def contrastive_evaluate(val_loader, model, memory_bank): top1 = AverageMeter('Acc@1', ':6.2f') model.eval() for batch in val_loader: images = batch['image'].cuda(non_blocking=True) target = batch['target'].cuda(non_blocking=True) constrastive_features, cluster_output = model(images) output = memory_bank.weighted_knn(constrastive_features) acc1 = 100*torch.mean(torch.eq(output, target).float()) top1.update(acc1.item(), images.size(0)) return top1.avg
时间: 2024-04-20 16:22:33 浏览: 198
这一个用于评估对比学习模型的函数contrastive_evaluate。它接受一个验证数据集val_loader,一个对比学习模型model,以及一个存储样本特征的内存库memory_bank。
首先,创建一个用于计算准确率的AverageMeter对象top1。然后将模型设置为评估模式,即model.eval()。
接下来,对于val_loader中的每个batch,获取图像数据images和目标标签target,并将它们移动到GPU上。
通过调用model(images)得到对比学习任务的特征向量constrastive_features和聚类任务的输出cluster_output。
然后,利用memory_bank中的样本特征进行加权K最近邻(weighted_knn)搜索,得到输出output。
计算准确率acc1为与目标标签target相等的元素所占的比例,并更新top1对象。
最后,返回top1.avg作为评估结果,即平均准确率。
相关问题
def contrastive_evaluate(val_loader, model, memory_bank): top1 = AverageMeter('Acc@1', ':6.2f') model.eval(),这段话是什么意思
这段代码是一个用于对模型进行对比学习评估的函数。函数的输入包括验证数据集的数据加载器`val_loader`、模型`model`和记忆库`memory_bank`。在函数内部,通过调用`model.eval()`将模型设置为评估模式。然后,使用`AverageMeter`类创建一个用于统计Top-1准确率的对象`top1`。函数的主要目的是计算模型在验证数据集上的Top-1准确率。
class ContrastiveModel(nn.Module): def __init__(self, backbone, head='mlp', features_dim=128): super(ContrastiveModel, self).__init__() self.backbone = backbone['backbone'] self.backbone_dim = backbone['dim'] self.head = head if head == 'linear': self.contrastive_head = nn.Linear(self.backbone_dim, features_dim) elif head == 'mlp': self.contrastive_head = nn.Sequential( nn.Linear(self.backbone_dim, self.backbone_dim), nn.ReLU(), nn.Linear(self.backbone_dim, features_dim)) else: raise ValueError('Invalid head {}'.format(head)) def forward(self, x): features = self.contrastive_head(self.backbone(x)) features = F.normalize(features, dim = 1) return features class ClusteringModel(nn.Module): def __init__(self, backbone, nclusters, nheads=1): super(ClusteringModel, self).__init__() self.backbone = backbone['backbone'] self.backbone_dim = backbone['dim'] self.nheads = nheads assert(isinstance(self.nheads, int)) assert(self.nheads > 0) self.cluster_head = nn.ModuleList([nn.Linear(self.backbone_dim, nclusters) for _ in range(self.nheads)]) def forward(self, x, forward_pass='default'): if forward_pass == 'default': features = self.backbone(x) out = [cluster_head(features) for cluster_head in self.cluster_head] elif forward_pass == 'backbone': out = self.backbone(x) elif forward_pass == 'head': out = [cluster_head(x) for cluster_head in self.cluster_head] elif forward_pass == 'return_all': features = self.backbone(x) out = {'features': features, 'output': [cluster_head(features) for cluster_head in self.cluster_head]} else: raise ValueError('Invalid forward pass {}'.format(forward_pass)) return out,这是什么模型啊
这个代码定义了两个模型:`ContrastiveModel` 和 `ClusteringModel`。
`ContrastiveModel` 是一个对比学习模型,用于训练图像特征。它接收一个 backbone 模型作为输入,该 backbone 模型提取输入图像的特征,然后通过一个 MLP 或 Linear 层将特征映射到一个固定维度的向量。最后,它对向量进行 L2 归一化,以获得最终的特征表示。该模型的作用是通过对比学习的方式,学习到图像的特征表示,以便用于其他任务,如分类、检索等。
`ClusteringModel` 是一个聚类模型,用于无监督图像分类。它接收一个 backbone 模型作为输入,该 backbone 模型提取输入图像的特征,然后通过一个线性层将特征映射到一个固定维度的向量,并将向量输入到多个聚类头(cluster_head)中。每个聚类头都是一个线性层,用于将特征向量映射到类别概率分布。该模型的作用是通过聚类的方式,将图像分为不同的类别,以便用于其他任务,如分类、检索等。
阅读全文
相关推荐
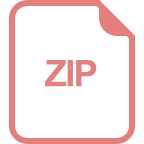
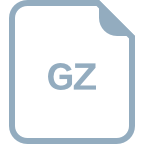
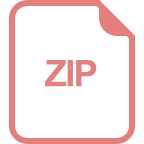



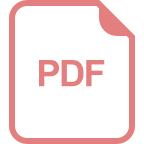
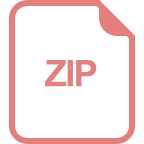
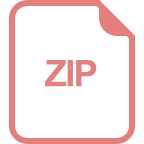
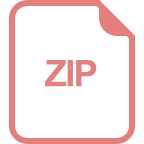




