network_input = [] network_output = [] for i in range(0, len(notes) - sequence_length, 1): # 循环次数,步长为1 sequence_in = notes[i:i + sequence_length] # 每次输入100个序列,每隔长度1取下一组,例如:(0,100),(1,101),(50,150) sequence_out = notes[i + sequence_length] # 真实值,从100开始往后 network_input.append([pitch_to_int[char] for char in sequence_in]) # 列表生成式 # 把sequence_in中的每个字符转为整数(pitch_to_int[char])放到network_input network_output.append(pitch_to_int[sequence_out]) # 把sequence_out的一个字符转为整数 n_patterns = len(network_input) # 输入序列长度 # 将输入序列的形状转成神经网络模型可以接受的 network_input = np.reshape(network_input, (n_patterns, sequence_length, 1)) # 输入,要改成的形状 # 将输入标准化,归一化 network_input = network_input / float(num_pitch) # 将期望输出转换成{0,1}布尔矩阵,配合categorical_crossentrogy误差算法的使用 network_output = tf.keras.utils.to_categorical(network_output) # keras中的这个方法可以将一个向量传进去转成布尔矩阵,供交叉熵的计算 return network_input, network_output这个代码里有时间步的概念吗
时间: 2024-03-29 11:38:58 浏览: 18
这段代码中确实有时间步的概念。在这段代码中,输入的音符序列被分成多个序列,每个序列包含一个固定长度的音符集合,这个长度就是序列的长度,也就是代码中的sequence_length。然后,每个序列都作为模型的一个时间步输入。在循环中,每次取一个长度为sequence_length的序列作为输入,然后在这个序列后面取一个音符作为输出,这个音符实际上是下一个时间步的输入。
因此,这段代码分割了原始音符序列成为多个长度为sequence_length的序列,将每个序列转换为整数,并将所有的序列作为模型的多个时间步输入。在reshape操作中,第二个维度被设置为sequence_length,表示每个时间步的输入包含一个长度为sequence_length的音符序列。因此,这个代码中的sequence_length就是时间步的概念。
相关问题
def prepare_sequences(notes, n_vocab): sequence_length = 100 pitchnames = sorted(set(notes)) note_to_int = dict((note, number) for number, note in enumerate(pitchnames)) network_input = [] network_output = [] # 创建输入序列和输出序列 for i in range(0, len(notes) - sequence_length, 1): sequence_in = notes[i:i + sequence_length] sequence_out = notes[i + sequence_length] network_input.append([note_to_int[char] for char in sequence_in]) network_output.append(note_to_int[sequence_out]) n_patterns = len(network_input) # 将输入序列转换为 LSTM 的三维格式 network_input = np.reshape(network_input, (n_patterns, sequence_length, 1)) # 将输出序列转换为 one-hot 编码 network_output = np_utils.to_categorical(network_output) return (network_input, network_output)的意思
这段代码是用于将音符序列转换为神经网络的输入和输出格式的函数。具体实现步骤如下:
- 确定序列长度,这里是100;
- 找到所有不同的音符,并将它们按字典序排序;
- 创建一个将音符映射为数字的字典;
- 创建两个空列表,分别用于存储输入序列和输出序列;
- 遍历整个音符序列,每次取出长度为sequence_length的一段作为输入,以及紧接着这段音符的下一个音符作为输出。将输入序列和输出序列转换为数字序列,并分别加入到之前创建的两个列表中;
- 将输入序列转换为LSTM模型需要的三维格式,即 (样本数, 时间步长, 特征数),这里的特征数是1;
- 将输出序列转换为one-hot编码,以便于神经网络的训练;
- 返回格式化后的输入序列和输出序列。
def prepare_sequences(notes, n_vocab): sequence_length = 100 pitchnames = sorted(set(item for item in notes)) # 将notes元素去重,按照字母顺序排列 note_to_int = dict((note, number) for number, note in enumerate(pitchnames)) # 创建一个字典将音符映射到整数 network_input = [] network_output = [] for i in range(0, len(notes) - sequence_length, 1): sequence_in = notes[i:i + sequence_length] # 输入序列 sequence_out = notes[i + sequence_length] # 输出序列 network_input.append([note_to_int[char] for char in sequence_in]) network_output.append(note_to_int[sequence_out]) # 将输入序列和输出序列转化为整数放入network_列表中 n_patterns = len(network_input) # 获取神经网络的输入数据中有多少个序列 network_input = np.reshape(network_input, (n_patterns, sequence_length, 1)) network_input = network_input / float(n_vocab) # 将网络输入中的每个元素都除以字典中不同字符的数量(即字典大小),以便将它们缩放到0到1之间的范围 network_output = np_utils.to_categorical(network_output) # 将网络输出向量进行one-hot编码,将每个离散的字符都转化为一个向量,以便将其作为神经网络的输出 return (network_input, network_output)
这是一个Python函数,用于将音符序列转换为神经网络的输入和输出序列。该函数接收两个参数:notes和n_vocab。notes是一个包含音符序列的列表,n_vocab是音符字典中不同字符的数量。该函数首先定义了一个序列长度sequence_length,然后使用sorted()函数将notes列表中的元素去除重复后按字母顺序排列,并将排列后的结果保存在pitchnames变量中。接着,该函数创建了一个note_to_int字典,将每个音符映射到一个整数。然后,该函数使用一个for循环将notes序列分割为输入序列和输出序列,并将它们转换为整数,并将它们保存到network_input和network_output两个列表中。最后,该函数使用np.reshape()函数将network_input列表转换为一个numpy数组,并将其除以n_vocab以将其缩放到0到1之间的范围。该函数还使用np_utils.to_categorical()函数将network_output列表转换为one-hot编码的向量,并将其作为神经网络的输出。最后,该函数返回network_input和network_output列表。
相关推荐
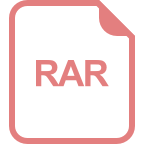
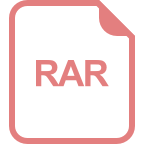







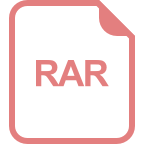
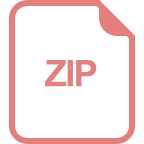
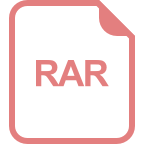
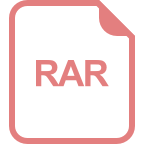
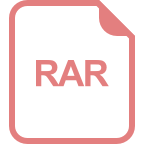