def xgboost_forecast(train, testX): # transform list into array train = asarray(train) # split into input and output columns trainX, trainy = train[:, :-1], train[:, -1] # fit model model = XGBRegressor(objective='reg:squarederror', n_estimators=1000) model.fit(trainX, trainy) # make a one-step prediction yhat = model.predict(asarray([testX])) return yhat[0]什么意思哦
时间: 2024-04-25 07:27:52 浏览: 11
这是一个使用 XGBoost 模型进行时间序列预测的 Python 函数。具体来说,它接受一个训练集 train 和一个测试数据 testX。训练集应该是一个二维数组,其中每一行代表一个样本,每一列代表一个特征,最后一列代表该样本的输出。函数首先将训练集转换为 Numpy 数组,并将其拆分为输入特征矩阵 trainX 和输出向量 trainy。然后,它使用 XGBRegressor 类初始化一个 XGBoost 回归模型,并使用训练集来拟合该模型。最后,函数将测试数据转换为 Numpy 数组,并使用模型来进行一步预测。预测结果以一个浮点数的形式返回。
相关问题
# walk-forwardvalidation for univariate data defwalk_forward_validation(data, n_test): predictions = list() # split dataset train, test = train_test_split(data,n_test) # seed history with training dataset history = [x for x in train] # step over each time-step in the testset for i in range(len(test)): # split test row into input andoutput columns testX, testy = test[i, :-1],test[i, -1] # fit model on history and make aprediction yhat = xgboost_forecast(history,testX) # store forecast in list ofpredictions predictions.append(yhat) # add actual observation tohistory for the next loop history.append(test[i]) # summarize progress print('>expected=%.1f,predicted=%.1f' % (testy, yhat)) # estimate prediction error error = mean_absolute_error(test[:, 1],predictions) return error, test[:, 1], predictions
这是一个用于单变量数据的走步验证(walk-forward validation)函数,其中包括以下步骤:
1. 将数据集分为训练集和测试集
2. 在训练集上训练模型,并在测试集上进行预测
3. 将预测结果存储在一个列表中
4. 为下一次循环将实际观测值添加到历史记录中
5. 计算预测误差并返回误差、测试集真实值和预测值
在该函数中,使用了 XGBoost 模型进行预测。
下面的函数中的参数各自代表什么意思def create_tf_dataset( data_array: np.ndarray, input_sequence_length: int, forecast_horizon: int, batch_size: int = 128, shuffle=True, multi_horizon=True, ):
这个函数是用来创建 TensorFlow 的数据集的,其中参数的含义如下:
- data_array: np.ndarray,代表输入的数据数组。
- input_sequence_length: int,代表输入序列的长度。
- forecast_horizon: int,代表预测的时间步数。
- batch_size: int,代表每个批次的大小。
- shuffle: bool,代表是否打乱数据集。
- multi_horizon: bool,代表是否使用多个预测时间步数。
相关推荐
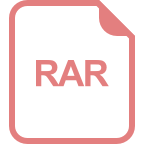
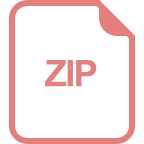
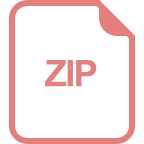









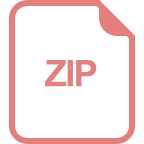
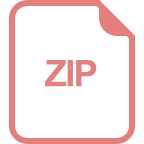
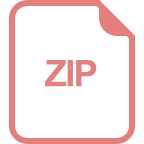