X_train, X_test, y_train, y_test = train_test_split(data['x'].values.reshape(-1, 1), data['y'], test_size=0.2, random_state=0)
时间: 2024-06-16 21:08:42 浏览: 14
这段代码是用Python的机器学习库sklearn的train_test_split函数将数据集分为训练集和测试集的代码。其中:
* `X_train, X_test`:这些是分别代表训练集和测试集的特征数据。这里特征数据是通过将`data['x'].values.reshape(-1, 1)`(这可能是一个二维的numpy数组或者pandas DataFrame)转换为一维,并使用`train_test_split`函数分割得到的。
* `y_train, y_test`:这些是分别代表训练集和测试集的目标数据。这里目标数据是通过`data['y']`得到的。
* `test_size=0.2`:这个参数定义了测试集的大小,即20%的数据将被用于测试,80%的数据将被用于训练。
* `random_state=0`:这个参数是一个种子值,用于确保每次运行代码时,分割的数据集都是一样的。
这段代码通常在机器学习或数据科学中用于将原始数据集分为训练集和测试集,以便评估模型的性能。
相关问题
解释以下代码def split_data(x, y, ratio=0.8): to_train = int(input_len * ratio) # 进行调整以匹配 batch_size to_train -= to_train % batch_size x_train = x[:to_train] y_train = y[:to_train] x_test = x[to_train:] y_test = y[to_train:] # 进行调整以匹配 batch_size to_drop = x.shape[0] % batch_size if to_drop > 0: x_test = x_test[:-1 * to_drop] y_test = y_test[:-1 * to_drop] # 一些重塑 reshape_3 = lambda x: x.values.reshape((x.shape[0], x.shape[1], 1)) x_train = reshape_3(x_train) x_test = reshape_3(x_test) reshape_2 = lambda x: x.values.reshape((x.shape[0], 1)) y_train = reshape_2(y_train) y_test = reshape_2(y_test) return (x_train, y_train), (x_test, y_test) (x_train, y_train), (x_test, y_test) = split_data(data_input, expected_output) print('x_train.shape: ', x_train.shape) print('y_train.shape: ', y_train.shape) print('x_test.shape: ', x_test.shape) print('y_test.shape: ', y_test.shape)
这段代码是一个数据分割函数,用于将输入数据和输出数据按照一定比例分割成训练集和测试集。其中,参数 x 和 y 分别是输入数据和输出数据,ratio 表示训练集所占比例,默认为 0.8。
首先,函数根据 ratio 计算出训练集的长度 to_train,并将其调整为能够匹配 batch_size 的长度。然后,函数将输入数据和输出数据分别划分为训练集和测试集,其中测试集的长度为输入数据总长度减去训练集长度。同样地,函数也将测试集的长度调整为能够匹配 batch_size 的长度。
接下来,函数对训练集和测试集进行了一些重塑操作,以便于后续的模型训练。其中,reshape_3 函数将训练集和测试集的输入数据转化为三维张量,reshape_2 函数将训练集和测试集的输出数据转化为二维张量。
最后,函数返回了训练集和测试集的输入数据和输出数据,分别存储在 (x_train, y_train) 和 (x_test, y_test) 中,并输出了各自的形状。
如何用我的.csv文件替换下列代码中的数据集,其中我的.csv文件是一个列数加上四个变量的五列数据,代码如下 #code-4-3.py #Simple Linear Regression from sklearn.datasets import load_boston from sklearn.linear_model import LinearRegression import matplotlib.pyplot as plt from sklearn. model_selection import train_test_split dataset = load_boston() x_data = dataset.data # 导入所有特征变量 y_data = dataset.target # 导入目标值(房价) name_data = dataset.feature_names #导入特征 x_train,x_test,y_train,y_test = train_test_split(x_data, y_data,test_size= 0.25,random_state= 1001) x_data_train = x_train[:, 5].reshape(-1, 1)#选取前400个样本作为训练集 y_data_train = y_train.reshape(-1, 1) x_data_test = x_test[:, 5].reshape(-1, 1)#选取剩余的样本作为训练集 y_data_test = y_test.reshape(-1, 1) simple_model = LinearRegression() #创建线性回归估计器实例 simple_model.fit(x_data_train,y_data_train)#用训练数据拟合模型 y_data_test_p = simple_model.predict(x_data_test)#用训练的模型对测试集进行预测 plt.subplot(1, 1, 1) plt.scatter(x_data_test,y_data_test,s = 20, color="r") plt.scatter(x_data_test,y_data_test_p,s = 20, color="b") plt.xlabel('Room Number') plt.ylabel('Price') plt.title(name_data[5]) plt.show() r_squared = simple_model.score(x_data_test, y_data_test) print('R2') print(r_squared)
可以使用pandas库中的read_csv()函数读取csv文件,并将读取的数据集存储在一个DataFrame对象中,然后将该DataFrame对象转换为NumPy数组,以便用于模型训练。
下面是替换后的代码示例:
``` python
#code-4-3.py #Simple Linear Regression
import pandas as pd
import numpy as np
from sklearn.linear_model import LinearRegression
import matplotlib.pyplot as plt
from sklearn.model_selection import train_test_split
# 读取csv文件
data = pd.read_csv('your_dataset.csv')
# 获取特征变量和目标值
x_data = data.iloc[:, :-4].values
y_data = data.iloc[:, -4:].values
# 选取某一特征变量作为训练集和测试集
x_train,x_test,y_train,y_test = train_test_split(x_data, y_data, test_size=0.25, random_state=1001)
x_data_train = x_train[:, 5].reshape(-1, 1)
y_data_train = y_train.reshape(-1, 1)
x_data_test = x_test[:, 5].reshape(-1, 1)
y_data_test = y_test.reshape(-1, 1)
simple_model = LinearRegression()
simple_model.fit(x_data_train, y_data_train)
y_data_test_p = simple_model.predict(x_data_test)
plt.subplot(1, 1, 1)
plt.scatter(x_data_test, y_data_test, s=20, color="r")
plt.scatter(x_data_test, y_data_test_p, s=20, color="b")
plt.xlabel('Room Number')
plt.ylabel('Price')
plt.title('your_feature_name')
plt.show()
r_squared = simple_model.score(x_data_test, y_data_test)
print('R2')
print(r_squared)
```
需要注意的是,你需要将代码中的“your_dataset.csv”和“your_feature_name”替换为你实际使用的文件名和特征名称。
相关推荐
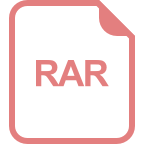
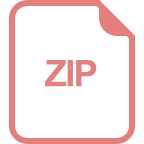
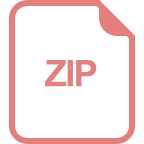












