R语言的支持向量机实现在二分类差异基因中筛选关键基因的代码和具体的数据表格格式
时间: 2024-02-22 22:01:48 浏览: 42
好的,以下是一份示例数据和相应的 R 代码,用于在二分类差异基因中筛选关键基因:
数据表格格式:
| Gene | Sample1_Control | Sample1_Disease | Sample2_Control | Sample2_Disease | Sample3_Control | Sample3_Disease | Class |
|-------|----------------|----------------|----------------|----------------|----------------|----------------|-------|
| Gene1 | 3.56 | 4.18 | 2.78 | 2.91 | 3.15 | 3.97 | 0 |
| Gene2 | 3.21 | 3.87 | 3.01 | 2.81 | 2.95 | 3.54 | 0 |
| Gene3 | 4.03 | 4.19 | 3.83 | 3.98 | 4.09 | 4.16 | 1 |
| Gene4 | 2.83 | 3.09 | 2.92 | 2.77 | 2.99 | 3.01 | 0 |
| Gene5 | 4.18 | 4.05 | 3.78 | 3.93 | 4.08 | 4.01 | 1 |
其中,Gene 列是基因名称,Sample1_Control、Sample2_Control、Sample3_Control 列是对照组样本的基因表达值,Sample1_Disease、Sample2_Disease、Sample3_Disease 列是疾病组样本的基因表达值,Class 列是样本类别(0 或 1)。
以下是相应的 R 代码:
```R
# 导入所需库
library(e1071)
# 导入数据
data <- read.csv("data.csv")
# 划分训练集和测试集
set.seed(123)
trainIndex <- createDataPartition(data$Class, p=0.7, list=FALSE)
trainData <- data[trainIndex,]
testData <- data[-trainIndex,]
# SVM 模型训练
svm_model <- svm(Class ~ ., data = trainData, kernel = "linear", cost = 1)
# 获取关键基因
key_genes <- rownames(trainData)[svm_model$index]
# SVM 模型预测
y_predict <- predict(svm_model, testData[,-ncol(testData)])
# 模型评估
table(testData$Class, y_predict)
```
这段代码使用了 SVM 模型对示例数据进行了二分类,其中使用 `read.csv` 函数导入了数据,使用 `createDataPartition` 函数划分了训练集和测试集。使用 `svm` 函数训练了一个线性 SVM 模型,使用 `svm_model$index` 获取了支持向量的索引,从而获取了关键基因。使用 `predict` 函数预测了测试集的标签,最后使用 `table` 函数对预测结果进行了评估。在实际应用中,你可以根据具体的数据集和问题进行调整和优化。
相关推荐
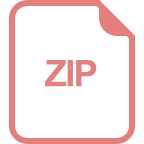
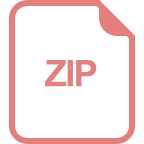
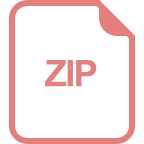
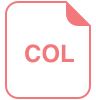
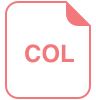
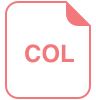











