transform=transforms.Compose([transforms.Resize((224,224)),transforms.ToTensor()])
时间: 2024-05-25 21:16:05 浏览: 82
This code creates a transformation pipeline using the `Compose` class from the `transforms` module in PyTorch. The pipeline consists of two transformations applied in sequence:
1. `Resize((224,224))`: This resizes the input image to have a height and width of 224 pixels. This is a common size used in many computer vision models.
2. `ToTensor()`: This converts the resized image to a PyTorch tensor. PyTorch models typically expect inputs to be in tensor format.
Overall, this transformation pipeline can be used to preprocess input images before passing them to a PyTorch model for inference or training.
相关问题
self.transform = transforms.Compose
self.transform = transforms.Compose是PyTorch中用于定义数据预处理操作的方法。它允许将多个预处理步骤组合在一起,以便在数据集上进行一系列的转换操作[^1]。
下面是一个示例,展示了如何使用transforms.Compose来定义一个数据预处理操作的序列:
```python
import torchvision.transforms as transforms
# 定义预处理操作
transform = transforms.Compose([
transforms.Resize(256), # 调整图像大小为256x256
transforms.CenterCrop(224), # 中心裁剪为224x224
transforms.ToTensor(), # 转换为张量
transforms.Normalize(mean=[0.485, 0.456, 0.406], std=[0.229, 0.224, 0.225]) # 标准化
])
# 在数据集上应用预处理操作
dataset = YourDataset(transform=transform)
```
在上面的示例中,我们首先导入了torchvision.transforms模块,并使用transforms.Compose方法定义了一个名为transform的预处理操作序列。这个序列包含了四个操作:Resize、CenterCrop、ToTensor和Normalize。这些操作将按照定义的顺序依次应用于数据集中的每个样本。
通过使用transforms.Compose,我们可以方便地将多个预处理操作组合在一起,以便在数据集上进行一系列的转换操作。这样可以简化代码,并且使得数据预处理过程更加灵活和可定制。
self.transform = transforms.Compose(transforms_)
在PyTorch的数据处理流程中,`self.transform = transforms.Compose(transforms_)` 这一行代码是用来设置模型训练或预处理数据时的一系列变换操作。`transforms.Compose` 是 torchvision 库中的一个功能,它接受一个由多个 `torchvision.transforms` 对象组成的列表(transforms_),然后按照列表中的顺序对输入数据进行依次变换。
例如,`transforms.Compose([transforms.Resize(size), transforms.CenterCrop(size), transforms.ToTensor()])` 表示首先将图片缩放到指定大小,接着在图片中心裁剪出同样大小的部分,最后将裁剪后的图像转换成 PyTorch 可用的 tensor 格式。这样做的目的是标准化数据,使其适应模型的输入要求,如卷积神经网络(CNN)通常需要预处理成固定大小的图片。
每个变换对象(如 Resize、CenterCrop 等)都接收特定的参数,如大小、位置等。当应用于实际数据集时,所有这些变换会按顺序串联执行。
相关推荐
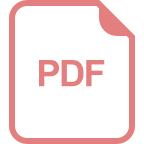
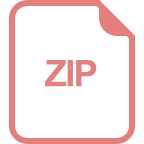
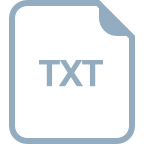












