将python中numpy矩阵转换成c++中opencv的矩阵
时间: 2023-12-03 22:03:14 浏览: 126
可以使用以下代码将numpy矩阵转换成OpenCV的矩阵:
```python
import cv2
import numpy as np
# 创建numpy矩阵
np_mat = np.array([[1, 2, 3], [4, 5, 6], [7, 8, 9]])
# 将numpy矩阵转换成OpenCV的矩阵
cv_mat = cv2.cv.fromarray(np_mat)
# 输出OpenCV矩阵
print(cv_mat)
```
在上述代码中,我们使用`cv2.cv.fromarray()`函数将numpy矩阵转换成OpenCV矩阵。注意,使用该函数时,需要确保numpy矩阵的数据类型与OpenCV矩阵的数据类型相同。
相关问题
python opencv实现C++ opencv中的convertTo
在Python的OpenCV库中,实现C++ OpenCV中的`convertTo`功能可以通过使用`cv2.convertScaleAbs`函数来完成,或者更常用的是通过直接进行数组操作来达到相同的目的。C++中的`convertTo`函数用于将图像矩阵转换成指定的数据类型,并且可以应用一个缩放因子和一个可选的偏移量。在Python中,可以使用NumPy库来对图像数组进行操作,实现类似的效果。
以下是一个Python中使用OpenCV和NumPy实现`convertTo`功能的例子:
```python
import cv2
import numpy as np
# 读取图像
image = cv2.imread('image.jpg', cv2.IMREAD_GRAYSCALE)
# 设置缩放因子和偏移量
alpha = 1.0 # 缩放因子,相当于C++中的scale
beta = 0 # 偏移量,相当于C++中的delta
# 应用convertTo功能
# 在Python中,可以使用NumPy的乘法和加法来模拟
# image.convertTo(image, dstType, alpha, beta)
result = image.astype(np.float32) * alpha + beta
# 将结果转换回原始图像的数据类型
if image.dtype == np.uint8:
result = np.clip(result, 0, 255)
result = result.astype(np.uint8)
else:
result = result.astype(image.dtype)
# 显示结果
cv2.imshow('Result', result)
cv2.waitKey(0)
cv2.destroyAllWindows()
```
请注意,对于不同数据类型的图像(比如uint8, float等),在进行操作后,可能需要进行类型转换和范围限制,以保证结果图像的像素值在合理的范围内。
能否将python代码转换成C++代码
是的,以下是一个基于OpenCV的C++三角测量示例代码:
```cpp
#include <opencv2/opencv.hpp>
using namespace cv;
int main()
{
// 读取图片
Mat img1 = imread("img1.jpg");
Mat img2 = imread("img2.jpg");
// 提取特征点和描述符
Ptr<SIFT> sift = SIFT::create();
std::vector<KeyPoint> kp1, kp2;
Mat des1, des2;
sift->detectAndCompute(img1, noArray(), kp1, des1);
sift->detectAndCompute(img2, noArray(), kp2, des2);
// 匹配特征点
BFMatcher bf(NORM_L2);
std::vector<std::vector<DMatch>> matches;
bf.knnMatch(des1, des2, matches, 2);
// 筛选匹配点
std::vector<DMatch> good;
for (size_t i = 0; i < matches.size(); i++)
{
if (matches[i][0].distance < 0.75 * matches[i][1].distance)
{
good.push_back(matches[i][0]);
}
}
// 获取匹配点在两个图像中的坐标
std::vector<Point2f> points1, points2;
for (size_t i = 0; i < good.size(); i++)
{
points1.push_back(kp1[good[i].queryIdx].pt);
points2.push_back(kp2[good[i].trainIdx].pt);
}
// 计算基础矩阵和本质矩阵
Mat F, mask;
F = findFundamentalMat(points1, points2, FM_RANSAC, 3.0, 0.99, mask);
Mat E = findEssentialMat(points1, points2, 1.0, Point2d(0, 0), RANSAC, 0.999, 1.0, mask);
// 计算相机位姿
Mat R, t;
recoverPose(E, points1, points2, R, t);
// 构建投影矩阵
double fx = 1000.0, fy = 1000.0, cx = img1.cols / 2.0, cy = img1.rows / 2.0;
Mat K = (Mat_<double>(3, 3) << fx, 0, cx, 0, fy, cy, 0, 0, 1);
Mat P1, P2;
hconcat(K, Mat::zeros(3, 1, CV_64F), P1);
hconcat(K * R, K * t, P2);
// 三角测量
Mat points4D;
triangulatePoints(P1, P2, points1, points2, points4D);
// 将齐次坐标转化为三维坐标
std::vector<Point3f> points3D;
for (int i = 0; i < points4D.cols; i++)
{
Point3f p(points4D.at<float>(0, i) / points4D.at<float>(3, i),
points4D.at<float>(1, i) / points4D.at<float>(3, i),
points4D.at<float>(2, i) / points4D.at<float>(3, i));
points3D.push_back(p);
}
// 输出结果
for (size_t i = 0; i < points3D.size(); i++)
{
std::cout << points3D[i].x << " " << points3D[i].y << " " << points3D[i].z << std::endl;
}
return 0;
}
```
需要注意的是,C++代码中的一些函数和数据类型与Python代码有所不同,需要进行相应的更改。例如,C++中需要使用`cv::Mat`代替Python中的`numpy`数组,需要使用`cv::Point2f`和`cv::Point3f`代替Python中的`(x,y)`和`(x,y,z)`坐标。此外,C++中需要手动进行内存分配和释放,需要注意避免内存泄漏等问题。
阅读全文
相关推荐
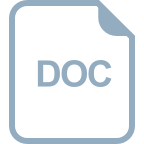
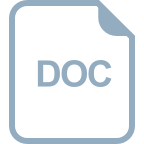
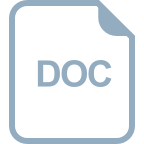
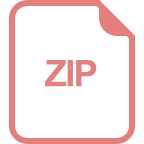
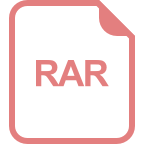
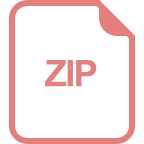
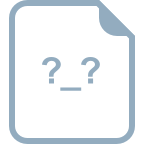
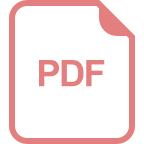
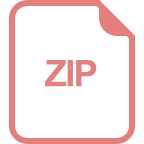
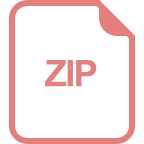
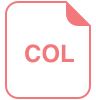
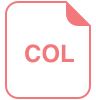




