代码实现词向量模型
时间: 2023-08-03 13:18:55 浏览: 50
以下是一个基于Skip-gram模型实现的词向量代码示例:
```python
import numpy as np
import random
from collections import Counter
class SkipGram:
def __init__(self, corpus, embedding_size=100, window_size=2, min_count=5, num_negative_samples=5, learning_rate=0.01):
self.vocab = self.build_vocab(corpus, min_count)
self.word2idx = {w: i for i, w in enumerate(self.vocab)}
self.idx2word = {i: w for i, w in enumerate(self.vocab)}
self.embedding_size = embedding_size
self.window_size = window_size
self.min_count = min_count
self.num_negative_samples = num_negative_samples
self.learning_rate = learning_rate
self.W1 = np.random.rand(len(self.vocab), embedding_size)
self.W2 = np.random.rand(embedding_size, len(self.vocab))
def build_vocab(self, corpus, min_count):
word_counts = Counter(corpus)
vocab = [word for word, count in word_counts.items() if count >= min_count]
return vocab
def generate_training_data(self, corpus):
training_data = []
for i, word in enumerate(corpus):
word_index = self.word2idx[word]
context_indices = [self.word2idx[context] for context in corpus[max(i - self.window_size, 0): min(i + self.window_size, len(corpus))]]
for context_index in context_indices:
if context_index != word_index:
training_data.append((word_index, context_index))
return training_data
def sigmoid(self, x):
return 1 / (1 + np.exp(-x))
def train(self, corpus, epochs):
training_data = self.generate_training_data(corpus)
for epoch in range(epochs):
random.shuffle(training_data)
for word_index, context_index in training_data:
context_vector = self.W1[context_index]
z = np.dot(self.W2.T, context_vector)
sigmoid_z = self.sigmoid(z)
negative_samples = random.sample(self.vocab, self.num_negative_samples)
negative_indices = [self.word2idx[negative_sample] for negative_sample in negative_samples]
negative_vectors = self.W1[negative_indices]
negative_z = np.dot(negative_vectors, self.W2.T)
negative_sigmoid_z = self.sigmoid(-negative_z)
error = sigmoid_z - 1
negative_error = negative_sigmoid_z
self.W2[:, word_index] -= self.learning_rate * error * context_vector
self.W1[context_index] -= self.learning_rate * error * self.W2[:, word_index]
for negative_index, negative_vector in zip(negative_indices, negative_vectors):
self.W2[:, negative_index] -= self.learning_rate * negative_error * negative_vector
self.W1[negative_index] -= self.learning_rate * negative_error * self.W2[:, negative_index]
print(f"Epoch {epoch + 1}/{epochs} completed.")
def get_word_vector(self, word):
try:
word_index = self.word2idx[word]
except KeyError:
raise KeyError(f"'{word}' not in vocabulary")
return self.W1[word_index]
```
在上述代码中,我们定义了一个SkipGram类,它包含了以下几个主要方法:
- `build_vocab`:从语料中建立词汇表。
- `generate_training_data`:生成训练数据,即单词和上下文单词的对。
- `sigmoid`:sigmoid函数。
- `train`:训练模型。
- `get_word_vector`:获取单词的词向量。
我们可以使用以下代码进行训练和获取单词的词向量:
```python
corpus = ["i", "am", "a", "boy", "you", "are", "a", "girl"]
skip_gram = SkipGram(corpus)
skip_gram.train(corpus, epochs=100)
print(skip_gram.get_word_vector("boy"))
```
以上代码会输出“boy”这个单词的词向量。
相关推荐
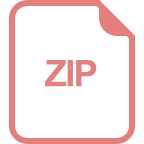
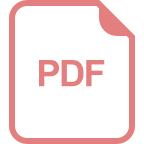
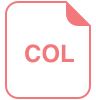
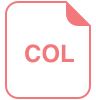
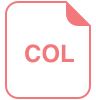
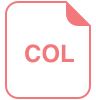
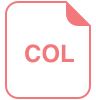









