result = ts.trainer.offpolicy_trainer( policy, train_collector, test_collector, max_epoch=max_epoch, step_per_epoch=step_per_epoch, collect_per_step=collect_per_step, episode_per_test=30, batch_size=64, train_fn=lambda e1, e2: policy.set_eps(0.1 / round), test_fn=lambda e1, e2: policy.set_eps(0.05 / round), writer=None)的collect_per_step是什么
时间: 2024-04-26 18:25:16 浏览: 31
collect_per_step是指在每个训练epoch中,agent从环境中采集数据的步数。具体来说,在每个epoch中,agent会执行step_per_epoch次训练步骤,每个训练步骤中,agent会从环境中连续采集collect_per_step个样本,这些样本将被用于训练agent的策略网络。因此,collect_per_step的值越大,agent每次采集的样本就越多,训练效率也就越高。但是,如果collect_per_step的值过大,可能会导致agent采集到的样本之间存在较大的相关性,从而影响训练效果。
相关问题
result = ts.trainer.offpolicy_trainer( policy, train_collector, test_collector, max_epoch=max_epoch, step_per_epoch=step_per_epoch, collect_per_step=collect_per_step, episode_per_test=30, batch_size=64, train_fn=lambda e1, e2: policy.set_eps(0.1 / round), test_fn=lambda e1, e2: policy.set_eps(0.05 / round), writer=None)
这段代码使用了 ts.trainer.offpolicy_trainer 训练器进行强化学习模型的训练,并将训练结果保存在 result 变量中。具体来说,这个训练器需要以下几个参数:
- policy:强化学习模型的策略网络,它将根据训练数据不断更新自己的参数,以提高在环境中的表现。
- train_collector:训练数据的采集器,它将负责从环境中收集训练数据,并将其保存到一个缓冲区中,供模型训练时使用。
- test_collector:测试数据的采集器,它将负责从环境中收集测试数据,用于评估模型在环境中的表现。
- max_epoch:最大训练轮数。
- step_per_epoch:每轮训练中的步数。
- collect_per_step:每步采集数据的次数。
- episode_per_test:每次测试中的回合数。
- batch_size:每次训练的样本数。
- train_fn:训练时的回调函数,用于设置模型的一些超参数。
- test_fn:测试时的回调函数,用于设置模型的一些超参数。
- writer:用于记录训练过程中的一些指标,如训练损失、测试得分等。
在训练过程中,训练器将根据训练数据对模型进行训练,并在每个 epoch 结束时使用测试数据对模型进行测试,最终返回训练结果 result。
ts.trainer.offpolicy_trainer
ts.trainer.offpolicy_trainer 是一个使用离线数据进行训练的强化学习训练器,它使用的算法是 Off-Policy Actor-Critic (OPAC)。它可以在离线数据集上进行训练,从而避免了在线学习时由于探索性行为导致的数据采集效率低下的问题。同时,它还可以使用多个离线数据集进行训练,从而增强模型的泛化能力。
相关推荐
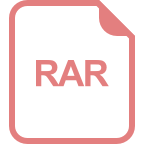
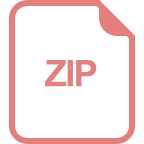











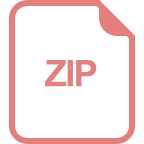
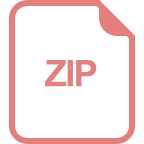