将深度收缩残差网络中的去噪自适应调节阈值模块和残差连接用于卷积自编码器去噪
时间: 2024-04-18 12:33:39 浏览: 180
下面是一个使用深度收缩残差网络中的去噪自适应调节阈值模块和残差连接的卷积自编码器去噪的示例代码:
```python
import tensorflow as tf
from tensorflow.keras.layers import Conv1D, Conv1DTranspose, Input, Lambda, GlobalAveragePooling1D, Dense, Reshape, Multiply, Add
from tensorflow.keras import backend as K
# 定义软阈值函数
def soft_threshold(x, threshold):
return K.sign(x) * K.maximum(K.abs(x) - threshold, 0.0)
# 定义SENet模块
def se_block(x):
squeeze = GlobalAveragePooling1D()(x)
excitation = Dense(K.int_shape(x)[-1] // 16, activation='relu')(squeeze)
excitation = Dense(K.int_shape(x)[-1], activation='sigmoid')(excitation)
excitation = Reshape((1, K.int_shape(x)[-1]))(excitation)
scale = Multiply()([x, excitation])
return scale
# 定义深度收缩残差网络中的去噪自适应调节阈值模块
def denoising_threshold_module(x):
threshold = Lambda(lambda x: K.mean(K.abs(x), axis=[1,2], keepdims=True))(x)
threshold = Lambda(lambda x: soft_threshold(x[0], x[1]))([x, threshold])
return threshold
# 定义带有深度收缩残差网络中的去噪自适应调节阈值模块和残差连接的卷积自编码器
def denoising_autoencoder(input_shape):
# 编码器
inputs = Input(shape=input_shape)
encoded = Conv1D(32, 3, activation='relu', padding='same')(inputs)
encoded = Conv1D(16, 3, activation='relu', padding='same')(encoded)
# 添加深度收缩残差网络中的去噪自适应调节阈值模块
thresholded_encoded = denoising_threshold_module(encoded)
# 添加残差连接
residual_encoded = Add()([encoded, thresholded_encoded])
# 解码器
decoded = Conv1DTranspose(16, 3, activation='relu', padding='same')(residual_encoded)
decoded = Conv1DTranspose(32, 3, activation='relu', padding='same')(decoded)
decoded = Conv1D(1, 3, activation='sigmoid', padding='same')(decoded)
# 构建自编码器模型
autoencoder = tf.keras.Model(inputs, decoded)
return autoencoder
# 创建卷积自编码器模型
input_shape = (256, 1) # 输入形状
model = denoising_autoencoder(input_shape)
model.summary()
```
在上述代码中,我们定义了一个带有深度收缩残差网络中的去噪自适应调节阈值模块和残差连接的卷积自编码器模型。在编码器部分的最后添加了一个去噪自适应调节阈值模块,该模块通过计算编码器输出的平均绝对值来动态调节软阈值函数的阈值。然后,我们使用去噪自适应调节阈值模块输出的编码器输出和原始编码器输出进行残差连接,以增强去噪效果。在解码器部分,我们保持与之前相同的结构。
最后,通过构建自编码器模型,并打印模型摘要,可以查看模型的结构和参数数量。请根据你的需求进行适当的修改和调整。
阅读全文
相关推荐
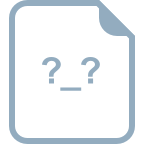

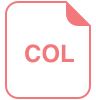
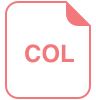
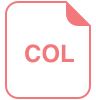
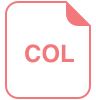
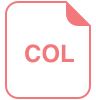
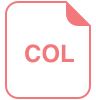
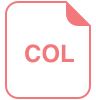
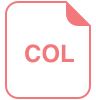
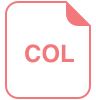
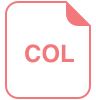
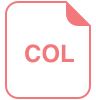
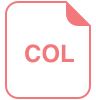
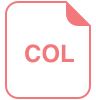
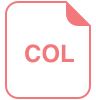
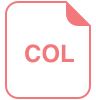