def set_grid(self, input_size): self.input_size = input_size self.grid_cell = self.create_grid(input_size) self.scale = np.array([[[input_size[1], input_size[0], input_size[1], input_size[0]]]]) self.scale_torch = torch.tensor(self.scale.copy(), device=self.device).float()
时间: 2024-04-18 22:26:35 浏览: 23
这段代码是一个方法,用于设置网格相关的参数。首先,将输入尺寸`input_size`保存到实例变量`self.input_size`中。然后调用`create_grid`方法生成网格矩阵,并将其保存到实例变量`self.grid_cell`中。接下来,创建一个形状为`[1, 1, 4]`的NumPy数组`scale`,其中包含了输入尺寸的四个坐标值`[input_size[1], input_size[0], input_size[1], input_size[0]]`。将该数组复制给变量`self.scale`。
最后,将`scale`数组复制一份,并将其转换为浮点型张量`scale_torch`,并将其移动到指定的设备上,并保存到实例变量`self.scale_torch`中。这个张量的形状为`[1, 1, 4]`,表示了输入尺寸的四个坐标值。
相关问题
请在这个DeepCFD的网络添加attention机制,并给出示例代码:import paddle import paddle.nn as nn class Attention(nn.Layer): def __init__(self, input_size, hidden_size): super(Attention, self).__init__() self.input_size = input_size self.hidden_size = hidden_size self.attn = nn.Linear(self.input_size + self.hidden_size, 1) self.softmax = nn.Softmax(axis=1) def forward(self, input, hidden): max_len = input.shape[0] attn_energies = paddle.zeros([max_len, 1]) for i in range(max_len): attn_energies[i] = self.score(input[i], hidden) attn_weights = self.softmax(attn_energies) context = paddle.sum(attn_weights * input, axis=0) return context.unsqueeze(0) def score(self, input, hidden): energy = self.attn(paddle.concat([input, hidden], axis=1)) return energy class DeepCFD(nn.Layer): def __init__(self, input_size, hidden_size, output_size): super(DeepCFD, self).__init__() self.input_size = input_size self.hidden_size = hidden_size self.output_size = output_size self.lstm = nn.LSTM(input_size, hidden_size, num_layers=2, batch_first=True) self.attention = Attention(input_size, hidden_size) self.fc = nn.Linear(hidden_size, output_size) def forward(self, input): output, (hidden, cell) = self.lstm(input) context = self.attention(output, hidden[-1]) output = self.fc(context) return output
好的,下面是添加了Attention机制的DeepCFD网络的示例代码:
import paddle
import paddle.nn as nn
class Attention(nn.Layer):
def __init__(self, input_size, hidden_size):
super(Attention, self).__init__()
self.input_size = input_size
self.hidden_size = hidden_size
self.attn = nn.Linear(self.input_size + self.hidden_size, 1)
self.softmax = nn.Softmax(axis=1)
def forward(self, input, hidden):
max_len = input.shape[1]
attn_energies = paddle.zeros([input.shape[0], max_len, 1])
for i in range(max_len):
attn_energies[:, i] = self.score(input[:, i, :], hidden)
attn_weights = self.softmax(attn_energies)
context = paddle.sum(attn_weights * input, axis=1)
return context
def score(self, input, hidden):
energy = self.attn(paddle.concat([input, hidden], axis=1))
return energy
class DeepCFD(nn.Layer):
def __init__(self, input_size, hidden_size, output_size):
super(DeepCFD, self).__init__()
self.input_size = input_size
self.hidden_size = hidden_size
self.output_size = output_size
self.lstm = nn.LSTM(input_size, hidden_size, num_layers=2, batch_first=True)
self.attention = Attention(input_size, hidden_size)
self.fc = nn.Linear(hidden_size, output_size)
def forward(self, input):
output, (hidden, cell) = self.lstm(input)
context = self.attention(output, hidden[-1])
output = self.fc(context)
return output
在这个示例代码中,我们将Attention机制应用到了LSTM的输出上。在Attention中,我们计算了每个时间步的注意力能量,然后使用softmax函数计算注意力权重。然后,我们将这些权重与LSTM输出相乘并求和,得到上下文向量作为Attention机制的输出。
在DeepCFD中,我们使用了两层LSTM,然后将LSTM输出和最后一个时刻的隐藏状态作为Attention机制的输入。最后,我们将Attention机制的输出传递到一个全连接层中,得到最终的输出。
这段代码哪里错了 class my_BiGRU(torch.nn.Module): def __init__(self, input_size, hidden_size, num_layers, output_size, gru_dropout): super(my_BiGRU, self).__init__() self.input_size = input_size self.hidden_size = hidden_size self.num_layers = num_layers self.output_size = output_size self.gru_dropout = gru_dropout self.gru = torch.nn.GRU(input_size, hidden_size, num_layers=num_layers, batch_first=True, bidirectional=True) self.fc = torch.nn.Linear(hidden_size * 2, output_size) def _setup_BiGRU_layers(self): self.BiGRU_layers = my_BiGRU(input_size=self.args.capsule_out_dim, output_size=self.args.apsule_out_features, hidden_size=self.args.BiGRU_hiddensize, num_layers=self.args.BiGRU_layers, gru_dropout=self.args.BiGRU_dropout, )
这段代码存在两个问题:
1.函数 `_setup_BiGRU_layers` 中的代码应该是在类的构造函数 `__init__` 中进行初始化,而不是在另外一个函数中进行初始化。
2.在调用 `my_BiGRU` 类时,参数名称有误,应该将 `apsule_out_features` 改为 `capsule_out_features`。
改正后的代码如下所示:
```
class my_BiGRU(torch.nn.Module):
def __init__(self, input_size, hidden_size, num_layers, output_size, gru_dropout, capsule_out_features):
super(my_BiGRU, self).__init__()
self.input_size = input_size
self.hidden_size = hidden_size
self.num_layers = num_layers
self.output_size = output_size
self.gru_dropout = gru_dropout
self.gru = torch.nn.GRU(input_size, hidden_size, num_layers=num_layers, batch_first=True, bidirectional=True)
self.fc = torch.nn.Linear(hidden_size * 2, output_size)
self.BiGRU_layers = my_BiGRU(input_size=self.input_size,
output_size=capsule_out_features,
hidden_size=self.hidden_size,
num_layers=self.num_layers,
gru_dropout=self.gru_dropout,
)
```
注意:这里假设 `capsule_out_dim` 和 `args` 都已经在代码中被定义好了。
相关推荐
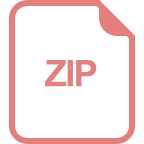
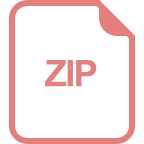
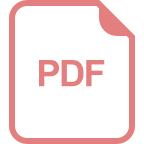













