如何在跑代码的时候用GPU并给出一段大致代码
时间: 2024-09-19 12:01:59 浏览: 54
在Python中,特别是深度学习和科学计算领域,利用GPU加速通常是通过使用NumPy、Pandas等库配合像TensorFlow、PyTorch这样的框架来实现的。如果你需要直接使用GPU资源,首先你需要安装支持GPU的版本,如anaconda的`tensorflow-gpu`或`pytorch torchvision cudatoolkit`。
以下是一个简单的例子,使用PyTorch:
```python
# 首先确保已经安装了torch和cuda
import torch
# 检查设备是否可用
device = torch.device("cuda" if torch.cuda.is_available() else "cpu")
print(f"Using device: {device}")
# 创建张量并在GPU上运行
x = torch.randn(5000, 5000).to(device)
y = x * 2 # 这里乘法运算会自动在GPU上进行
# 看看GPU占用情况
torch.cuda.memory_allocated()
```
在这个例子中,`torch.randn()`函数创建了一个随机张量,并通过`.to(device)`将其移动到GPU上。如果GPU不可用,程序会自动切换到CPU。
相关问题
给出利用对话数据集训练一个基于深度学习的智能问答系统,并能在前端进行交互的代码
这是一个相对复杂的项目,需要一定的深度学习和编程技能。我可以提供一个大致的步骤,但具体实现需要根据数据集和所选技术进行调整。
步骤一:数据预处理
1. 下载对话数据集(如Cornell Movie Dialogs Corpus或Ubuntu Dialog Corpus),并解析成一组对话对(question, answer)的形式。
2. 对原始数据进行清洗、分词、去停用词、词干提取等处理,以便于后续建模。
步骤二:建立模型
1. 选择合适的深度学习模型,如循环神经网络(RNN)、长短时记忆网络(LSTM)或变压器(Transformer)等。
2. 根据数据集的大小和特点,确定模型的超参数(如层数、隐藏单元数、学习率等)。
3. 将数据集分为训练集、验证集和测试集,并进行批量处理。
步骤三:训练模型
1. 将预处理后的数据集输入到模型中进行训练,使用损失函数(如交叉熵损失)和优化器(如Adam)对模型进行优化。
2. 定期保存模型权重,并在验证集上进行评估,以便调整模型参数和超参数。
3. 训练模型的时间和资源取决于模型的复杂性和数据集的大小,可能需要使用GPU或分布式计算。
步骤四:前端交互
1. 在前端设计交互界面,例如一个简单的聊天窗口。
2. 将模型部署到服务器上,以便与前端进行交互。
3. 使用Web框架(如Flask或Django)创建API,使得前端可以发送查询请求并接收模型的响应。
4. 在API中添加逻辑,以便在接收查询后,将输入传递给模型进行推理,并将输出返回给前端展示。
下面是一个基于Python的代码示例,以使用Cornell Movie Dialogs Corpus数据集和LSTM模型为例。
1. 数据预处理
```python
import re
import os
import pickle
import numpy as np
def load_conversations():
with open('data/movie_conversations.txt', 'r', encoding='iso-8859-1') as f:
conversations = f.readlines()
return [line.split(' +++$+++ ')[-1][1:-2].replace("'", "").replace(",","") for line in conversations]
def load_lines():
with open('data/movie_lines.txt', 'r', encoding='iso-8859-1') as f:
lines = f.readlines()
return {line.split(' +++$+++ ')[0]: line.split(' +++$+++ ')[-1][:-1] for line in lines}
def clean_text(text):
text = text.lower()
text = re.sub(r"i'm", "i am", text)
text = re.sub(r"he's", "he is", text)
text = re.sub(r"she's", "she is", text)
text = re.sub(r"it's", "it is", text)
text = re.sub(r"that's", "that is", text)
text = re.sub(r"what's", "what is", text)
text = re.sub(r"where's", "where is", text)
text = re.sub(r"\'ll", " will", text)
text = re.sub(r"\'ve", " have", text)
text = re.sub(r"\'re", " are", text)
text = re.sub(r"\'d", " would", text)
text = re.sub(r"won't", "will not", text)
text = re.sub(r"can't", "cannot", text)
text = re.sub(r"n't", " not", text)
text = re.sub(r"\W+", " ", text)
text = text.strip()
return text
def load_dataset():
conversations = load_conversations()
lines = load_lines()
inputs = []
outputs = []
for i in range(0, len(conversations), 2):
input_line = clean_text(lines[conversations[i]])
output_line = clean_text(lines[conversations[i+1]])
inputs.append(input_line)
outputs.append(output_line)
return inputs, outputs
def build_vocab(inputs, outputs):
vocab = set()
for sentence in inputs + outputs:
words = sentence.split()
for word in words:
vocab.add(word)
return sorted(vocab)
def save_dataset(inputs, outputs, vocab):
if not os.path.exists('data/processed'):
os.makedirs('data/processed')
with open('data/processed/inputs.pkl', 'wb') as f:
pickle.dump(inputs, f)
with open('data/processed/outputs.pkl', 'wb') as f:
pickle.dump(outputs, f)
with open('data/processed/vocab.pkl', 'wb') as f:
pickle.dump(vocab, f)
inputs, outputs = load_dataset()
vocab = build_vocab(inputs, outputs)
save_dataset(inputs, outputs, vocab)
```
2. 建立模型
```python
import tensorflow as tf
from tensorflow.keras.layers import Embedding, LSTM, Dense, Bidirectional
from tensorflow.keras.models import Sequential
