Failed to convert a NumPy array to a Tensor (Unsupported object type numpy.ndarray).
时间: 2024-02-11 10:05:30 浏览: 30
这个错误通常是因为你试图将一个numpy数组转换为Tensor时出现了问题。在PyTorch中,Tensor是其核心数据结构之一,它与NumPy数组非常相似。
要解决这个问题,你可以尝试使用`torch.from_numpy()`函数将numpy数组转换为Tensor。例如,如果你有一个名为`numpy_array`的numpy数组,你可以使用以下代码将其转换为Tensor:
```
import torch
tensor = torch.from_numpy(numpy_array)
```
如果你仍然遇到问题,请确保你的numpy数组的数据类型与PyTorch支持的数据类型匹配。例如,如果你的numpy数组的数据类型是`float64`,你可以使用以下代码将其转换为`float32`类型:
```
import numpy as np
import torch
numpy_array = np.array([[1,2,3],[4,5,6]], dtype=np.float64)
tensor = torch.from_numpy(numpy_array.astype(np.float32))
```
这将强制将数组转换为`float32`类型,并将其转换为Tensor。
相关问题
Failed to convert a NumPy array to a Tensor (Unsupported object type numpy.ndarray)
这个错误通常是因为你试图将一个numpy数组转换为Tensor时出现了问题。在PyTorch中,Tensor是其核心数据结构之一,它与NumPy数组非常相似。
要解决这个问题,你可以尝试使用`torch.from_numpy()`函数将numpy数组转换为Tensor。例如,如果你有一个名为`numpy_array`的numpy数组,你可以使用以下代码将其转换为Tensor:
```
import torch
tensor = torch.from_numpy(numpy_array)
```
如果你仍然遇到问题,请确保你的numpy数组的数据类型与PyTorch支持的数据类型匹配。例如,如果你的numpy数组的数据类型是`float64`,你可以使用以下代码将其转换为`float32`类型:
```
import numpy as np
import torch
numpy_array = np.array([[1,2,3],[4,5,6]], dtype=np.float64)
tensor = torch.from_numpy(numpy_array.astype(np.float32))
```
这将强制将数组转换为`float32`类型,并将其转换为Tensor。
failed to convert a numpy array to a tensor (unsupported object type numpy.ndarray).
### 回答1:
这个错误是因为你试图将一个NumPy数组转换为Tensor,但是这个数组的对象类型不受支持,导致转换失败。
为了解决这个问题,你需要查看你的NumPy数组的数据类型,并将其转换为Tensor支持的数据类型。你可以使用`torch.tensor()`或`torch.from_numpy()`将NumPy数组转换为Tensor。如果你使用的是PyTorch框架,也可以使用`torch.as_tensor()`将NumPy数组转换为Tensor。
例如,如果你有一个NumPy数组`numpy_array`,你可以使用以下代码将其转换为Tensor:
```
import torch
import numpy as np
numpy_array = np.array([1, 2, 3])
tensor = torch.from_numpy(numpy_array)
```
这将创建一个与NumPy数组相同的Tensor,你可以在后续的代码中使用这个Tensor。
### 回答2:
错误信息显示无法将NumPy数组转换为张量,因为不支持NumPy数组这种类型的对象。这个错误通常发生在使用TensorFlow时,因为TensorFlow使用张量来表示计算图中的数据。张量是多维数组,其中每个元素都具有相同的数据类型。由于NumPy数组在很多方面与张量类似,所以TensorFlow也支持将NumPy数组转换为张量。
但是,当出现“unsupported object type numpy.ndarray”错误时,通常是因为NumPy数组中的数据类型未被支持或是格式不正确。例如,如果数组中包含复数,而TensorFlow只支持实数,转换就会失败。
解决此错误的方法有很多,以下是一些可能的解决方案:
1. 检查NumPy数组中的数据类型。确保所有数据都是支持的类型,比如整数或浮点数,而不是字符串或复数。
2. 确保NumPy数组的形状(shape)与期望的张量形状相同。如果形状不对,可以使用NumPy中的reshape()函数来修改形状。
3. 将NumPy数组转换为TensorFlow张量时,可以显式指定数据类型。例如,可以使用tf.constant()函数而不是tf.convert_to_tensor()函数,并指定数据类型为tf.float32或tf.int32等。
4. 如果数据类型无法转换,则可以尝试转换为支持的类型,如int、float或bool等。
总之,解决此错误的关键是确保NumPy数组在类型和形状上与TensorFlow所需的张量相同。可以使用NumPy或TensorFlow的函数来修改数组的形状或数据类型,以确保成功地将NumPy数组转换为TensorFlow张量。
### 回答3:
在使用TensorFlow进行深度学习模型训练时,经常需要将数据从numpy数组转换成Tensor对象。但在某些情况下,转换过程中可能会遇到“failed to convert a numpy array to a tensor (unsupported object type numpy.ndarray)”这个错误提示,这是由于TensorFlow不支持numpy数组类型而导致的。
为了解决这个问题,我们可以使用TensorFlow的API函数tf.convert_to_tensor()将numpy数组转换成Tensor对象。该函数会将numpy数组包装成Tensor对象,让其可被TensorFlow框架识别和使用。
下面是一个示例代码:
```python
import tensorflow as tf
import numpy as np
data = np.array([[1, 2], [3, 4]])
tensor = tf.convert_to_tensor(data)
print(tensor)
```
这里我们定义了一个numpy二维数组data,然后使用tf.convert_to_tensor()函数将其转换成了Tensor对象tensor。最后我们输出tensor的值,可以看到它包含了原始numpy数组中的所有元素。
除了使用tf.convert_to_tensor()函数外,还有其他方法可以实现numpy数组到Tensor对象的转换。例如,我们可以使用TensorFlow的from_tensorflow方法或在创建Tensor时直接指定数据类型等。但无论哪种方法,都需要保证转换后的Tensor对象能被TensorFlow正常使用。
总结一下,当我们遇到“failed to convert a numpy array to a tensor (unsupported object type numpy.ndarray)”错误时,需要使用TensorFlow的tf.convert_to_tensor()函数将numpy数组转换成Tensor对象,以便TensorFlow可以正常使用。
相关推荐
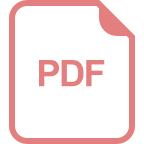
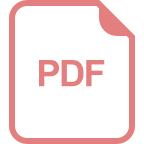
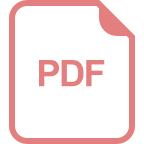












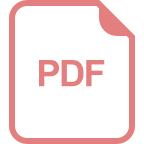