else Dis = Dis - dis time = timeList[i] end end if Dis > 0 then time = time + Dis / speedList[#speedList] end speed = speed / time self.mFinalPos = ExtUtils.GetPredictPos(ownerpos, aim:GetPosition(), aim:GetForward():Clone():NormalizeSelf(), aimSpeed, speed) else self.mFinalPos = ExtUtils.GetPredictPos(ownerpos, aim:GetPosition(), aim:GetForward():Clone():NormalizeSelf(), aimSpeed, self:GetSpeed()) end if arg.target == nil then --非弹射 self.mOwner:LookAt(self.mFinalPos, true) end self.mFinalPos.y = self.mFinalPos.y + aim:GetHurtDummyPos().y self.mDir = self.mFinalPos:Sub(self.mPos):NormalizeSelf() end end 注释
时间: 2024-02-10 22:34:36 浏览: 179
这是一段Lua代码,用于实现一个角色在攻击时预测目标移动的位置,从而能够命中目标。首先会获取目标的移动速度,然后根据设定的速度变化规则来计算目标预计到达的位置。如果目标正在跑步,则会根据速度变化规则来计算预计的速度,否则直接使用目标的巡逻速度。最后将预测的位置调整到目标的伤害点,并计算出角色的朝向。如果攻击不是弹射攻击,则角色会朝着预测的位置看去。
相关问题
运行class GuidedBackpropReLUModel: def __init__(self, model, use_cuda): self.model = model self.model.eval() self.cuda = use_cuda if self.cuda: self.model = model.cuda() for idx, module in self.model.features._modules.items(): if module.__class__.__name__ == 'ReLU': self.model.features._modules[idx] = GuidedBackpropReLU() def forward(self, input): return self.model(input) def forward_static(self, input): if self.cuda: output = self.forward(input.cuda()) else: output = self.forward(input) return output def __call__(self, input, index = None): output = self.forward_static(input) if index == None: index = np.argmax(output.cpu().data.numpy()) one_hot = np.zeros((1, output.size()[-1]), dtype = np.float32) one_hot[0][index] = 1 one_hot = Variable(torch.from_numpy(one_hot), requires_grad = True) if self.cuda: one_hot = torch.sum(one_hot.cuda() * output) else: one_hot = torch.sum(one_hot * output) one_hot.backward() output = input.grad.cpu().data.numpy() output = output[0,:,:,:] return output报错Legacy autograd function with non-static forward method is deprecated. Please use new-style autograd function with static forward method. 如何修改代码
这个错误提示是因为代码中使用了旧版本的autograd函数,需要使用新版本的autograd函数。可以使用`@staticmethod`修饰`GuidedBackpropReLU`类中的`forward`方法,将其转换为静态方法。修改后的代码如下:
```
class GuidedBackpropReLU(nn.ReLU):
@staticmethod
def forward(input):
output = torch.clamp(input, min=0.0)
grad = torch.zeros_like(output)
grad[output > 0] = 1.0
output = output * grad
return output
class GuidedBackpropReLUModel:
def __init__(self, model, use_cuda):
self.model = model
self.model.eval()
self.cuda = use_cuda
if self.cuda:
self.model = model.cuda()
for idx, module in self.model.features._modules.items():
if module.__class__.__name__ == 'ReLU':
self.model.features._modules[idx] = GuidedBackpropReLU()
def forward(self, input):
return self.model(input)
def forward_static(self, input):
if self.cuda:
output = self.forward(input.cuda())
else:
output = self.forward(input)
return output
def __call__(self, input, index=None):
output = self.forward_static(input)
if index is None:
index = np.argmax(output.cpu().data.numpy())
one_hot = np.zeros((1, output.size()[-1]), dtype=np.float32)
one_hot[0][index] = 1
one_hot = Variable(torch.from_numpy(one_hot), requires_grad=True)
if self.cuda:
one_hot = torch.sum(one_hot.cuda() * output)
else:
one_hot = torch.sum(one_hot * output)
one_hot.backward()
output = input.grad.cpu().data.numpy()
output = output[0, :, :, :]
return output
```
class GuidedBackpropReLUModel: def init(self, model, use_cuda): self.model = model self.model.eval() self.cuda = use_cuda if self.cuda: self.model = model.cuda() for idx, module in self.model.features._modules.items(): if module.class.name == 'ReLU': self.model.features._modules[idx] = GuidedBackpropReLU() def forward(self, input): model1 = nn.Sequential(*list(self.model.children())[1:]) return model1(input) def forward_static(self, input): if self.cuda: output = self.forward(input.cuda()) else: output = self.forward(input) return output def call(self, input, index=None): output = self.forward_static(input) if index is None: index = np.argmax(output.cpu().data.numpy()) one_hot = np.zeros((1, output.size()[-1]), dtype=np.float32) one_hot[0][index] = 1 one_hot = Variable(torch.from_numpy(one_hot), requires_grad=True) if self.cuda: one_hot = torch.sum(one_hot.cuda() * output) else: one_hot = torch.sum(one_hot * output) one_hot.backward() output = input.grad.cpu().data.numpy() output = output[0, :, :, :] return output 代码中input.gard为空怎么解决,代码如何修改
你的代码中有一个小错误,`__init__` 方法的名称应该是 `__init__` 而不是 `init`。
以下是修改后的代码,其中通过调用 `input.requires_grad_(True)` 将输入的 `input` 设置为需要计算梯度,从而解决了 `input.grad` 为空的问题:
```
class GuidedBackpropReLUModel:
def __init__(self, model, use_cuda):
self.model = model
self.model.eval()
self.cuda = use_cuda
if self.cuda:
self.model = model.cuda()
for idx, module in self.model.features._modules.items():
if module.__class__.__name__ == 'ReLU':
self.model.features._modules[idx] = GuidedBackpropReLU()
def forward(self, input):
model1 = nn.Sequential(*list(self.model.children())[1:])
return model1(input)
def forward_static(self, input):
if self.cuda:
output = self.forward(input.cuda())
else:
output = self.forward(input)
return output
def __call__(self, input, index=None):
input.requires_grad_(True) # 将输入设置为需要计算梯度
output = self.forward_static(input)
if index is None:
index = np.argmax(output.cpu().data.numpy())
one_hot = np.zeros((1, output.size()[-1]), dtype=np.float32)
one_hot[0][index] = 1
one_hot = Variable(torch.from_numpy(one_hot), requires_grad=True)
if self.cuda:
one_hot = torch.sum(one_hot.cuda() * output)
else:
one_hot = torch.sum(one_hot * output)
one_hot.backward()
output = input.grad.cpu().data.numpy()
output = output[0, :, :, :]
return output
```
阅读全文
相关推荐
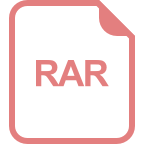
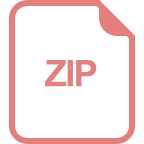
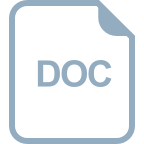



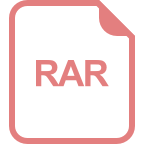
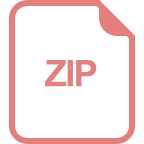
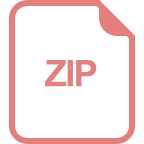
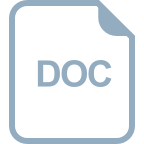