def build_model(vocab_size, embedding_dim, hidden_dim):
model = Sequential([
Embedding(vocab_size, embedding_dim),
Bidirectional(LSTM(hidden_dim)),
Dense(vocab_size, activation='softmax')
])
model.compile(loss='categorical_crossentropy', optimizer='adam', metrics=['accuracy'])
return model
```
3. 训练模型
```python
import pickle
from tensorflow.keras.preprocessing.text import Tokenizer
from tensorflow.keras.preprocessing.sequence import pad_sequences
from tensorflow.keras.utils import to_categorical
def load_dataset():
with open('data/processed/inputs.pkl', 'rb') as f:
inputs = pickle.load(f)
with open('data/processed/outputs.pkl', 'rb') as f:
outputs = pickle.load(f)
with open('data/processed/vocab.pkl', 'rb') as f:
vocab = pickle.load(f)
return inputs, outputs, vocab
def preprocess_data(inputs, outputs, vocab, maxlen):
tokenizer = Tokenizer(num_words=len(vocab))
tokenizer.fit_on_texts(vocab)
input_seqs = tokenizer.texts_to_sequences(inputs)
output_seqs = tokenizer.texts_to_sequences(outputs)
input_seqs = pad_sequences(input_seqs, maxlen=maxlen, padding='post')
output_seqs = pad_sequences(output_seqs, maxlen=maxlen, padding='post')
output_seqs = to_categorical(output_seqs, num_classes=len(vocab))
return input_seqs, output_seqs, tokenizer
def train_model(model, inputs, outputs, epochs, batch_size):
history = model.fit(inputs, outputs, epochs=epochs, batch_size=batch_size, validation_split=0.2)
return history
MAXLEN = 50
VOCAB_SIZE = 10000
EMBEDDING_DIM = 128
HIDDEN_DIM = 256
BATCH_SIZE = 64
EPOCHS = 10
inputs, outputs, vocab = load_dataset()
input_seqs, output_seqs, tokenizer = preprocess_data(inputs, outputs, vocab, MAXLEN)
model = build_model(VOCAB_SIZE, EMBEDDING_DIM, HIDDEN_DIM)
history = train_model(model, input_seqs, output_seqs, EPOCHS, BATCH_SIZE)
model.save('model.h5')
```
4. 前端交互
```python
import tensorflow as tf
import pickle
from tensorflow.keras.preprocessing.text import Tokenizer
from tensorflow.keras.preprocessing.sequence import pad_sequences
MAXLEN = 50
VOCAB_SIZE = 10000
def load_model():
return tf.keras.models.load_model('model.h5')
def load_tokenizer():
with open('data/processed/vocab.pkl', 'rb') as f:
vocab = pickle.load(f)
tokenizer = Tokenizer(num_words=len(vocab))
tokenizer.fit_on_texts(vocab)
return tokenizer
def preprocess_query(query, tokenizer):
query = [query]
query = tokenizer.texts_to_sequences(query)
query = pad_sequences(query, maxlen=MAXLEN, padding='post')
return query
def generate_response(query, model, tokenizer):
query = preprocess_query(query, tokenizer)
prediction = model.predict(query)[0]
predicted_word_idx = tf.argmax(prediction).numpy()
return tokenizer.index_word[predicted_word_idx]
model = load_model()
tokenizer = load_tokenizer()
while True:
query = input('You: ')
response = generate_response(query, model, tokenizer)
print('Bot:', response)
```
这段代码使用了一个简单的命令行界面,可以从控制台输入问题并获得模型的响应。要在Web应用程序中使用该模型,可以使用Flask或Django等框架来构建API,并使用JavaScript和HTML构建交互界面。
阅读全文
相关推荐
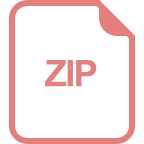
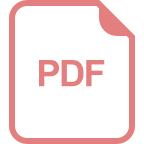
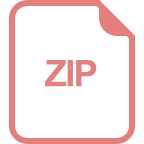
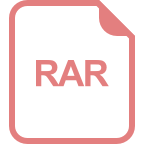
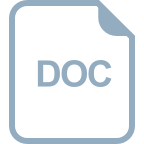
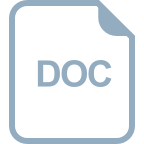
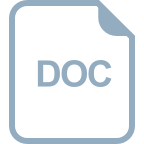
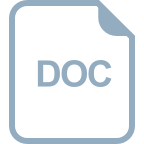
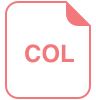
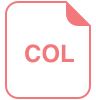
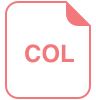
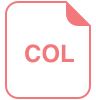
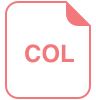
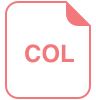
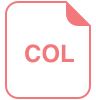
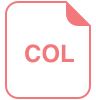
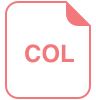