请举例使用python进行强化学习识别复杂网络重要节点方法
时间: 2023-09-06 14:10:41 浏览: 47
以下是使用 Python 进行基于 Q-learning 的强化学习识别复杂网络重要节点的示例代码:
```python
import numpy as np
import networkx as nx
# 构造一个简单的网络
G = nx.Graph()
G.add_edges_from([(1, 2), (1, 3), (2, 3), (2, 4), (3, 4)])
# 定义节点状态和动作
states = ["low", "medium", "high"]
actions = ["add", "delete"]
# 定义 Q-learning 模型
Q = np.zeros((len(G.nodes), len(states), len(actions)))
# 定义奖励函数
def reward(state, action):
if state == "high" and action == "delete":
return 1
elif state == "low" and action == "add":
return 1
else:
return -1
# 定义状态转移函数
def transition(state, action):
if state == "low" and action == "add":
return "medium"
elif state == "medium" and action == "add":
return "high"
elif state == "high" and action == "delete":
return "medium"
else:
return state
# 训练 Q-learning 模型
alpha = 0.1 # 学习率
gamma = 0.9 # 折扣因子
for i in range(1000):
state = np.random.choice(states)
node = np.random.choice(list(G.nodes))
s = states.index(state)
for j in range(10):
action = np.argmax(Q[node, s, :])
if np.random.rand() < 0.1:
action = np.random.choice(len(actions))
a = actions.index(actions[action])
next_state = transition(state, actions[action])
r = reward(state, actions[action])
next_s = states.index(next_state)
Q[node, s, a] += alpha * (r + gamma * np.max(Q[node, next_s, :]) - Q[node, s, a])
state = next_state
s = next_s
# 计算每个节点的 Q 值和重要性
q_vals = np.max(Q, axis=1)
importance = np.sum(q_vals, axis=1)
# 输出结果
print("节点重要性:", importance)
```
该示例代码中使用 Q-learning 模型训练节点的 Q 值,并根据 Q 值计算节点的重要性。在训练过程中,通过随机选择节点和状态,模拟节点动态变化的过程。最终得到每个节点的 Q 值和重要性。
需要注意的是,这只是一个简单的示例代码,实际应用中需要根据具体问题进行调整和优化。
相关推荐
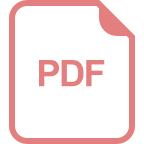
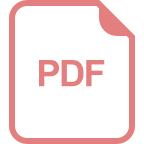
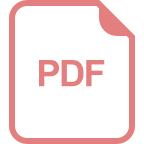














