python线性回归算法定义
时间: 2023-11-07 21:58:25 浏览: 104
线性回归是一种用于建立自变量和因变量之间线性关系的统计模型。在Python中,可以使用sklearn库中的LinearRegression类来实现线性回归算法。首先,实例化LinearRegression类,然后使用fit()方法对模型进行拟合。通过coef_属性可以查看回归系数,intercept_属性可以查看截距。可以使用predict()方法对测试集进行预测,使用score()方法计算R2分数来评估模型的拟合程度。
相关问题
python线性回归算法代码
以下是Python实现一元线性回归的代码,包括假设函数、损失函数和梯度下降法:
```python
import numpy as np
# 定义假设函数
def hypothesis(theta, X):
return np.dot(X, theta)
# 定义损失函数
def cost_function(theta, X, y):
m = len(y)
J = np.sum((hypothesis(theta, X) - y) ** 2) / (2 * m)
return J
# 定义梯度下降函数
def gradient_descent(theta, X, y, alpha, num_iters):
m = len(y)
J_history = np.zeros(num_iters)
for i in range(num_iters):
theta = theta - alpha / m * np.dot(X.T, (hypothesis(theta, X) - y))
J_history[i] = cost_function(theta, X, y)
return theta, J_history
```
其中,假设函数hypothesis(theta, X)用于计算预测值,损失函数cost_function(theta, X, y)用于计算预测值与真实值之间的误差,梯度下降函数gradient_descent(theta, X, y, alpha, num_iters)用于更新参数theta,使得损失函数最小化。
下面是一个使用上述代码进行一元线性回归的例子:
```python
import matplotlib.pyplot as plt
# 构造数据
X = np.array([1, 2, 3, 4, 5])
y = np.array([3, 5, 7, 9, 11])
# 在X前面添加一列1,用于计算截距
X = np.vstack([np.ones(len(X)), X]).T
# 初始化参数
theta = np.zeros(2)
# 设置学习率和迭代次数
alpha = 0.01
num_iters = 1000
# 进行梯度下降
theta, J_history = gradient_descent(theta, X, y, alpha, num_iters)
# 输出最终的参数和损失函数值
print('theta:', theta)
print('cost:', cost_function(theta, X, y))
# 绘制数据和拟合直线
plt.scatter(X[:, 1], y)
plt.plot(X[:, 1], hypothesis(theta, X))
plt.show()
```
python线性回归_用Python实现线性回归算法
好的,关于使用 Python 实现线性回归算法,我可以给你一些基本的思路和代码实现。
首先,线性回归是一种用于建立两个变量之间线性关系的统计学方法。在机器学习中,我们通常使用线性回归来进行预测。下面是一个简单的例子。
假设我们有一个数据集,其中包含了房屋的面积和价格。我们想要通过这个数据集来预测房屋的价格。首先,我们需要将数据集导入到 Python 程序中,可以使用 Pandas 库来读取 CSV 文件。
```
import pandas as pd
data = pd.read_csv('data.csv')
```
接着,我们需要将数据集分成两个部分:训练集和测试集。训练集用于训练模型,测试集用于评估模型的性能。
```
from sklearn.model_selection import train_test_split
train_data, test_data = train_test_split(data, test_size=0.2)
```
然后,我们需要定义一个模型,用于预测房屋的价格。在这里,我们使用 scikit-learn 库提供的线性回归模型。
```
from sklearn.linear_model import LinearRegression
model = LinearRegression()
```
接着,我们需要将训练集的特征和标签分开,并用它们来训练模型。
```
X_train = train_data[['area']]
y_train = train_data[['price']]
model.fit(X_train, y_train)
```
最后,我们可以使用测试集来评估模型的性能。
```
X_test = test_data[['area']]
y_test = test_data[['price']]
score = model.score(X_test, y_test)
```
这里的 score 表示模型的拟合程度,越接近 1 表示模型的性能越好。
以上就是使用 Python 实现线性回归算法的基本思路和代码实现。当然,实际应用中还有很多细节需要注意,例如特征工程、数据清洗等。
阅读全文
相关推荐
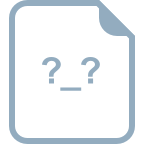
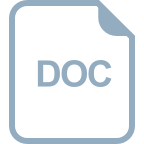
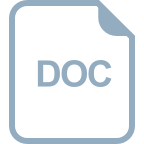

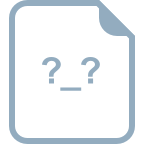
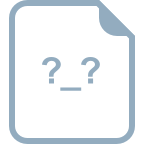
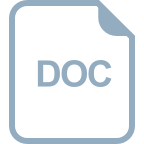






