python编写代码实现任意一种树模型算法(id3, c4.5, least squares回归树, etc),包括回归和分类【可直接调用sklearn库实现】 ,与sklearn中的算法对比
时间: 2024-11-16 19:20:29 浏览: 0
Python是一种流行的编程语言,其scikit-learn(sklearn)库提供了丰富的机器学习算法,包括决策树模型如ID3、C4.5(通过`DecisionTreeClassifier`和`DecisionTreeRegressor`实现),以及线性回归树(Least Squares Regression Tree,也称为LSR树,虽然sklearn没有直接提供这个名称,但可以使用`ensemble.ExtraTreesRegressor`作为近似实现,因为它是基于随机森林的思想,其中包含多个回归树)。
下面是一个简单的例子,展示如何使用sklearn库实现ID3决策树分类:
```python
from sklearn.tree import DecisionTreeClassifier
from sklearn.datasets import load_iris
# 加载鸢尾花数据集
iris = load_iris()
X = iris.data
y = iris.target
# 创建ID3分类器
clf = DecisionTreeClassifier(criterion='gini', max_depth=3) # 使用信息增益(Gini impurity)度量和最大深度为3
# 训练模型
clf.fit(X, y)
# 预测新样本
new_samples = [[5.1, 3.5, 1.4, 0.2]] # 示例特征向量
predictions = clf.predict(new_samples)
print("预测结果:", predictions[0])
```
对于回归问题,你可以使用类似的方式,用`DecisionTreeRegressor`替换`DecisionTreeClassifier`:
```python
from sklearn.tree import DecisionTreeRegressor
# 示例:房价预测
house_data = load_boston() # 加载波士顿房价数据
X = house_data.data
y = house_data.target
regr = DecisionTreeRegressor(max_depth=3)
regr.fit(X, y)
new_house_features = [[0.0379624, 0.06917724, 0.027847, ..., 17.8]] # 示例房屋特征
predicted_price = regr.predict(new_house_features)
print("预测房价:", predicted_price[0])
```
sklearn的算法通常经过优化,并且有详细的文档支持。与自定义实现相比,它们更稳定、高效,且易于使用。不过,如果你需要深入了解算法的工作原理或对性能有特殊需求,自定义实现可能会更有优势。同时,sklearn还提供了许多选项供你调整模型的复杂度和性能。
阅读全文
相关推荐
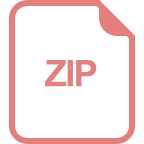
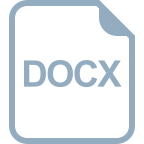
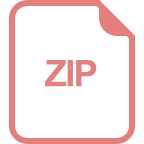















