import cv2 import torch # 加载模型 model = torch.hub.load('./base_s16', 'custom' , path='base_s16/weights/best.pt', source='local') # 1.获取视频对象 cap = cv2.VideoCapture('gao.mp4') # 2.判断是否读取成功 while(cap.isOpened()): # 3.获取每一帧图像 ret, frame = cap.read() # 镜像 # frame = cv2.flip(frame, 1) # print(model) # bgr --> rgb img_cvt = cv2.cvtColor(frame, cv2.COLOR_BGR2RGB) results = model(img_cvt) # 推理结果 pd = results.pandas().xyxy[0] person_list = pd[pd['name'] == 'person'].to_numpy() print(person_list) helmet_list = pd[pd['name'].str.contains('helmet')].to_numpy() print(helmet_list) # 4.获取成功显示图像 if ret == True: cv2.imshow('frame',frame) # 5.每一帧间隔为25ms break # if cv2.waitKey(25) & 0xFF == ord('q'): # break # 6.释放视频对象 cap.release() cv2.destoryAllwindows()修改一下
时间: 2024-04-05 17:29:16 浏览: 82
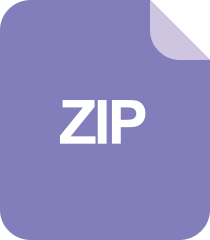
基于Torch Hub的深度估计模型MiDaS-python源码.zip
这段代码实现的功能是:加载一个已经训练好的模型,使用 OpenCV 库获取一个视频对象,读取视频中的每一帧图像并进行镜像处理,将每一帧图像输入到模型中进行推理,得到人和安全帽的位置坐标,最后在每一帧图像上标记出人和安全帽的位置,并显示出来。其中,torch.hub.load()函数的作用是从指定的目录或 URL 加载一个已经训练好的 PyTorch 模型,并返回该模型。
阅读全文
相关推荐
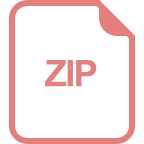
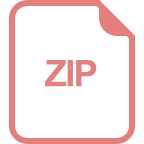
















